Market index price prediction using Deep Neural Networks with a Self-Similarity approach
Applied Soft Computing(2023)
摘要
Stock indexes are of vital importance to understand financial and economic markets of sectors and nations. Given the importance of market indexes, researchers, investors, and policy makers are continuously working to improve models to forecast market movements; small improvements in modeling have the potential for large financial gains. To this end, machine learning approaches have gained in popularity as both software and hardware performance have increased. In this work, we apply machine learning applications to the S&P 500, DAX, AEX and the SMI indexes to improve forecasting performance. In particular, we take advantage of the fractal and Self-Similarity behaviors that exist in the time series of these indexes using simple Recurrent Neural Network, Multilayer Perceptron, and Long-Short Term Memory architectures. We apply the architecture using 60-, 30-, and 15-min windows of daily series for each approach and index. The results indicate that for the S&P 500 the proposed self-similarity models outperform all base approaches. However, results are not uniform across all models and indexes. Overall, performance differences across models and indexes are presented. To check the difference between the forecasts of the self-similarity and base models, Model Confidence Set was used.
更多查看译文
关键词
Deep learning,Intraday data,Index stock market,Price stock prediction,Self-similarity,Fractal geometry finance
AI 理解论文
溯源树
样例
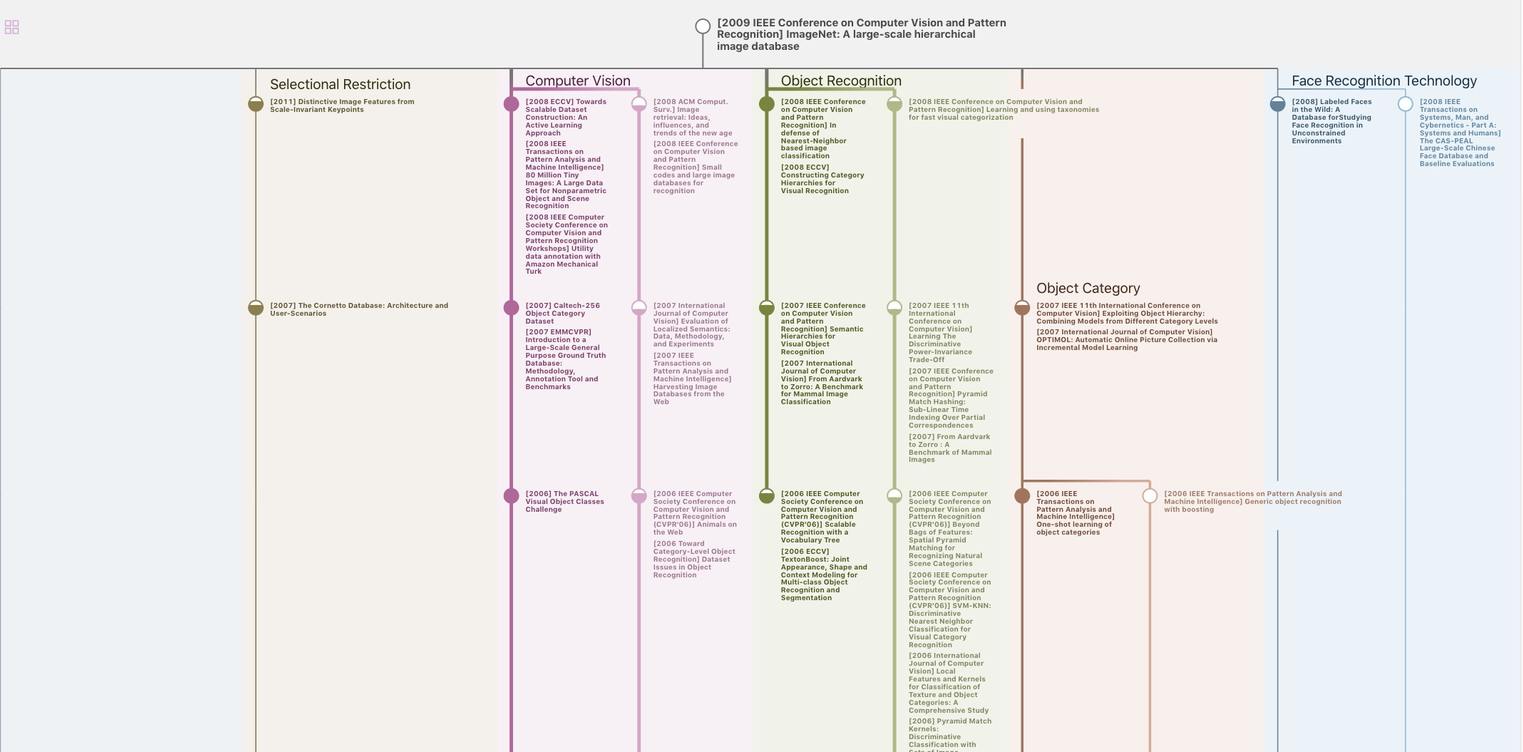
生成溯源树,研究论文发展脉络
Chat Paper
正在生成论文摘要