Thermal error modeling of motorized spindle considering the effect of milling head heat source
INTERNATIONAL JOURNAL OF ADVANCED MANUFACTURING TECHNOLOGY(2023)
摘要
The thermal behavior of the machine tool is an important cause of machining errors, and the thermal error prediction model can predict the errors in real time and improve the machining accuracy of the motorized spindle. Neural networks are widely used in thermal error modeling because of their fast learning speed, excellent prediction accuracy, and robustness. Firstly, the thermal characteristics are analyzed using the motorized spindle as the study object to determine its temperature field distribution. Secondly, the measurement point locations are selected based on the temperature field distribution, and the K -means++ algorithm and gray correlation analysis are used to reduce the 11 temperature measurement points to 4, which improved the input quality of the model. Finally, this paper combines GA and ACO to search the optimal parameters of the BP neural network to build a neural network thermal error prediction model, which overcomes the problems of slow convergence and the tendency to fall into local extremes. Compared with the radial basis function neural network (RBF neural network) model and ACO-BP neural network model, the average value of root mean square error (RMSE) of the Z -directional thermal errors predicted by the GA-ACO-BP neural network model is reduced by 45.3% and 58.3%; and the average value of mean absolute error (MAE) is decreased by 65.2% and 53.8%, respectively.
更多查看译文
关键词
K -means++ algorithm
AI 理解论文
溯源树
样例
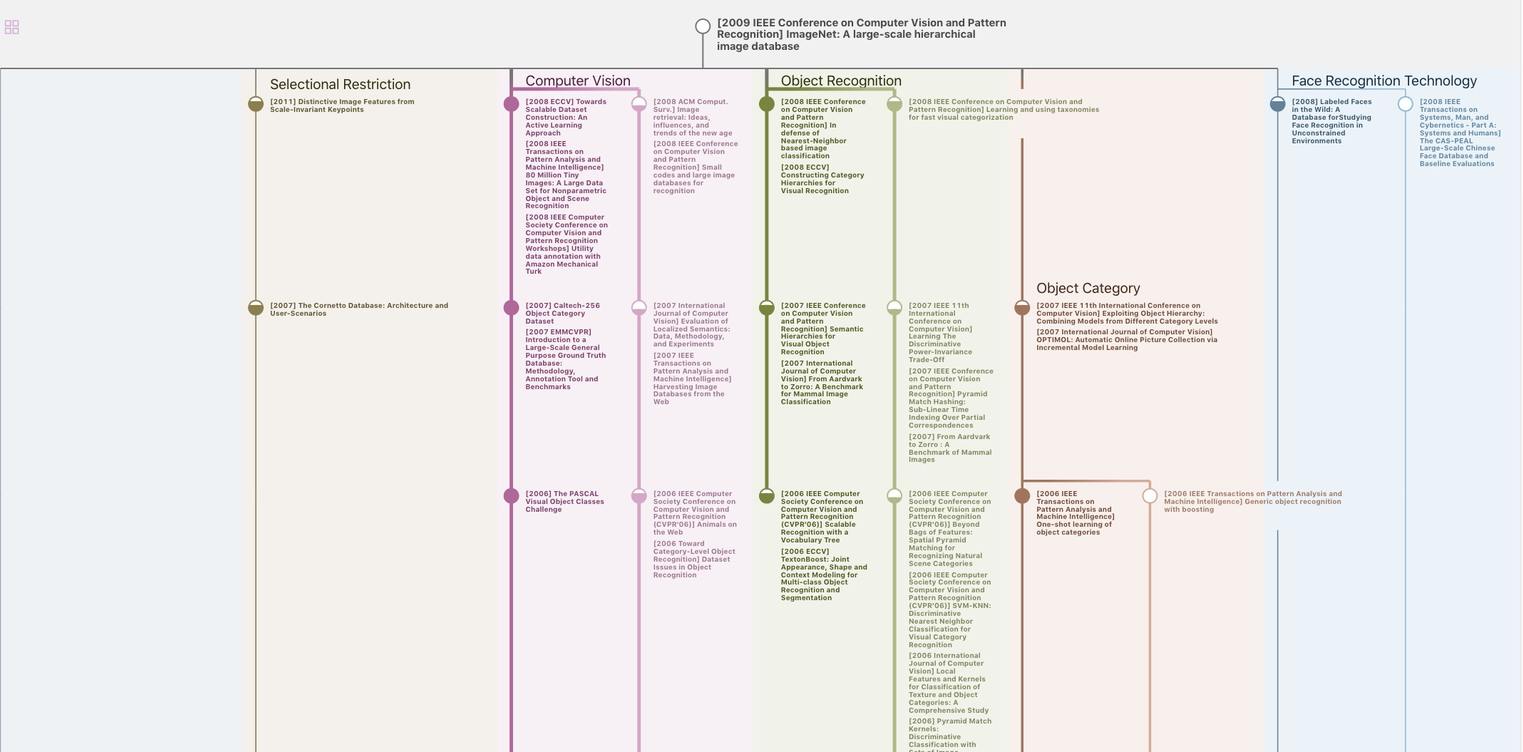
生成溯源树,研究论文发展脉络
Chat Paper
正在生成论文摘要