Investigating Self-Rationalizing Models for Commonsense Reasoning
Stats(2023)
摘要
The rise of explainable natural language processing spurred a bulk of work on datasets augmented with human explanations, as well as technical approaches to leverage them. Notably, generative large language models offer new possibilities, as they can output a prediction as well as an explanation in natural language. This work investigates the capabilities of fine-tuned text-to-text transfer Transformer (T5) models for commonsense reasoning and explanation generation. Our experiments suggest that while self-rationalizing models achieve interesting results, a significant gap remains: classifiers consistently outperformed self-rationalizing models, and a substantial fraction of model-generated explanations are not valid. Furthermore, training with expressive free-text explanations substantially altered the inner representation of the model, suggesting that they supplied additional information and may bridge the knowledge gap. Our code is publicly available, and the experiments were run on open-access datasets, hence allowing full reproducibility.
更多查看译文
关键词
explainability, generative models, large language models, natural language processing, rationales, X-AI
AI 理解论文
溯源树
样例
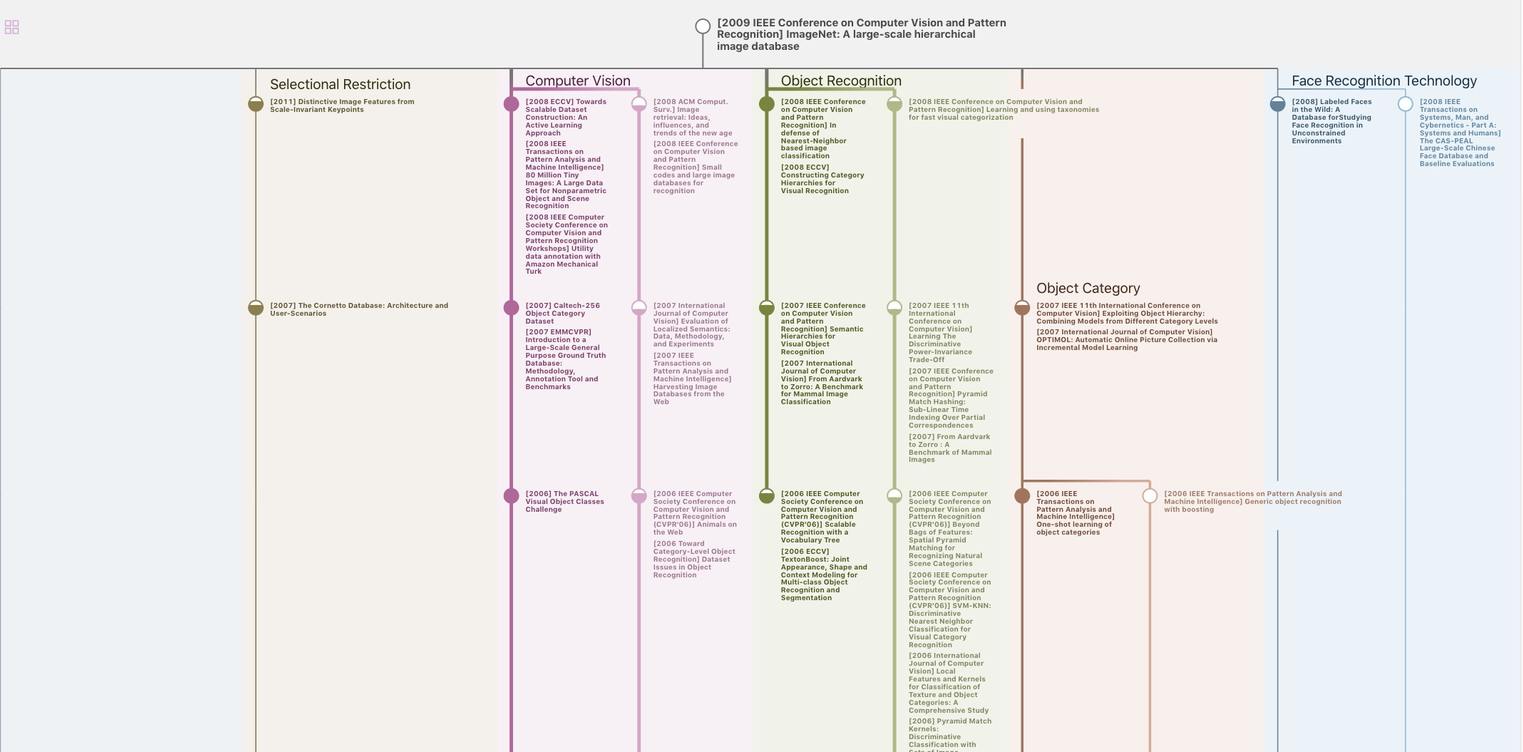
生成溯源树,研究论文发展脉络
Chat Paper
正在生成论文摘要