Data-driven decision support for individualised cardiovascular resuscitation in sepsis: a scoping review and primer for clinicians
medRxiv (Cold Spring Harbor Laboratory)(2023)
摘要
Background We conducted a scoping review of machine learning systems that inform individualised cardiovascular resuscitation of adults in hospital with sepsis. Our study reviews the resuscitation tasks that the systems aim to assist with, system robustness and potential to improve patient care, and progress towards deployment in clinical practice. We assume no expertise in machine learning from the reader and introduce technical concepts where relevant.
Methods This study followed the Preferred Reporting Items for Systematic reviews and Meta-Analyses extension for Scoping Reviews guidance. MEDLINE, EMBASE, Scopus, ClinicalTrials.gov, arXiv, bioRxiv and medRxiv were systematically searched up to September 2021. We present a narrative synthesis of the included studies, which also aims to equip clinicians with an understanding of the foundational machine learning concepts necessary to interpret them.
Results 73 studies were included with 80% published after 2018. Supervised learning systems were often used to predict septic shock onset. Reinforcement learning systems were increasingly popular in the last five years, and were used to guide specific dosing of fluids and vasopressors. A minority of studies proposed systems containing biological models augmented with machine learning. Sepsis and septic shock were heterogeneously defined and 63% of studies derived their systems using a single dataset. Most studies performed only retrospective internal validation, with no further steps taken towards translating their proposed systems into clinical practice.
Conclusions Machine learning systems can theoretically match, or even exceed, human performance when predicting patient outcomes and choosing the most suitable cardiovascular treatment strategy in sepsis. However, with some notable exceptions, the vast majority of systems to date exist only as proof of concept, with significant barriers to translation.
### Competing Interest Statement
FJRC, MN, PF and ZB, SH, AAF and ACG have no competing interests to declare. MK has received consulting fees from Philips Healthcare, and speaker honoraria from GE Healthcare.
### Clinical Protocols
### Funding Statement
FJRC is supported by a Wellcome Trust 4i Clinical PhD Fellowship (222882/Z/21/Z). MN and PF are supported by the UKRI CDT in AI for Healthcare (http://ai4health.io; grant EP/S023283/1). ZB is supported by a National Institute for Health Research (NIHR) Academic Clinical Fellowship. MK and ACG are supported by the NIHR Imperial Biomedical Research Centre. ACG is supported by the NIHR (RP-2015-06-018). AAF is supported by a UKRI Turing AI Fellowship (EP/V025449/1). SH is supported by the NIHR University College London Hospitals Biomedical Research Centre, and by University College London Collaborative Healthcare Innovation through Mathematics, EngineeRing and AI (UCL CHIMERA, EPSRC award EP/T017791/1).
### Author Declarations
I confirm all relevant ethical guidelines have been followed, and any necessary IRB and/or ethics committee approvals have been obtained.
Yes
I confirm that all necessary patient/participant consent has been obtained and the appropriate institutional forms have been archived, and that any patient/participant/sample identifiers included were not known to anyone (e.g., hospital staff, patients or participants themselves) outside the research group so cannot be used to identify individuals.
Yes
I understand that all clinical trials and any other prospective interventional studies must be registered with an ICMJE-approved registry, such as ClinicalTrials.gov. I confirm that any such study reported in the manuscript has been registered and the trial registration ID is provided (note: if posting a prospective study registered retrospectively, please provide a statement in the trial ID field explaining why the study was not registered in advance).
Yes
I have followed all appropriate research reporting guidelines, such as any relevant EQUATOR Network research reporting checklist(s) and other pertinent material, if applicable.
Yes
All extracted study data are included in Additional file 2.
* EMBASE
: Excerpta Medica Database
ICU
: Intensive care unit
IQR
: Interquartile range
MEDLINE
: Medical Literature Analysis and Retrieval System Online
MIMIC
: Medical Information Mart for Intensive Care
PRESS
: Peer review of electronic search strategies
PRISMA-ScR
: Preferred reporting items for systematic reviews and meta-analyses, extension for scoping reviews
RL
: Reinforcement learning
SOFA
: Sequential organ failure assessment
更多查看译文
关键词
individualised cardiovascular resuscitation,sepsis,decision support,data-driven
AI 理解论文
溯源树
样例
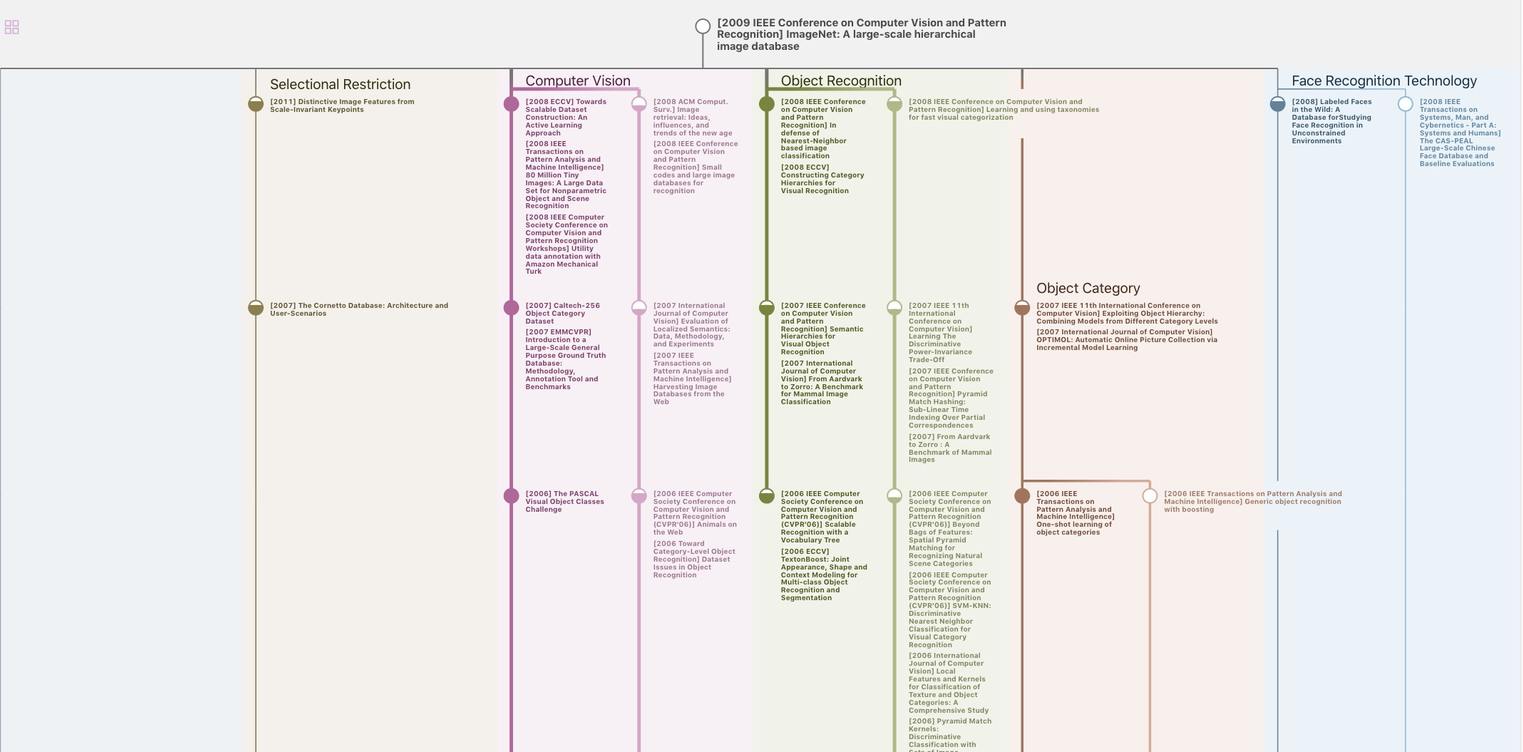
生成溯源树,研究论文发展脉络
Chat Paper
正在生成论文摘要