Managing Energy Consumption of Devices with Multiconnectivity by Deep Learning and Software-Defined Networking
Sensors(2023)
摘要
Multiconnectivity allows user equipment/devices to connect to multiple radio access technologies simultaneously, including 5G, 4G (LTE), and WiFi. It is a necessity in meeting the increasing demand for mobile network services for the 5G and beyond wireless networks, while ensuring that mobile operators can still reap the benefits of their present investments. Multipath TCP (MPTCP) has been introduced to allow uninterrupted reliable data transmission over multiconnectivity links. However, energy consumption is a significant issue for multihomed wireless devices since most of them are battery-powered. This paper employs software-defined networking (SDN) and deep neural networks (DNNs) to manage the energy consumption of devices with multiconnectivity running MPTCP. The proposed method involves two lightweight algorithms implemented on an SDN controller, using a real hardware testbed of dual-homed wireless nodes connected to WiFi and cellular networks. The first algorithm determines whether a node should connect to a specific network or both networks. The second algorithm improves the selection made by the first by using a DNN trained on different scenarios, such as various network sizes and MPTCP congestion control algorithms. The results of our extensive experimentation show that this approach effectively reduces energy consumption while providing better network throughput performance compared to using single-path TCP or MPTCP Cubic or BALIA for all nodes.
更多查看译文
关键词
multipath TCP,software-defined networking,energy consumption,neural networks,congestion control,wireless,multihoming,multiconnectivity
AI 理解论文
溯源树
样例
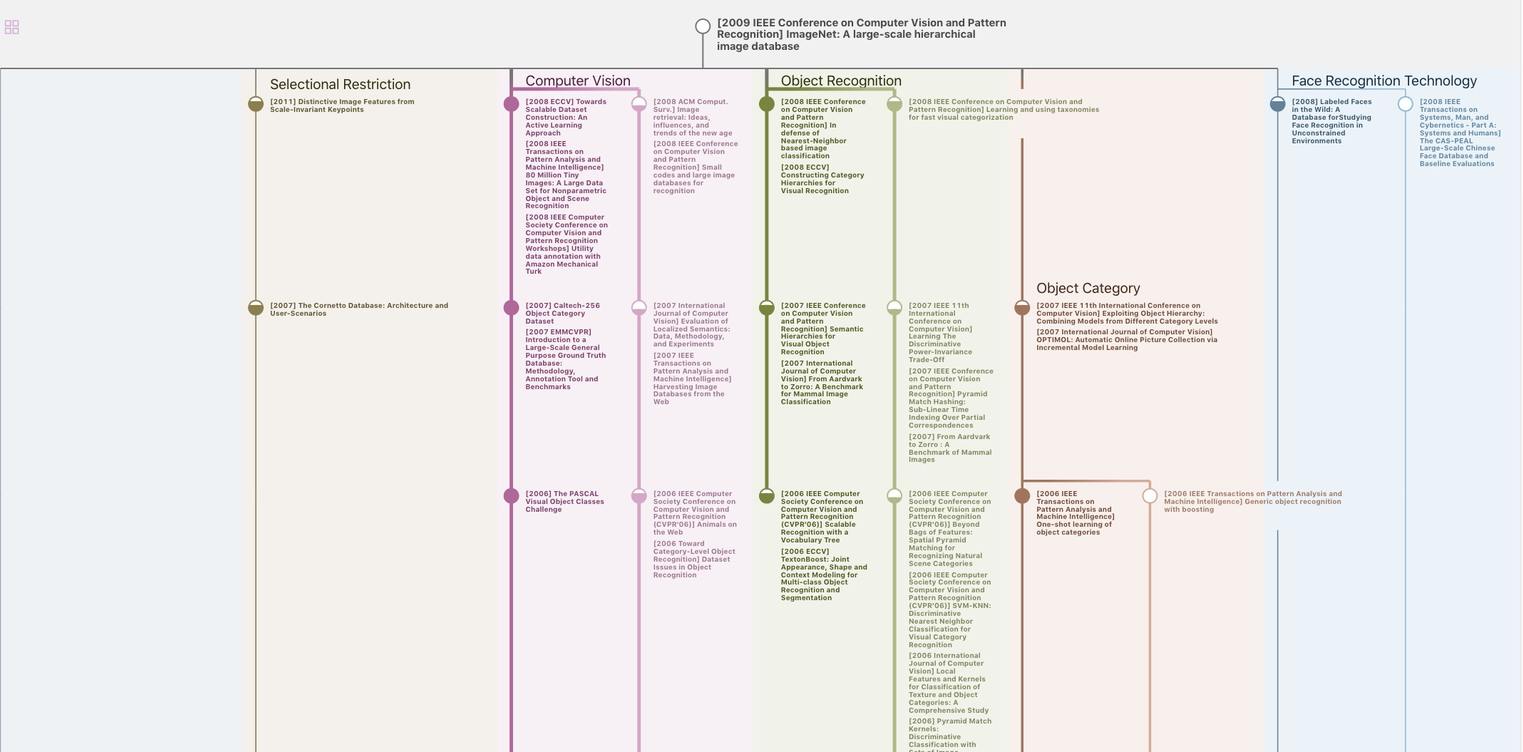
生成溯源树,研究论文发展脉络
Chat Paper
正在生成论文摘要