The scaling of mental computation in a sorting task.
Cognition(2023)
摘要
Many cognitive models provide valuable insights into human behavior. Yet the algorithmic complexity of candidate models can fail to capture how human reaction times scale with increasing input complexity. In the current work, we investigate the algorithms underlying human cognitive processes. Computer science characterizes algorithms by their time and space complexity scaling with problem size. We propose to use participants' reaction times to study how human computations scale with increasing input complexity. We tested this approach in a task where participants had to sort sequences of rectangles by their size. Our results showed that reaction times scaled close to linearly with sequence length and that participants learned and actively used latent structure whenever it was provided. This behavior was in line with a computational model that used the observed sequences to form hypotheses about the latent structures, searching through candidate hypotheses in a directed fashion. These results enrich our understanding of plausible cognitive models for efficient mental sorting and pave the way for future studies using reaction times to investigate the scaling of mental computations across psychological domains.
更多查看译文
AI 理解论文
溯源树
样例
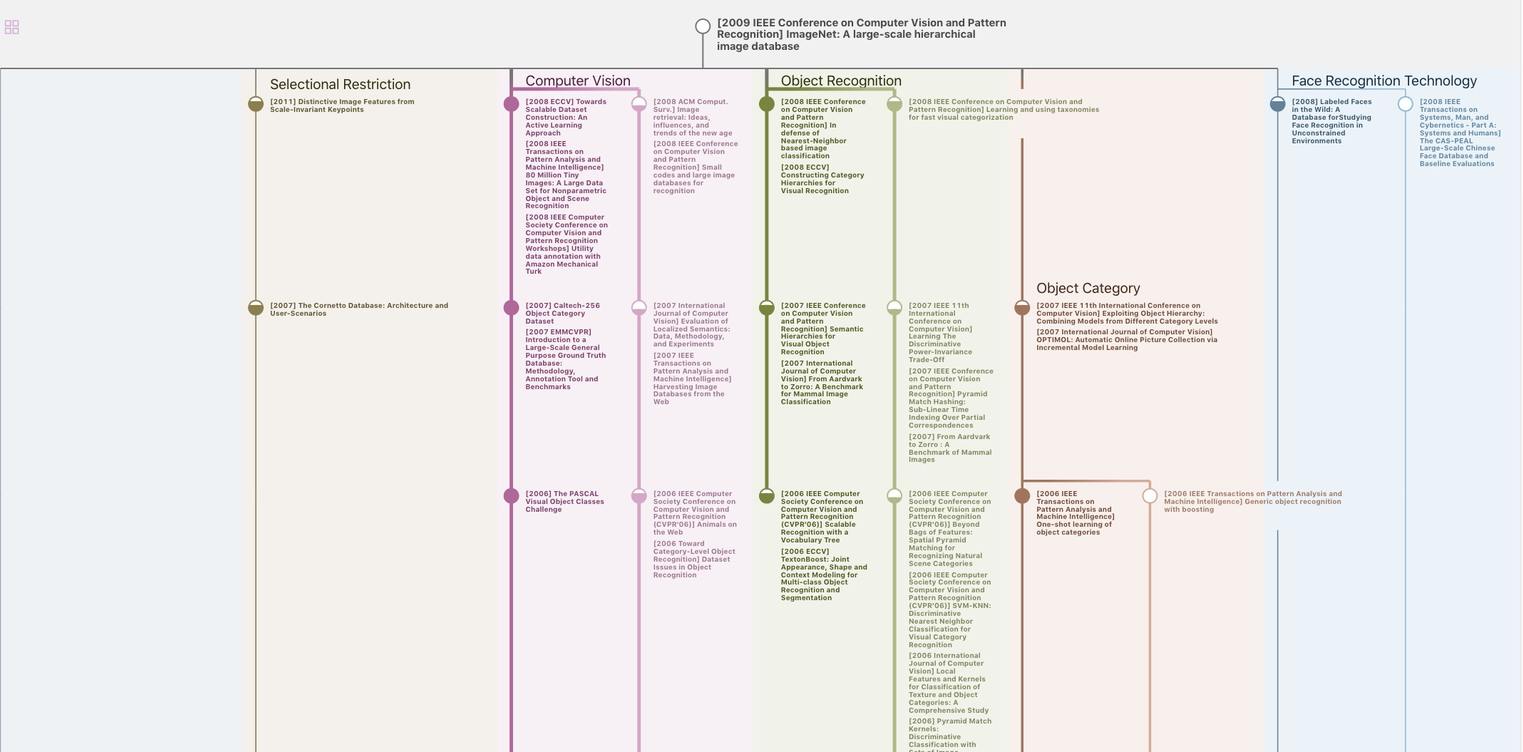
生成溯源树,研究论文发展脉络
Chat Paper
正在生成论文摘要