Automating Question Generation From Educational Text
ARTIFICIAL INTELLIGENCE XL, AI 2023(2023)
摘要
The use of question-based activities (QBAs) is wide-spread in education, traditionally forming an integral part of the learning and assessment process. In this paper, we design and evaluate an automated question generation tool for formative and summative assessment in schools. We present an expert survey of one hundred and four teachers, demonstrating the need for automated generation of QBAs, as a tool that can significantly reduce the workload of teachers and facilitate personalized learning experiences. Leveraging the recent advancements in generative AI, we then present a modular framework employing transformer based language models for automatic generation of multiple-choice questions (MCQs) from textual content. The presented solution, with distinct modules for question generation, correct answer prediction, and distractor formulation, enables us to evaluate different language models and generation techniques. Finally, we perform an extensive quantitative and qualitative evaluation, demonstrating trade-offs in the use of different techniques and models.
更多查看译文
关键词
Automatic question generation,Large language models,Generative AI,Distractors,Question-based activities,Multiple-choice questions
AI 理解论文
溯源树
样例
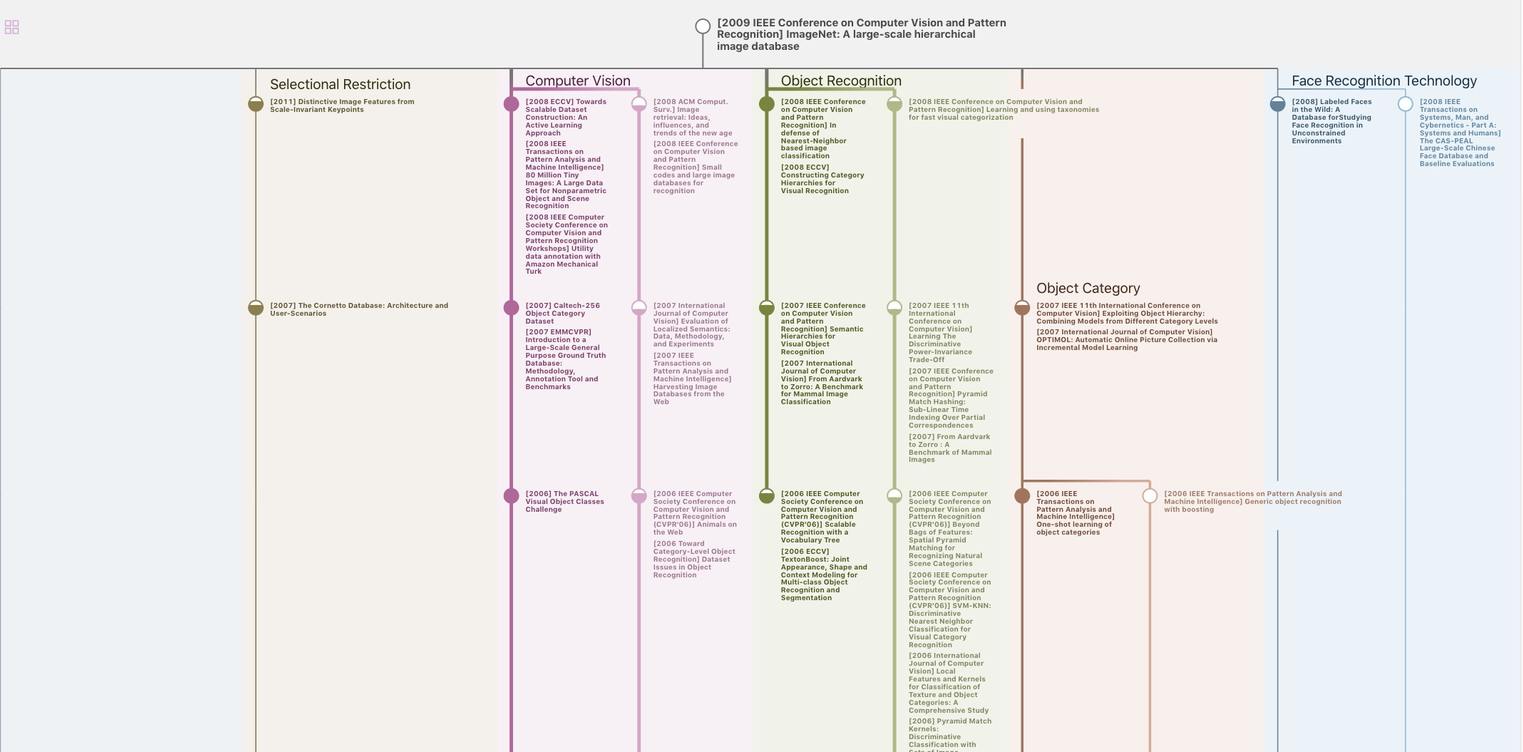
生成溯源树,研究论文发展脉络
Chat Paper
正在生成论文摘要