Deep Learning for Heart Sound Analysis: A Literature Review
medrxiv(2023)
摘要
Heart sound auscultation is a physical examination routinely used in clinical practice to identify potential cardiac abnormalities. However, accurate interpretation of heart sounds requires specialized training and experience, thereby limiting its generalizability. Deep learning, a subset of machine learning, involves training artificial neural networks to learn from large datasets and perform complex tasks related to intricate patterns, such as disease diagnosis, event prediction, and clinical decision-making. Over the past decade, deep learning has been successfully applied to heart sound analysis with remarkable achievements. Meanwhile, as heart sound analysis is gaining attention, many public and private heart sound datasets have been established for model training. The massive accumulation of heart sound data improves the performance of deep learning-based heart sound models and extends their clinical application scenarios. In this review, we will compile the commonly used datasets in heart sound analysis, introduce the fundamentals and state-of-the-art techniques in heart sound analysis and deep learning, and summarize the current applications of deep learning for heart sound analysis and their limitations for future improvement.
### Competing Interest Statement
The authors have declared no competing interest.
### Funding Statement
This study was funded by the National Natural Science Foundation of China (No. 62102008) and Peking University People's Hospital Scientific Research Development Funds (RDJP2022-39).
### Author Declarations
I confirm all relevant ethical guidelines have been followed, and any necessary IRB and/or ethics committee approvals have been obtained.
Yes
I confirm that all necessary patient/participant consent has been obtained and the appropriate institutional forms have been archived, and that any patient/participant/sample identifiers included were not known to anyone (e.g., hospital staff, patients or participants themselves) outside the research group so cannot be used to identify individuals.
Yes
I understand that all clinical trials and any other prospective interventional studies must be registered with an ICMJE-approved registry, such as ClinicalTrials.gov. I confirm that any such study reported in the manuscript has been registered and the trial registration ID is provided (note: if posting a prospective study registered retrospectively, please provide a statement in the trial ID field explaining why the study was not registered in advance).
Yes
I have followed all appropriate research reporting guidelines, such as any relevant EQUATOR Network research reporting checklist(s) and other pertinent material, if applicable.
Yes
The data availability is based on each literature discussed in the review.
* DL
: deep learning
PCG
: phonocardiogram
AS
: aortic stenosis
MR
: mitral regurgitation
MS
: mitral stenosis
MVP
: mitral valve prolapse
STFT
: Short-Time Fourier-Transform
MFCCs
: Mel-frequency Cepstral Coefficients
CEEMD
: Complementary Ensemble Empirical Mode Decomposition
TQWT
: Tunable-Q Wavelet Transform
CWT
: Continuous Wavelet Transform
CNNs
: convolutional neural networks
RNNs
: recurrent neural networks
NN
: neural network
ML
: machine learning
VHD
: Valvular heart disease
DNN
: Deep Neural Network
CHD
: Congenital heart disease
ASD
: atrial septal defects
VSD
: ventricular septal defects
PDA
: patent ductus arteriosus
TGNN
: Time Growing Neural Network
LVEF
: left ventricular ejection fraction
GRU
: gated recurrent unit
HFpEF
: Heart failure with preserved ejection fraction
HFrEF
: Heart failure with reduced ejection fraction
LVDD
: left ventricular diastolic dysfunction
CAD
: Coronary artery disease
RHD
: Rheumatic heart disease
BP
: blood pressure
PH
: pulmonary hypertension
PAP
: pulmonary artery pressure
更多查看译文
关键词
heart sound analysis,deep learning
AI 理解论文
溯源树
样例
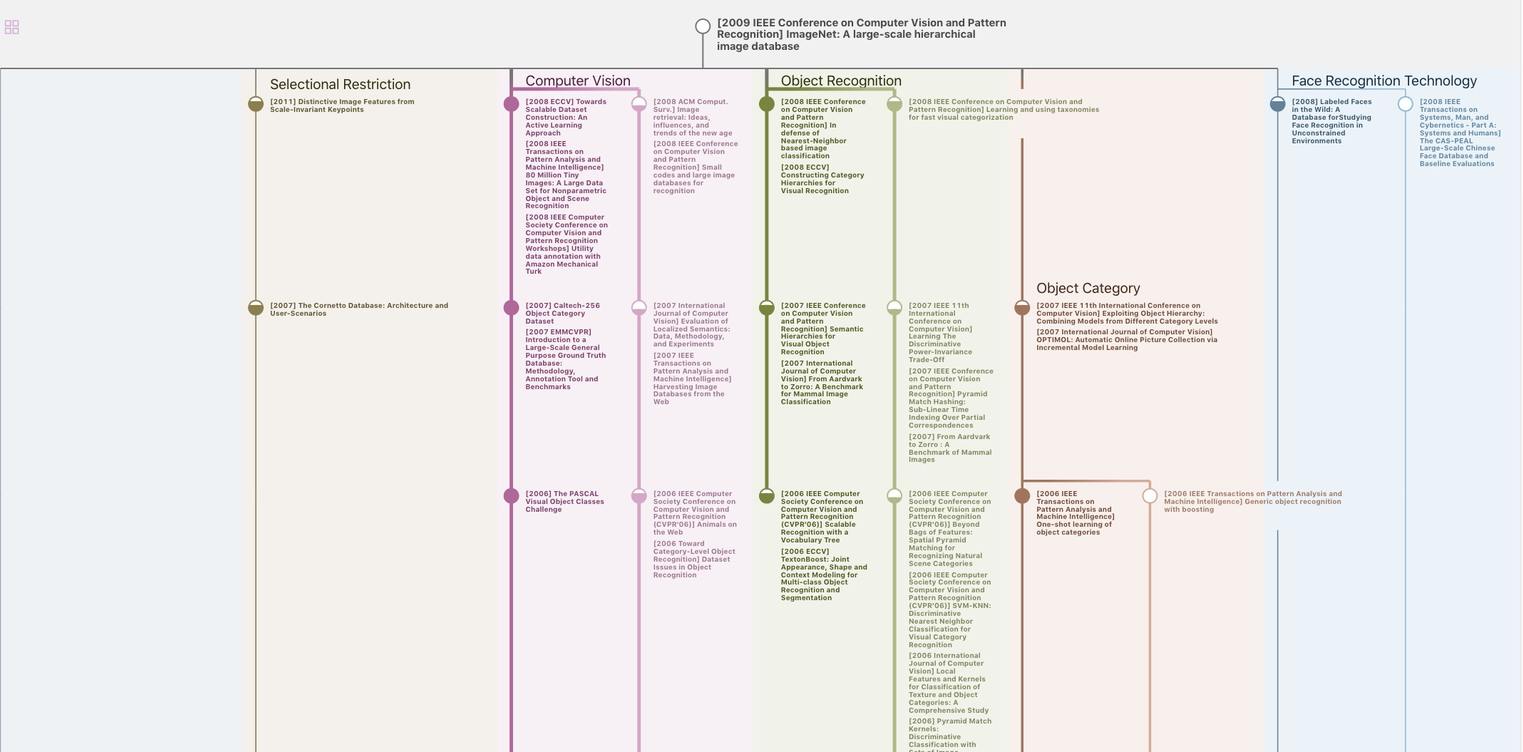
生成溯源树,研究论文发展脉络
Chat Paper
正在生成论文摘要