Evaluation of an artificial intelligence model for identification of intracranial hemorrhage subtypes on computed tomography of the head
medrxiv(2023)
摘要
Importance Intracranial hemorrhage is a critical finding on computed tomography (CT) of the head.
Objective This study compared the accuracy of an AI model (Annalise Enterprise CTB) to consensus neuroradiologist interpretations in detecting four hemorrhage subtypes: acute subdural/epidural hematoma, acute subarachnoid hemorrhage, intra-axial hemorrhage and intraventricular hemorrhage.
Design A retrospective standalone performance assessment was conducted on datasets of non-contrast CT head cases acquired between 2016 and 2022 for each hemorrhage subtype.
Setting The cases were obtained from five hospitals in the United States.
Participants The cases were obtained from patients aged 18 years or older. The positive cases were selected based on the original clinical reports using natural language processing and manual confirmation. The negative cases were selected by taking the next negative case acquired from the same CT scanner after positive cases.
Interventions Each case was interpreted independently by up to three neuroradiologists to establish consensus interpretations. Each case was then interpreted by the AI model for the presence of the relevant hemorrhage subtype. The neuroradiologists were provided with the entire CT study. The AI model separately received thin (≤1.5mm) and/or thick (>1.5 and ≤5mm) axial series.
Results The four cohorts included 571 cases for acute subdural/epidural hematoma, 310 cases for acute subarachnoid hemorrhage, 926 cases for intra-axial hemorrhage and 199 cases for intraventricular hemorrhage. The AI model identified acute subdural/epidural hematoma with area under the curve (AUC) 0.973 (95% confidence interval (CI), 0.958-0.984) on thin series and 0.942 (95% CI, 0.921-0.959) on thick series; acute subarachnoid hemorrhage with AUC 0.993 (95% CI, 0.984-0.998) on thin series and 0.966 (95% CI, 0.945-0.983) on thick series; intra-axial hemorrhage with AUC 0.969 (95% CI, 0.956-0.980) on thin series and 0.966 (95% CI, 0.953-0.976) on thick series; and intraventricular hemorrhage with AUC 0.987 (95% CI, 0.969-0.997) on thin series and 0.983 (95% CI, 0.968-0.994) on thick series. Each finding had at least one operating point with sensitivity and specificity greater than 80%.
Conclusions and Relevance The assessed AI model accurately identified intracranial hemorrhage subtypes in this CT dataset. Its use could assist the clinical workflow especially through enabling triage of abnormal CTs.
Question Does a commercial artificial intelligence model accurately identify intracranial hemorrhage subtypes on computed tomography (CT) of the head?
Findings This retrospective study used non-contrast CT studies to compare artificial intelligence model outputs to consensus neuroradiologist interpretations. The model was provided with either thin (≤1.5mm) or thick (>1.5 and ≤5mm) series. The model detected each of acute subdural/epidural hematoma, acute subarachnoid hemorrhage, intra-axial hemorrhage and intraventricular hemorrhage with sensitivity and specificity greater than 80%.
Meaning This artificial intelligence model could assist radiologists through its accurate detection of intracranial hemorrhage subtypes.
### Competing Interest Statement
This study was funded by Annalise-AI. JMH, BCB, INC, SFM, JKC, AG, EL, ALM, ASS, KB, JC, SP, SR, KJD, WAM are employees of Mass General Brigham and/or Massachusetts General Hospital, which had received institutional funding from Annalise-AI for the study.
### Funding Statement
This study was funded by Annalise-AI. JMH, BCB, INC, SFM, JKC, AG, EL, ALM, ASS, KB, JC, SP, SR, KJD, WAM are employees of Mass General Brigham and/or Massachusetts General Hospital, which had received institutional funding from Annalise-AI for the study.
### Author Declarations
I confirm all relevant ethical guidelines have been followed, and any necessary IRB and/or ethics committee approvals have been obtained.
Yes
The details of the IRB/oversight body that provided approval or exemption for the research described are given below:
This study was approved by the Mass General Brigham Institutional Review Board with waiver of informed consent.
I confirm that all necessary patient/participant consent has been obtained and the appropriate institutional forms have been archived, and that any patient/participant/sample identifiers included were not known to anyone (e.g., hospital staff, patients or participants themselves) outside the research group so cannot be used to identify individuals.
Yes
I understand that all clinical trials and any other prospective interventional studies must be registered with an ICMJE-approved registry, such as ClinicalTrials.gov. I confirm that any such study reported in the manuscript has been registered and the trial registration ID is provided (note: if posting a prospective study registered retrospectively, please provide a statement in the trial ID field explaining why the study was not registered in advance).
Yes
I have followed all appropriate research reporting guidelines, such as any relevant EQUATOR Network research reporting checklist(s) and other pertinent material, if applicable.
Yes
The AI model is part of the FDA cleared Annalise Enterprise CTB Triage Trauma device. The test dataset generated for this study contains protected patient information. Some data may be available for research purposes from the corresponding author upon reasonable request.
更多查看译文
关键词
intracranial hemorrhage subtypes,artificial intelligence,tomography,artificial intelligence model,head
AI 理解论文
溯源树
样例
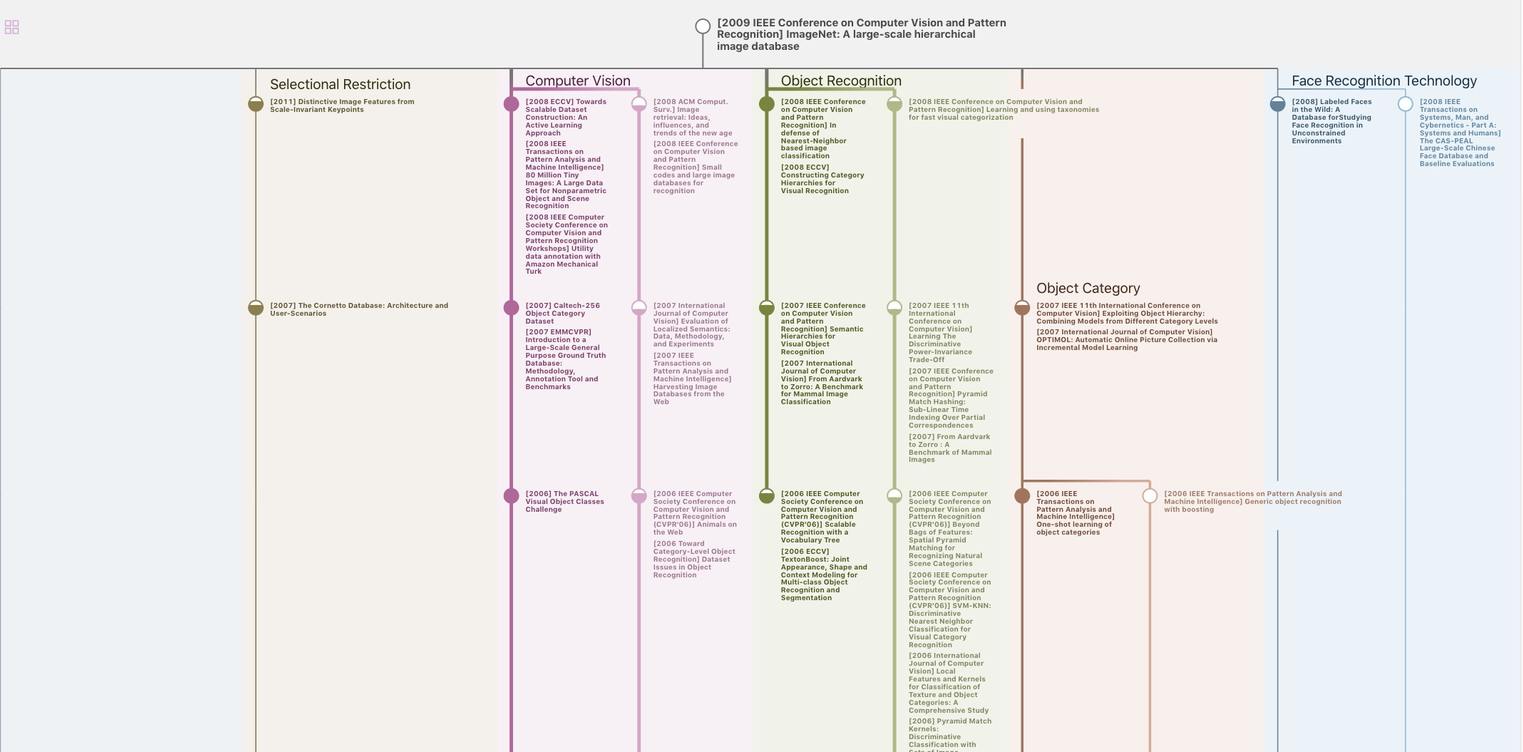
生成溯源树,研究论文发展脉络
Chat Paper
正在生成论文摘要