Towards mitigating health inequity via machine learning: a nationwide cohort study to develop and validate ethnicity-specific models for prediction of cardiovascular disease risk in COVID-19 patients
medRxiv (Cold Spring Harbor Laboratory)(2023)
摘要
Background Emerging data-driven technologies in healthcare, such as risk prediction models, hold great promise but also pose challenges regarding potential bias and exacerbation of existing health inequalities, which have been observed across diseases such as cardiovascular disease (CVD) and COVID-19. This study addresses the impact of ethnicity in risk prediction modelling for cardiovascular events following SARS-CoV-2 infection and explores the potential of ethnicity-specific models to mitigate disparities.
Methods This retrospective cohort study utilises six linked datasets accessed through National Health Service (NHS) England’s Secure Data Environment (SDE) service for England, via the BHF Data Science Centre’s CVD-COVID-UK/COVID-IMPACT Consortium. Inclusion criteria were established, and demographic information, risk factors, and ethnicity categories were defined. Four feature selection methods (LASSO, Random Forest, XGBoost, QRISK) were employed and ethnicity-specific prediction models were trained and tested using logistic regression. Discrimination (AUROC) and calibration performance were assessed for different populations and ethnicity groups.
Findings Several differences were observed in the models trained on the whole study cohort vs ethnicity-specific groups. At the feature selection stage, ethnicity-specific models yielded different selected features. AUROC discrimination measures showed consistent performance across most ethnicity groups, with QRISK-based models performing relatively poorly. Calibration performance exhibited variation across ethnicity groups and age categories. Ethnicity-specific models demonstrated the potential to enhance calibration performance for certain ethnic groups.
Interpretation This research highlights the importance of considering ethnicity in risk prediction modelling to ensure equitable healthcare outcomes. Differences in selected features and asymmetric calibration across ethnicities underscore the necessity of tailored approaches. Ethnicity-specific models offer a pathway to addressing disparities and improving model performance. The study emphasises the role of data-driven technologies in either alleviating or exacerbating existing health inequalities.
Evidence before this study Research has suggested that SARS-CoV-2 infections may have prognostic value in predicting later cardiovascular disease outcomes, two diseases where ethnicity-based health inequalities have been observed. Existing health inequalities are at risk of being exacerbated by bias in emerging data-driven technologies such as risk prediction models, and there currently exists no recommended practice to mitigate this issue. Model performances are not typically stratified by ethnic groups and, if reported, ethnic groups are often only included in higher-level categories that have been criticised for simplicity of definition and for missing key ethnic heterogeneity.
Added value of this study This study demonstrates the impact of including an in-depth consideration of ethnicity and its granularity in risk prediction modelling for cardiovascular event prediction in patients following a SARS-CoV-2 infection. This is one of, if not the first, set of models specifically built for and representative of all ethnic groups across an entire population, evaluating different practices to best mitigate ethnicity-based disparities in prediction algorithms. Moreover, ethnicity data has historically not been well captured, with as many as 1 in 3 individuals missing ethnicity data in their health records. With data linkage, this work is the first to analyse 96% complete ethnicity records in one of the world’s largest ethnically diverse routinely collected datasets.
Implications of all of the available evidence This study highlights the potential of tailoring feature selection, performance measures, and probability scores to different ethnic groups through ethnicity-specific risk prediction models to mitigate prediction bias. We identify differences between models trained on the global study populations to cohorts of specific ethnicities, and encourage the use of more granular ethnicity categories to capture the diversity of underlying populations. Such approaches will allow for newly developed data-driven tools to cater to the ethnic heterogeneity present between populations and ensure that emerging technologies translate into equitable health outcomes for everyone.
### Competing Interest Statement
The authors have declared no competing interest.
### Funding Statement
The British Heart Foundation Data Science Centre (grant No SP/19/3/34678, awarded to Health Data Research (HDR) UK) funded co-development (with NHS England) of the Secure Data Environment service for England, provision of linked datasets, data access, user software licences, computational usage, and data management and wrangling support, with additional contributions from the HDR UK Data and Connectivity component of the UK Government Chief Scientific Adviser's National Core Studies programme to coordinate national COVID-19 priority research. Consortium partner organisations funded the time of contributing data analysts, biostatisticians, epidemiologists, and clinicians.
### Author Declarations
I confirm all relevant ethical guidelines have been followed, and any necessary IRB and/or ethics committee approvals have been obtained.
Yes
The details of the IRB/oversight body that provided approval or exemption for the research described are given below:
The North East - Newcastle and North Tyneside 2 research ethics committee provided ethical approval for the CVD-COVID-UK/COVID-IMPACT research programme (REC no: 20/NE/0161) to access, within secure trusted research environments, unconsented, whole-population, de-identified data from electronic health records collected as part of patients' routine healthcare. Our project (proposal CCU037, short title: Minimising bias in ethnicity data) agreed the objectives of the consortium's ethical and regulatory approvals, and was authorized by the BHF Data Science Centre's Approvals and Oversight Board. Approved researchers (MPM, AD, SK) conducted the analyses within the NHS England's SDE via secure remote access. Ensuring the anonymity of individuals, only summarized-aggregated results that were manually reviewed by the NHS England safe outputs escrow service were exported from the SDE.
I confirm that all necessary patient/participant consent has been obtained and the appropriate institutional forms have been archived, and that any patient/participant/sample identifiers included were not known to anyone (e.g., hospital staff, patients or participants themselves) outside the research group so cannot be used to identify individuals.
Yes
I understand that all clinical trials and any other prospective interventional studies must be registered with an ICMJE-approved registry, such as ClinicalTrials.gov. I confirm that any such study reported in the manuscript has been registered and the trial registration ID is provided (note: if posting a prospective study registered retrospectively, please provide a statement in the trial ID field explaining why the study was not registered in advance).
Yes
I have followed all appropriate research reporting guidelines, such as any relevant EQUATOR Network research reporting checklist(s) and other pertinent material, if applicable.
Yes
The data used in this study are available in NHS England’s Secure Data Environment (SDE) service for England, but as restrictions apply they are not publicly available (). The CVD-COVID-UK/COVID-IMPACT programme, led by the BHF Data Science Centre ( ), received approval to access data in NHS England’s SDE service for England from the Independent Group Advising on the Release of Data (IGARD) () via an application made in the Data Access Request Service (DARS) Online system (ref. DARS-NIC-381078-Y9C5K) (). The CVD-COVID-UK/COVID-IMPACT Approvals & Oversight Board ( ) subsequently granted approval to this project to access the data within NHS England’s SDE service for England. The de-identified data used in this study were made available to accredited researchers only. Those wishing to gain access to the data should contact bhfdsc{at}hdruk.ac.uk in the first instance.
更多查看译文
关键词
cardiovascular disease risk,nationwide cohort study,cohort study,cardiovascular disease,health inequity,ethnicity-specific
AI 理解论文
溯源树
样例
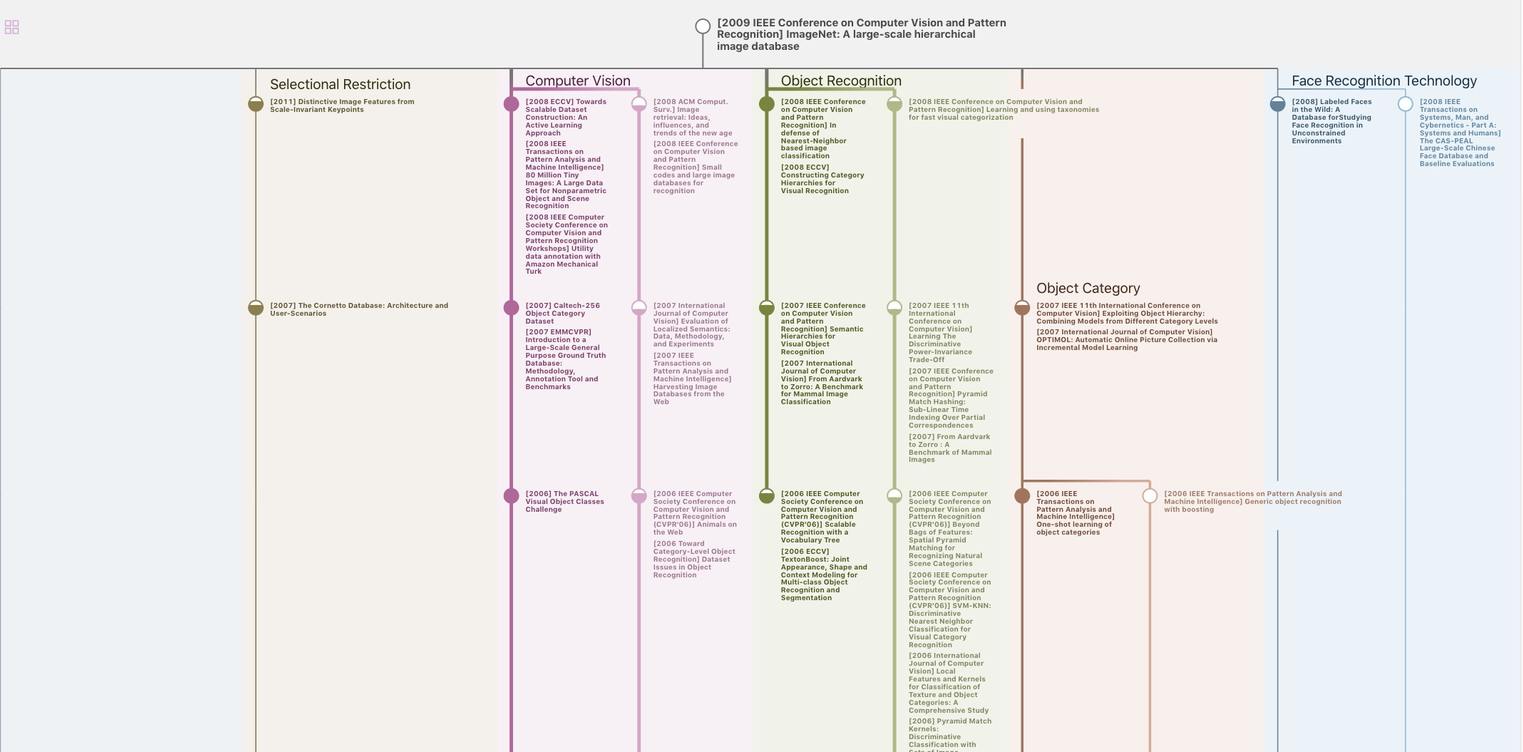
生成溯源树,研究论文发展脉络
Chat Paper
正在生成论文摘要