DTC: Deep Tracking Control
SCIENCE ROBOTICS(2024)
摘要
Legged locomotion is a complex control problem that requires both accuracy and robustness to cope with real-world challenges. Legged systems have traditionally been controlled using trajectory optimization with inverse dynamics. Such hierarchical model-based methods are appealing because of intuitive cost function tuning, accurate planning, generalization, and, most importantly, the insightful understanding gained from more than one decade of extensive research. However, model mismatch and violation of assumptions are common sources of faulty operation. Simulation-based reinforcement learning, on the other hand, results in locomotion policies with unprecedented robustness and recovery skills. Yet, all learning algorithms struggle with sparse rewards emerging from environments where valid footholds are rare, such as gaps or stepping stones. In this work, we propose a hybrid control architecture that combines the advantages of both worlds to simultaneously achieve greater robustness, foot-placement accuracy, and terrain generalization. Our approach uses a model-based planner to roll out a reference motion during training. A deep neural network policy is trained in simulation, aiming to track the optimized footholds. We evaluated the accuracy of our locomotion pipeline on sparse terrains, where pure data-driven methods are prone to fail. Furthermore, we demonstrate superior robustness in the presence of slippery or deformable ground when compared with model-based counterparts. Last, we show that our proposed tracking controller generalizes across different trajectory optimization methods not seen during training. In conclusion, our work unites the predictive capabilities and optimality guarantees of online planning with the inherent robustness attributed to offline learning.
更多查看译文
AI 理解论文
溯源树
样例
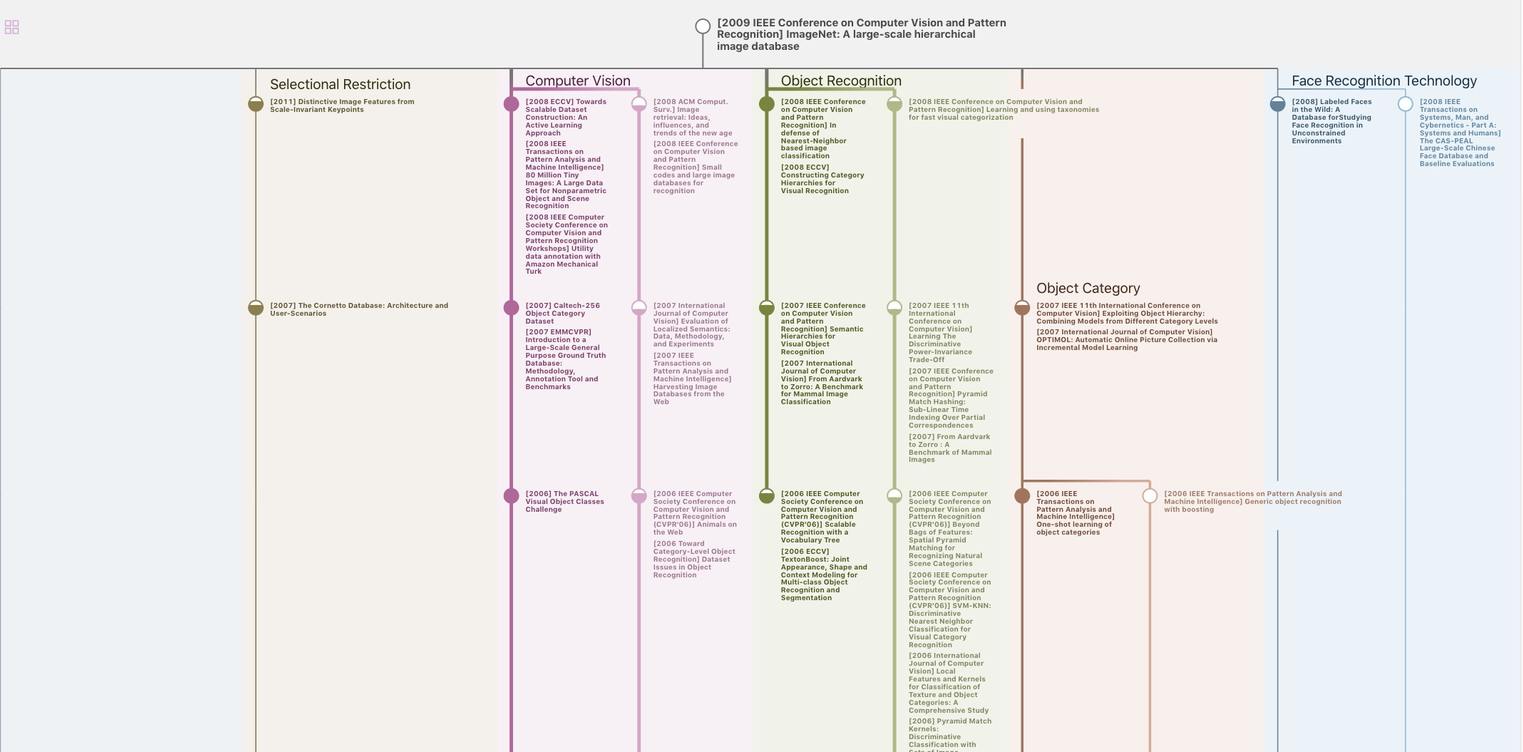
生成溯源树,研究论文发展脉络
Chat Paper
正在生成论文摘要