Locality-Preserving Directions for Interpreting the Latent Space of Satellite Image GANs
IEEE GEOSCIENCE AND REMOTE SENSING LETTERS(2024)
摘要
We present a locality-aware method for interpreting the latent space of wavelet-based generative adversarial networks (GANs), that can well capture the large spatial and spectral variability that is characteristic of satellite imagery. By focusing on preserving locality, the proposed method is able to decompose the weight-space of pre-trained GANs and recover interpretable directions that correspond to high-level semantic concepts (such as urbanization, structure density, flora presence)-that can subsequently be used for guided synthesis of satellite imagery. In contrast to typically used approaches that focus on capturing the variability of the weight-space in a reduced dimensionality space (i.e., based on principal component analysis (PCA)), we show that preserving locality leads to vectors with different angles, that are more robust to artifacts and can better preserve class information. Via a set of quantitative and qualitative examples, we further show that the proposed approach can outperform both baseline geometric augmentations, as well as global, PCA-based approaches for data synthesis in the context of data augmentation for satellite scene classification.
更多查看译文
关键词
Generative adversarial networks (GANs),interpretability,locality,remote sensing,wavelets
AI 理解论文
溯源树
样例
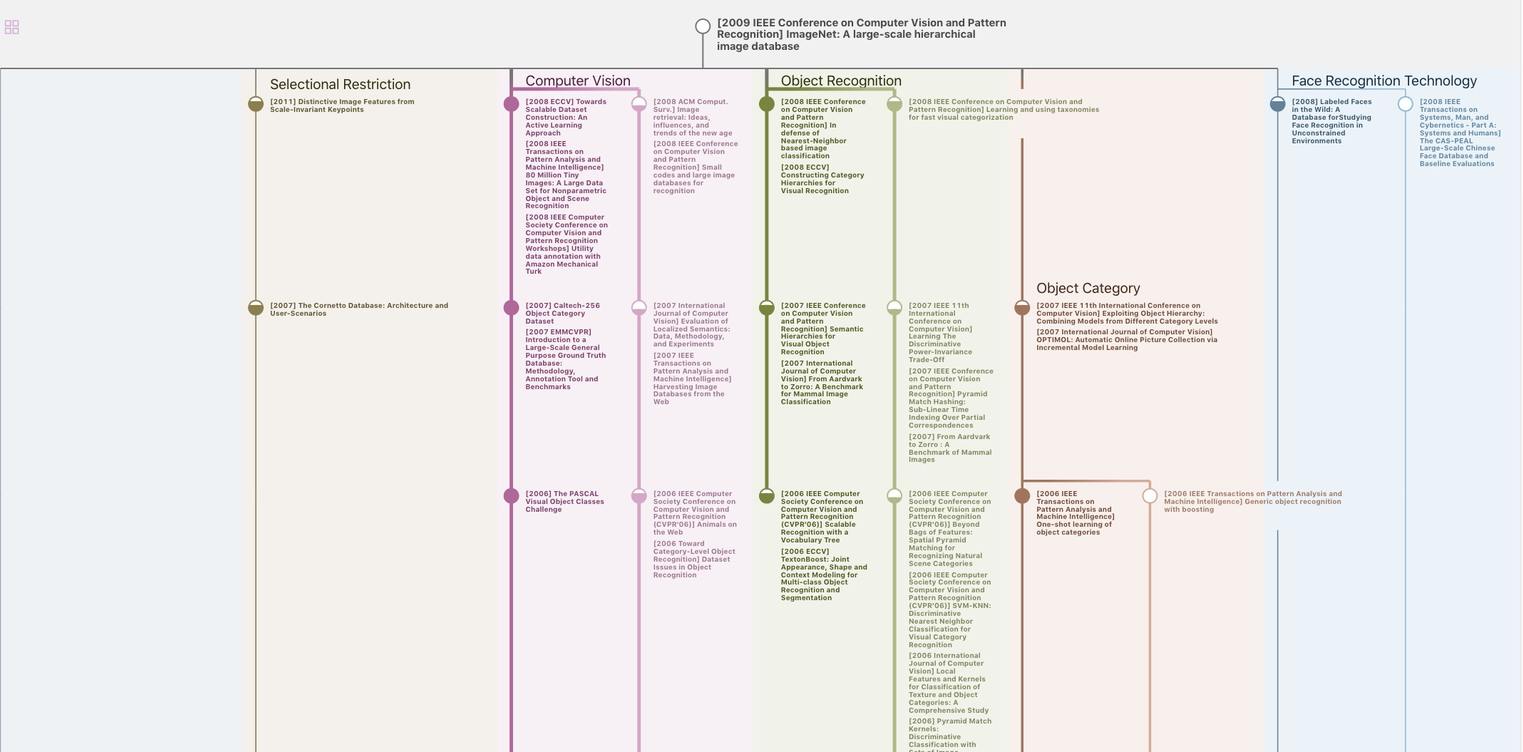
生成溯源树,研究论文发展脉络
Chat Paper
正在生成论文摘要