RatioFi: Unlocking the Potential of WiFi CSI
2023 International Conference on Ubiquitous Communication (Ucom)(2023)
摘要
Wi-Fi sensing empowered by channel state information (CSI) has shown superiority among various sensors (e.g., camera, radar, infra-red, etc.) for its cost-effective, privacy-preserving and contactless merits. However, commercial off-the-shelf Wi-Fi devices use communication waveforms that are not emphasized on sensing, resulting in its disadvantages of environment dependence and noisiness of the recorded data, comparing to sensing dedicated devices like radars. This, in turn, leads to the requirement of a dedicated CSI recognition model trained on a big labeled dataset that covers every deploying environment, which is impractical for massive deployment. To overcome this problem, we propose a three-stage deep learning-based workflow RatioFi, integrating semi-supervised learning and few-shot learning. In the first stage, taking amplitude and phase as two different view that share common effective feature for downstream tasks of CSI samples, the model employs a two-flow structure for the two views to learn about fine-grained information about CSI. Next, the model is trained on a small task-specific labeled dataset, which is easier to obtain, and can adapt to different classification task, overcoming the aforementioned problem of environment dependence and noisiness of the sensed data with less effort on data collection, further facilitating the large-scale deployment of Wi-Fi sensing. Then the model make inference for every newly collected sample. We implement and show that the workflow can be adapted to different classifying tasks such as human identification and activity recognition. We demonstrate the performance supremacy of the proposed design to the existing benchmark algorithms designed for the same scenario.
更多查看译文
关键词
Wi-Fi sensing,few-shot learning(FSL),human identification,human activity recognition,deep learning
AI 理解论文
溯源树
样例
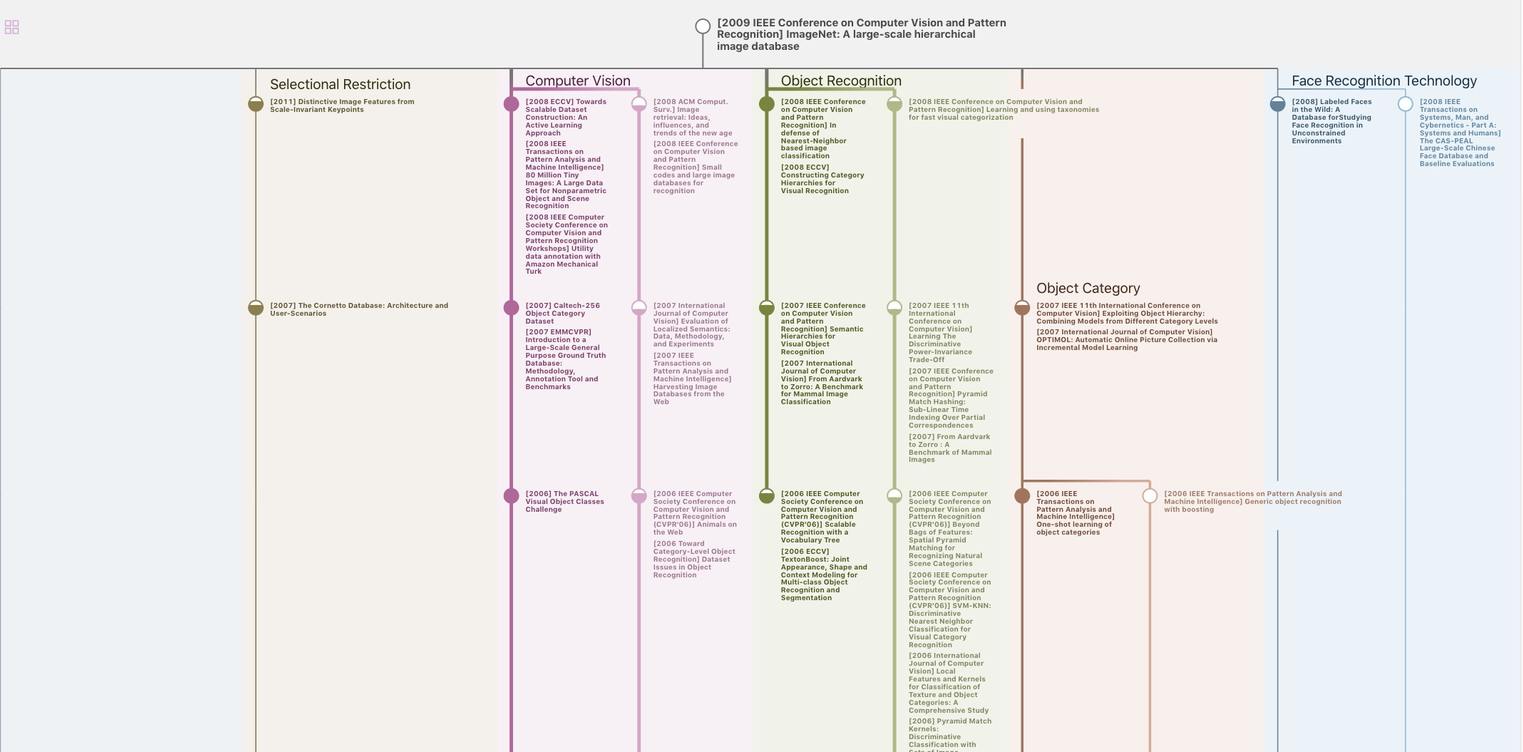
生成溯源树,研究论文发展脉络
Chat Paper
正在生成论文摘要