Clusters of multiple long-term conditions in three UK datasets: a latent class analysis
medRxiv (Cold Spring Harbor Laboratory)(2023)
摘要
Introduction Latent class analysis (LCA) can be used to identify subgroups within populations based on unobserved variables. LCA can be used to explore whether certain long-term conditions (LTC) occur together more frequently than others in patients with multiple-long term conditions. In this manuscript we present findings from applying LCA in three large-scale UK databanks.
Methods We applied LCA to three different UK databanks: Secure Anonymised Information Linkage databank [SAIL], UK Biobank, and Understanding Society: the UK Household Longitudinal Study [UKHLS] and four different age groups: 18-36, 37-54, 55-73, and 74+ years. The optimal number of classes in each LCA was determined using maximum likelihood. Sample size adjusted Bayesian Information Criterion (aBIC) was used to assess model fit and elbow plots and model entropy were used to assess the best number of latent classes in each model.
Results Between three to six clusters were identified in the different datasets and age groups. Although different in detail, similar types of clusters were identified between datasets and age groups which combine disorders around similar systems incl. Cardiometabolic clusters, Pulmonary clusters, Mental health clusters, Painful conditions clusters, and cancer clusters.
### Competing Interest Statement
The authors have declared no competing interest.
### Funding Statement
This work was funded by the UK National Institute for Health and Care Research (NIHR). The funders of the study had no role in study design, data collection, data analysis, data interpretation, or writing of the manuscript.
### Author Declarations
I confirm all relevant ethical guidelines have been followed, and any necessary IRB and/or ethics committee approvals have been obtained.
Yes
The details of the IRB/oversight body that provided approval or exemption for the research described are given below:
This study is part of an ongoing NIHR-funded Research project Personalised exercise rehabilitation for people with multiple long-term conditions (PERFORM). This study used data from UK Biobank, the Secure Anonymised Information Linkage databank (SAIL), and Understanding Society: the UK Household Longitudinal Study (UKHLS) The UK Biobank has full ethical approval from the NHS National Research Ethics Service (16/NW/0274). This study was conducted as part of UK Biobank Project 14151. The use and analysis of SAIL data was approved by the SAIL information governance review panel (Project 0830). UKHLS data access and use was granted by the UK Data Service (Project ID: 221571).
I confirm that all necessary patient/participant consent has been obtained and the appropriate institutional forms have been archived, and that any patient/participant/sample identifiers included were not known to anyone (e.g., hospital staff, patients or participants themselves) outside the research group so cannot be used to identify individuals.
Yes
I understand that all clinical trials and any other prospective interventional studies must be registered with an ICMJE-approved registry, such as ClinicalTrials.gov. I confirm that any such study reported in the manuscript has been registered and the trial registration ID is provided (note: if posting a prospective study registered retrospectively, please provide a statement in the trial ID field explaining why the study was not registered in advance).
Yes
I have followed all appropriate research reporting guidelines, such as any relevant EQUATOR Network research reporting checklist(s) and other pertinent material, if applicable.
Yes
Access to data from Biobank can be requested via the UK Biobank Access Management System Access to SAIL data can be requested via the independent Information Governance Review Panel Access to UKHLS data can be requested via UK Data Service
更多查看译文
关键词
uk datasets,conditions,long-term
AI 理解论文
溯源树
样例
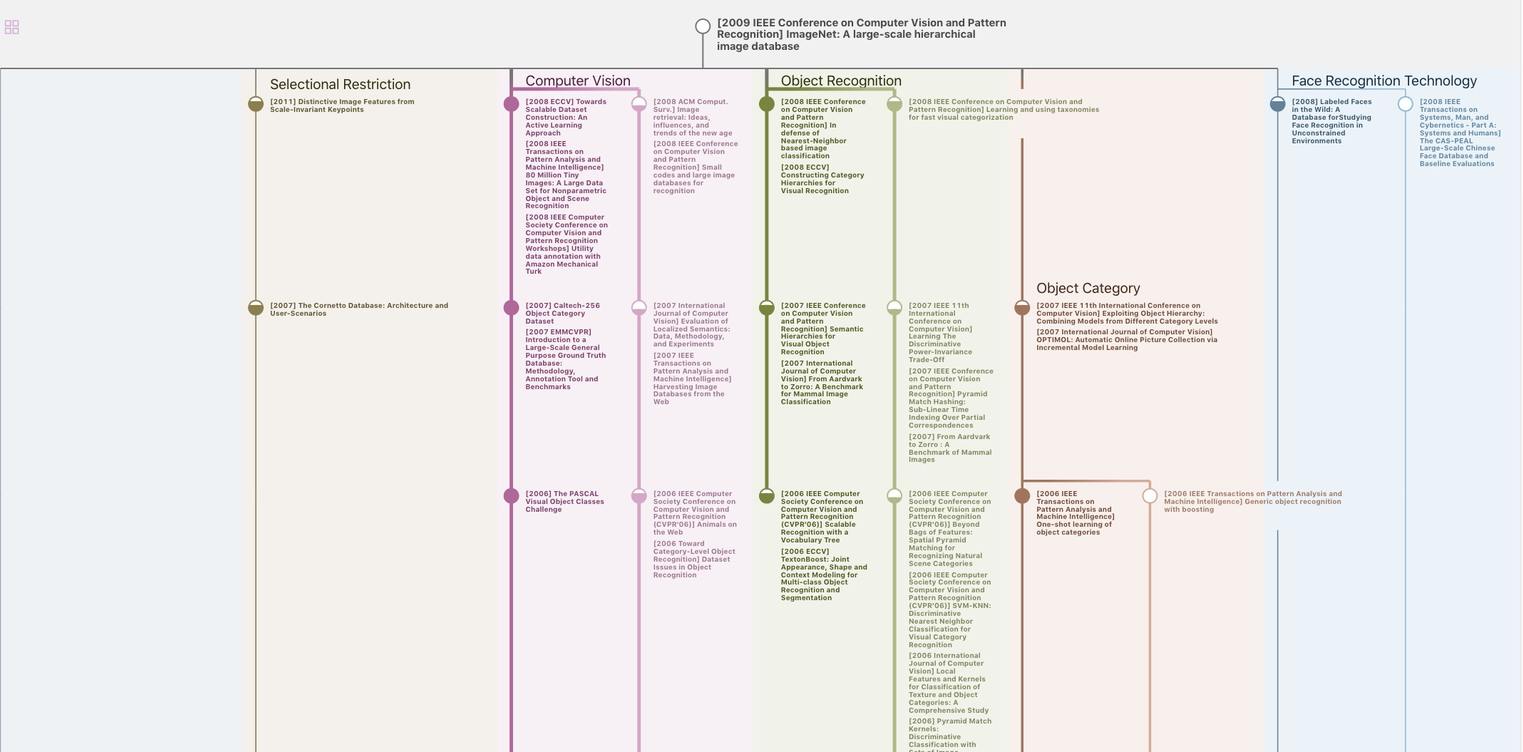
生成溯源树,研究论文发展脉络
Chat Paper
正在生成论文摘要