Defining Volume Targets for Regionalization of Pancreaticoduodenectomy, Esophagectomy, and Major Lung Resection Centers to Improve Oncologic and Surgical Outcomes.
Annals of surgical oncology(2023)
摘要
BACKGROUND:Performance of complex cancer surgeries at high-volume (HV) centers has been shown to reduce operative mortality. However, the case volume threshold that should be used to define HV centers is unknown. In this study, we determined thresholds to define HV pancreaticoduodenectomy, esophagectomy, and major lung resection centers based on clinical parameters. Then, we assessed the association of hospital volume with oncologic outcomes and overall survival.
METHODS:We identified adult NCDB patients undergoing pancreaticoduodenectomy, esophagectomy, and major lung resections between 2004 and 2015. Multivariable models with restricted cubic splines were built to predict 5-year overall survival for each surgery group according to average yearly case volume, adjusting for demographic and clinicopathologic factors. The change point procedure was then used to identify volume cut-points for each surgery type.
RESULTS:We identified the following thresholds to define HV status: 25 cases/year for pancreaticoduodenectomy; 18 cases/year for esophagectomy; and 54 cases/year for major lung resections. For all surgery types, treatment at a HV center was associated with an increased likelihood of R0 resection and adequate lymph node evaluation. HV centers had significantly decreased 30- and 90-day, postoperative mortality after adjusting for age, sex, race, comorbidities, histology, and stage. An overall survival benefit also was observed for patients undergoing resections at HV centers.
CONCLUSIONS:Using novel methodology, our study identified volume thresholds for HV pancreaticoduodenectomy, esophagectomy, and major lung resection centers that were associated with improved oncologic outcomes and overall survival. These definitions of HV centers should be considered when evaluating regionalization of complex cancer care.
更多查看译文
AI 理解论文
溯源树
样例
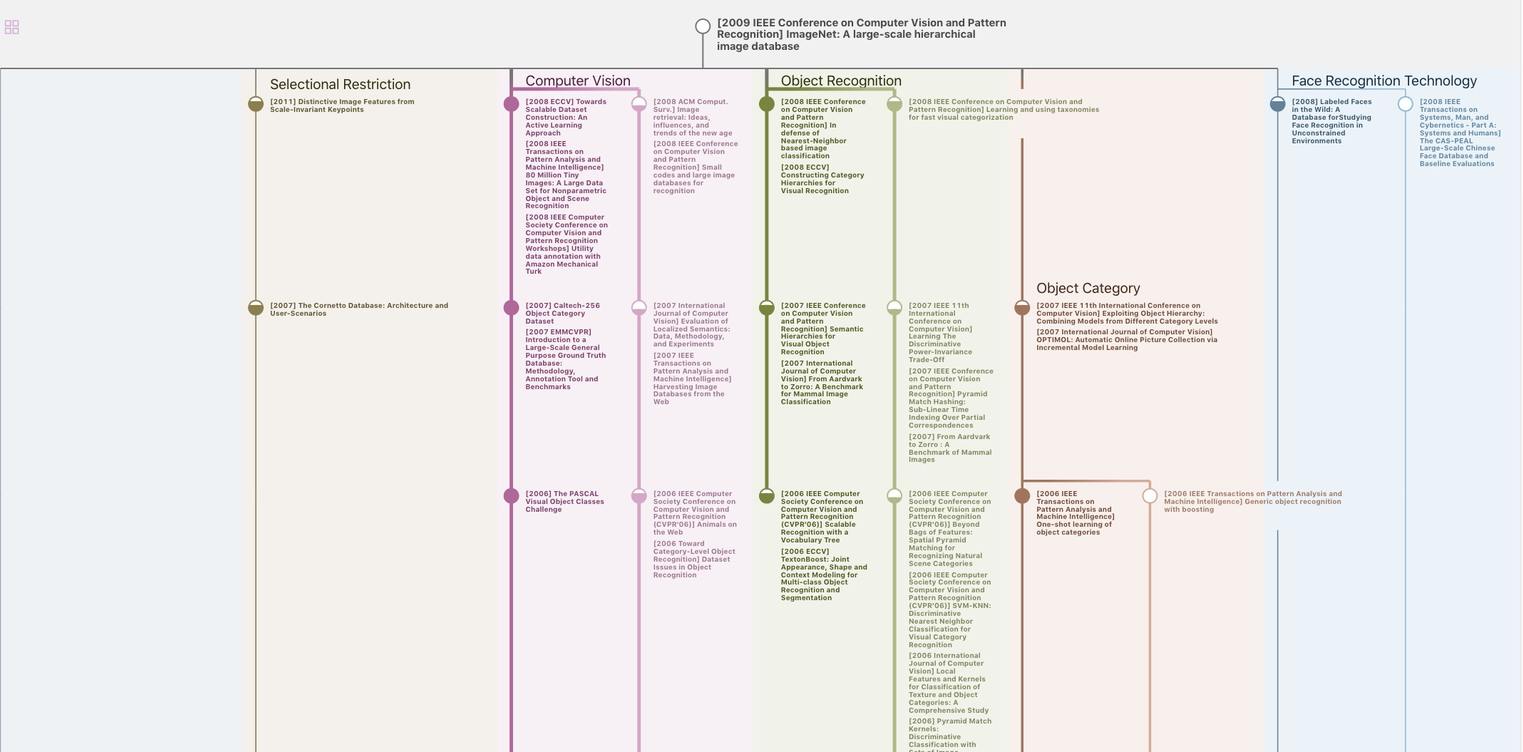
生成溯源树,研究论文发展脉络
Chat Paper
正在生成论文摘要