Interpretable Machine Learning Reveals Potential to Overcome Reactive Flood Adaptation in the Continental US
Earth's Future(2023)
摘要
Abstract Floods cause average annual losses of more than US$30 billion in the US and are estimated to significantly increase due to global change. Flood resilience, which currently differs strongly between socio‐economic groups, needs to be substantially improved by proactive adaptive measures, such as timely purchase of flood insurance. Yet, knowledge about the state and uptake of private adaptation and its drivers is so far scarce and fragmented. Based on interpretable machine learning and large insurance and socio‐economic open data sets covering the whole continental US we reveal that flood insurance purchase is characterized by reactive behavior after severe flood events. However, we observe that the Community Rating System helps overcome this behavior by effectively fostering proactive insurance purchase, irrespective of socio‐economic backgrounds in the communities. Thus, we recommend developing additional targeted measures to help overcome existing inequalities, for example, by providing special incentives to the most vulnerable and exposed communities.
更多查看译文
关键词
FEMA, machine learning, flood insurance, human behavior, flood resilience
AI 理解论文
溯源树
样例
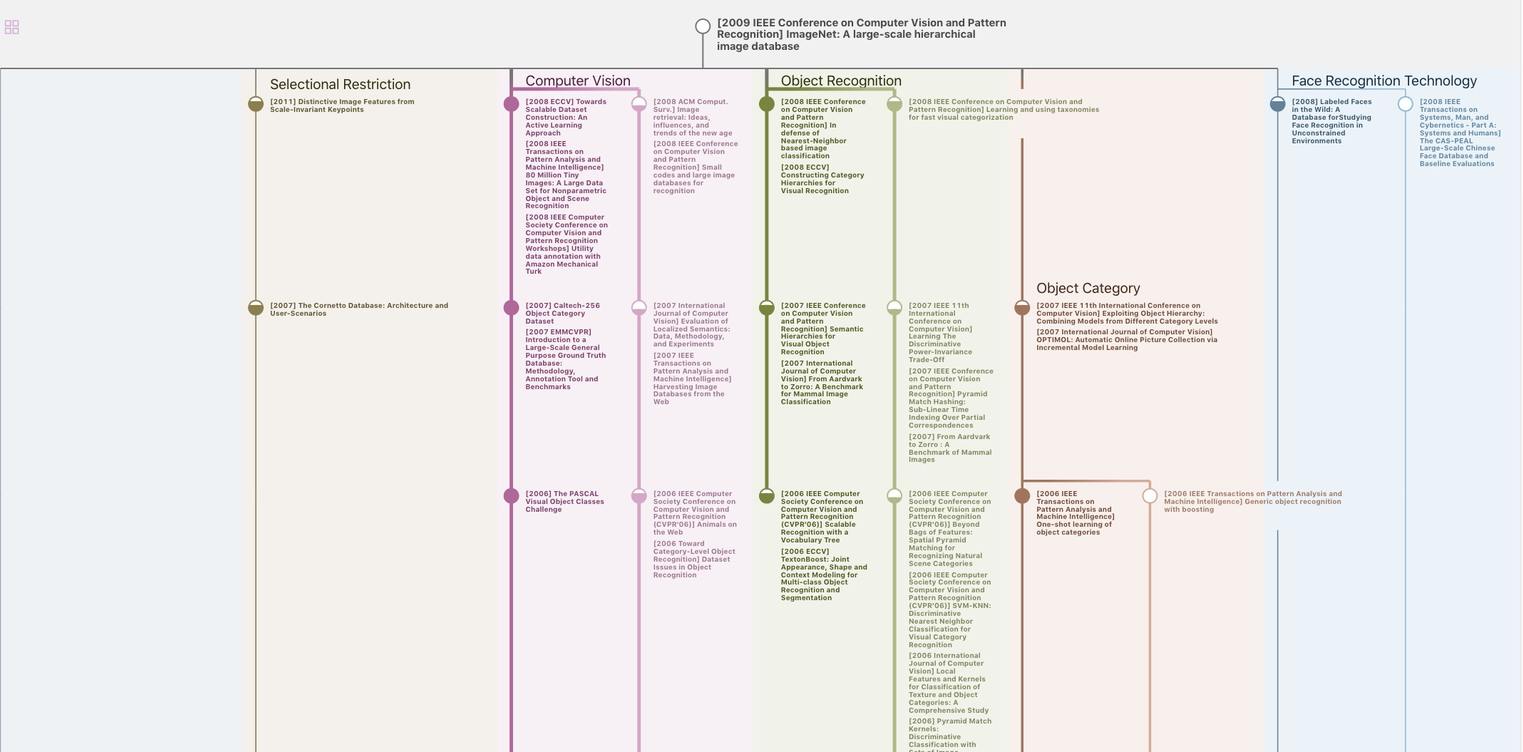
生成溯源树,研究论文发展脉络
Chat Paper
正在生成论文摘要