Voice-Face Cross-Modal Association Learning Based on Deep Residual Shrinkage Network
2023 IEEE International Conference on Image Processing and Computer Applications (ICIPCA)(2023)
摘要
Establishing associations between voices and faces has grown in popularity in recent years, but current voice-face cross-modal association methods face challenges such as limited feature extraction capability and insufficient semantic associations. To address the aforementioned issues, we propose a voice-face cross-modal association learning method based on a deep residual shrinkage network. First, a deep residual shrinkage block is added to the dual-stream residual network to improve network training efficiency and acquire more discriminative embedded features. Then the multi-similarity loss function is used in metric learning to tap into the connections between voice and face modalities and enhance the network's robustness and generalization ability. In voice-face cross-modal verification, cross-modal matching, and cross-modal retrieval tasks, experimental findings indicate that our method improves accuracy by about 2% over existing baseline methods.
更多查看译文
关键词
cross-modal association learning,deep residual shrinkage network,multi-similarity loss,cross-modal matching
AI 理解论文
溯源树
样例
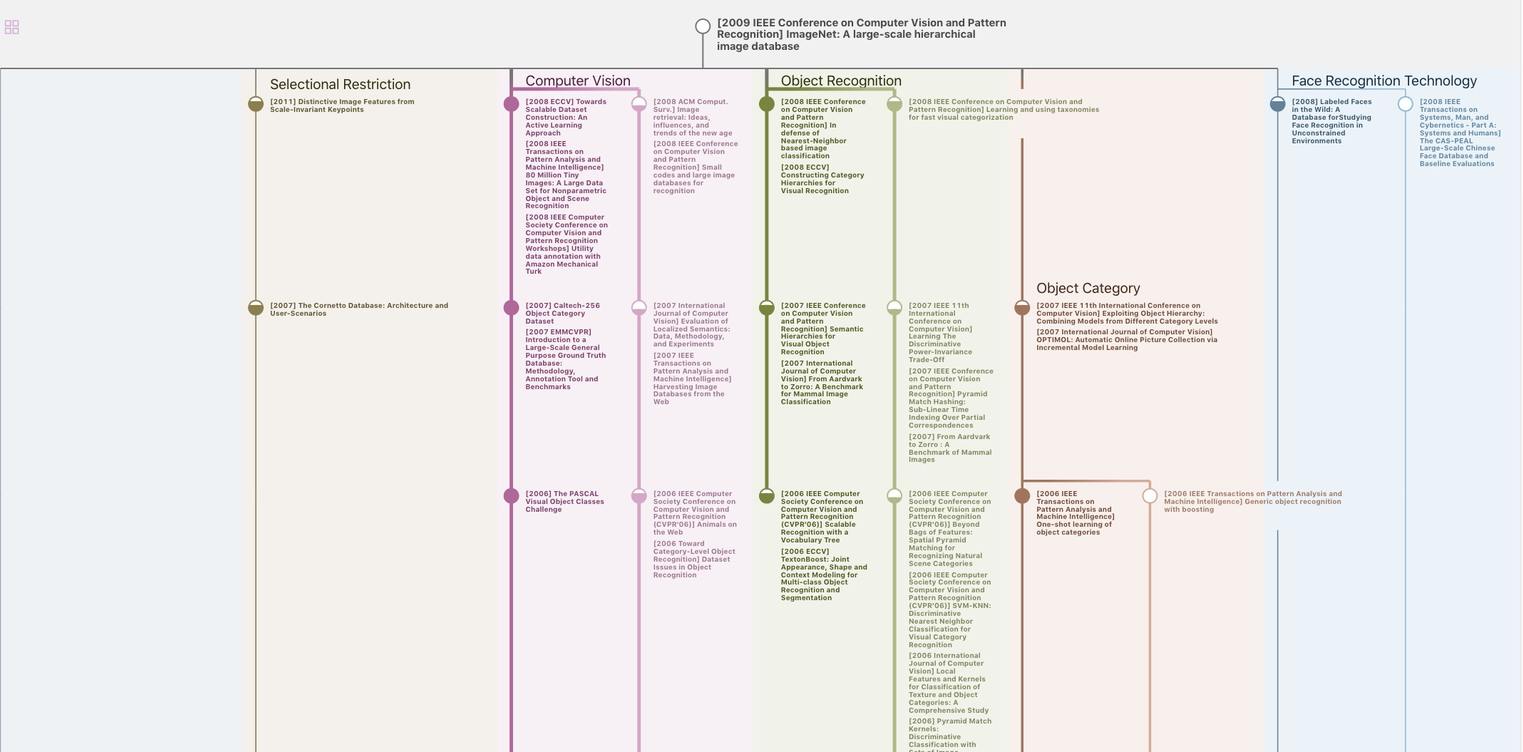
生成溯源树,研究论文发展脉络
Chat Paper
正在生成论文摘要