An Exploration of Optimal Parameters for Efficient Blind Source Separation of EEG Recordings Using AMICA
2023 IEEE 23rd International Conference on Bioinformatics and Bioengineering (BIBE)(2023)
摘要
EEG continues to find a multitude of uses in both neuroscience research and medical practice, and independent component analysis (ICA) continues to be an important tool for analyzing EEG. A multitude of ICA algorithms for EEG decomposition exist, and in the past, their relative effectiveness has been studied. AMICA is considered the benchmark against which to compare the performance of other ICA algorithms for EEG decomposition. AMICA exposes many parameters to the user to allow for precise control of the decomposition. However, several of the parameters currently tend to be set according to "rules of thumb" shared in the EEG community. Here, AMICA decompositions are run on data from a collection of subjects while varying certain key parameters. The running time and quality of decompositions are analyzed based on two metrics: Pairwise Mutual Information (PMI) and Mutual Information Reduction (MIR). Recommendations for selecting starting values for parameters are presented.
更多查看译文
关键词
EEG,ICA,BSS,Mutual Information
AI 理解论文
溯源树
样例
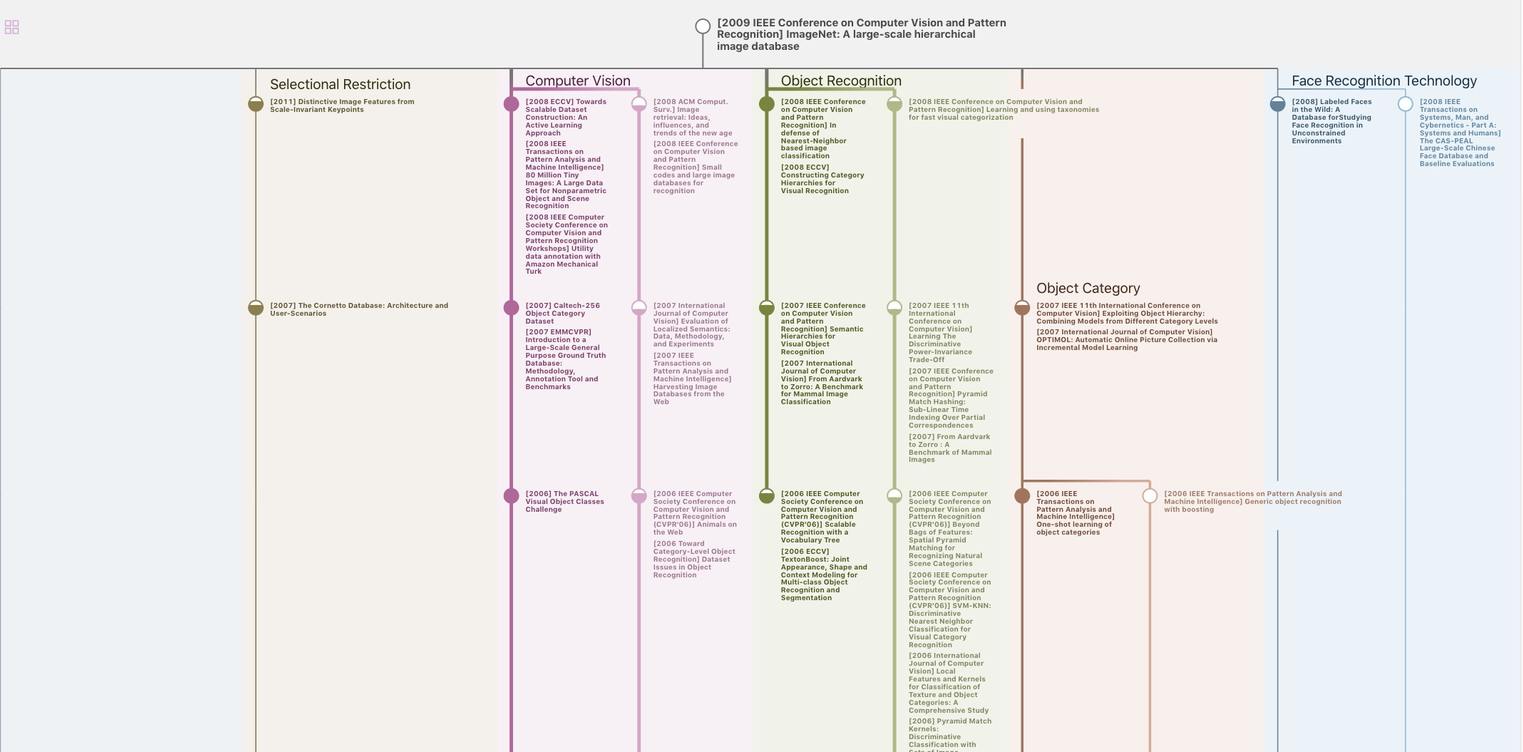
生成溯源树,研究论文发展脉络
Chat Paper
正在生成论文摘要