Electroencephalogram-Based Subject Matching Learning (ESML): A Deep Learning Framework on Electroencephalogram-Based Biometrics and Task Identification.
Behavioral sciences (Basel, Switzerland)(2023)
摘要
An EEG signal (Electroencephalogram) is a bioelectric phenomenon reflecting human brain activities. In this paper, we propose a novel deep learning framework ESML (EEG-based Subject Matching Learning) using raw EEG signals to learn latent representations for EEG-based user identification and tack classification. ESML consists of two parts: one is the ESML1 model via an LSTM-based method for EEG-user linking, and one is the ESML2 model via a CNN-based method for EEG-task linking. The new model ESML is simple, but effective and efficient. It does not require any restrictions for EEG data collection on motions and thinking for users, and it does not need any EEG preprocessing operations, such as EEG denoising and feature extraction. The experiments were conducted on three public datasets and the results show that ESML performs the best and achieves significant performance improvement when compared to baseline methods (i.e., SVM, LDA, NN, DTS, Bayesian, AdaBoost and MLP). The ESML1 model provided the best precision at 96% with 109 users and the ESML2 model achieved 99% precision at 3-Class task classification. These experimental results provide direct evidence that EEG signals can be used for user identification and task classification.
更多查看译文
关键词
subject matching learning,biometrics,task identification,electroencephalogram-based
AI 理解论文
溯源树
样例
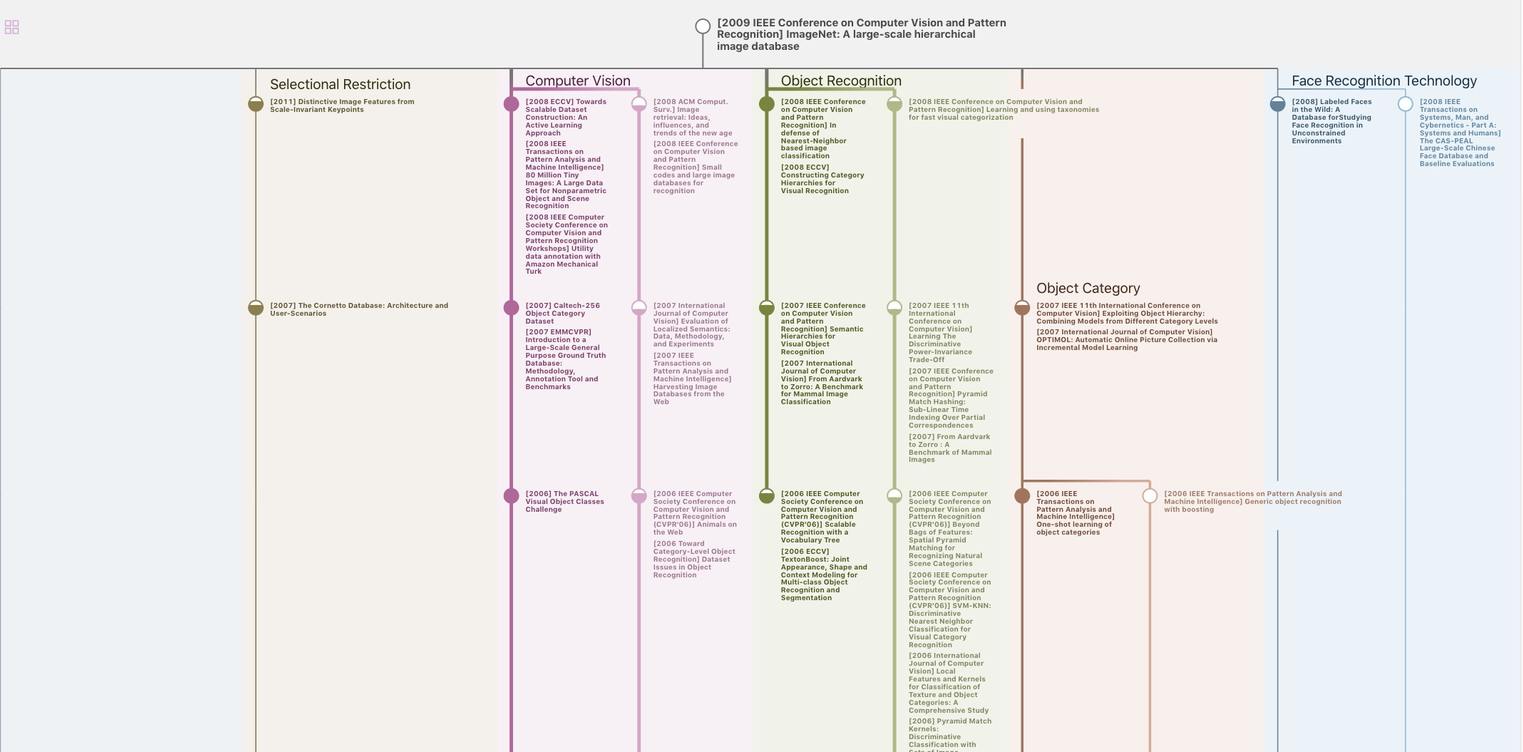
生成溯源树,研究论文发展脉络
Chat Paper
正在生成论文摘要