Predicting self-harm at one year in female prisoners: a retrospective cohort study using machine learning
medRxiv (Cold Spring Harbor Laboratory)(2023)
摘要
Background Self-harm and suicide are relatively overrepresented in incarcerated populations, especially in female prisons. Identifying those most at risk of significant self-harm could provide opportunities for effective, targeted interventions.
Aims To develop and validate a machine learning-based algorithm capable of achieving a clinically useful level of accuracy when predicting the risk of self-harm in female prisoners.
Method Data were available on 31 variables for 286 female prisoners from a single UK-based prison. This included sociodemographic factors, nature of the index offence, and responses to several psychometric assessment tools used at baseline. At 12-month follow-up any self-harm incidents were reported. A machine learning algorithm (CatBoost) to predict self-harm at one-year was developed and tested. To quantify uncertainty about the accuracy of the algorithm, the model building and evaluation process was repeated 2000 times and the distribution of results summarised.
Results The mean Area Under the Curve (AUC) for the model on unseen (validation) data was 0.92 (SD 0.04). Sensitivity was 0.83 (SD 0.07), specificity 0.94 (SD 0.03), positive predictive value 0.78 (SD 0.08) and the negative predictive value 0.95 (0.02). If the algorithm was used in this population, for every 100 women screened, this would equate to approximately 17 ‘true positives’ and five ‘false positives’.
Conclusions The accuracy of the algorithm was superior to those previously reported for predicting future self-harm in general and prison populations and likely to provide clinically useful levels of prediction. Research is needed to evaluate the feasibility of implementing this approach in a prison setting.
### Competing Interest Statement
The authors have declared no competing interest.
### Funding Statement
This study did not receive any funding
### Author Declarations
I confirm all relevant ethical guidelines have been followed, and any necessary IRB and/or ethics committee approvals have been obtained.
Yes
The details of the IRB/oversight body that provided approval or exemption for the research described are given below:
Ethics Committee at the University of York gave ethical approval for the original study.
I confirm that all necessary patient/participant consent has been obtained and the appropriate institutional forms have been archived, and that any patient/participant/sample identifiers included were not known to anyone (e.g., hospital staff, patients or participants themselves) outside the research group so cannot be used to identify individuals.
Yes
I understand that all clinical trials and any other prospective interventional studies must be registered with an ICMJE-approved registry, such as ClinicalTrials.gov. I confirm that any such study reported in the manuscript has been registered and the trial registration ID is provided (note: if posting a prospective study registered retrospectively, please provide a statement in the trial ID field explaining why the study was not registered in advance).
Yes
I have followed all appropriate research reporting guidelines, such as any relevant EQUATOR Network research reporting checklist(s) and other pertinent material, if applicable.
Yes
The analytic dataset and code used in this analysis are available from
更多查看译文
关键词
female prisoners,machine learning,cohort study,self-harm
AI 理解论文
溯源树
样例
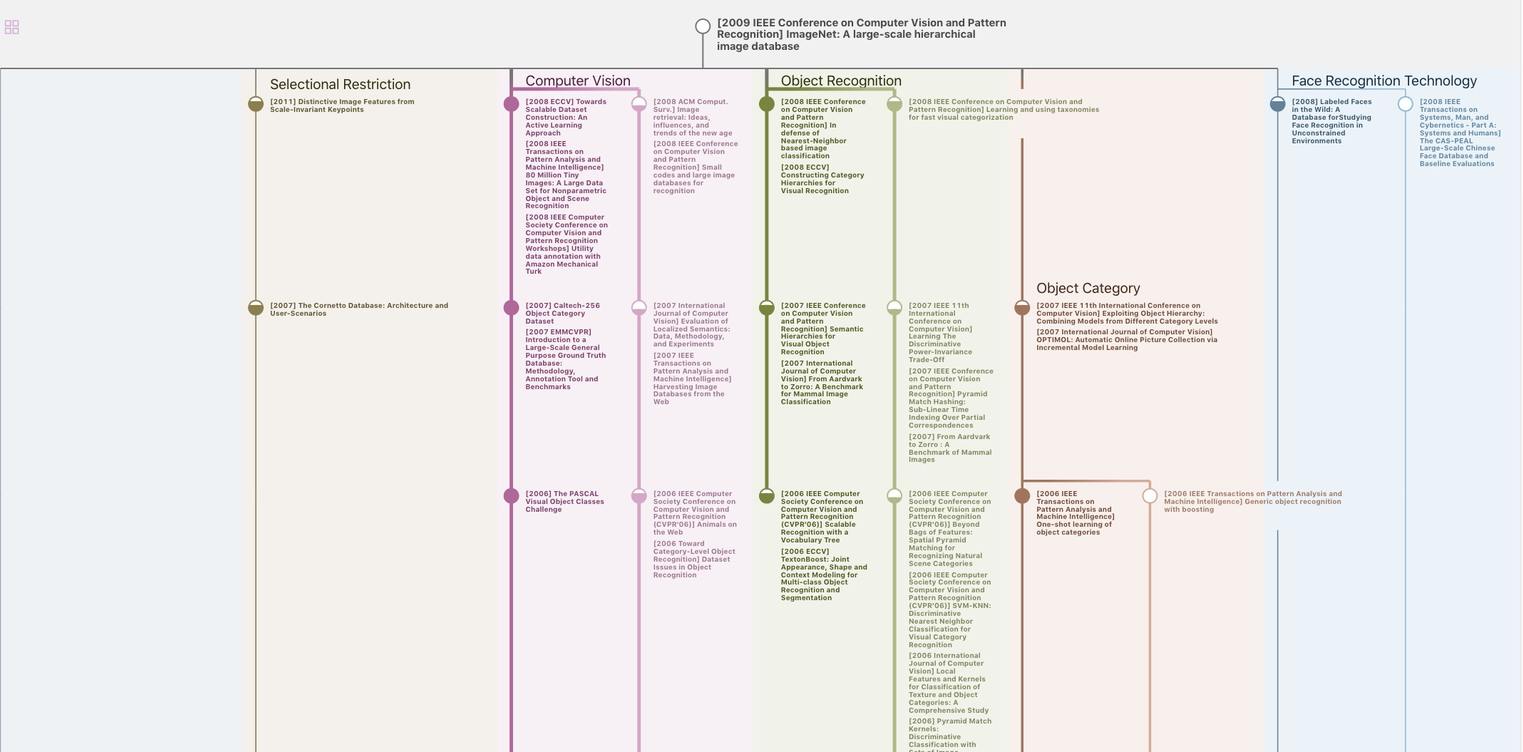
生成溯源树,研究论文发展脉络
Chat Paper
正在生成论文摘要