Physics of Language Models: Part 3.2, Knowledge Manipulation
CoRR(2023)
摘要
Language models can store vast amounts of factual knowledge, but their ability to use this knowledge for logical reasoning remains questionable. This paper explores a language model's ability to manipulate its stored knowledge during inference. We focus on four manipulation types: retrieval (e.g., "What is person A's attribute X"), classification (e.g., "Is A's attribute X even or odd?"), comparison (e.g., "Is A greater than B in attribute X?") and inverse search (e.g., "Which person's attribute X equals T?") We observe that pre-trained language models like GPT2/3/4 excel in knowledge retrieval but struggle with simple classification or comparison tasks unless Chain of Thoughts (CoTs) are employed during both training and inference. They also perform poorly in inverse knowledge search, irrespective of the prompts. Our primary contribution is a synthetic dataset for a controlled experiment that confirms these inherent weaknesses: a language model cannot efficiently manipulate knowledge from pre-training data, even when such knowledge is perfectly stored and fully extractable in the models, and despite adequate instruct fine-tuning.
更多查看译文
关键词
language models,knowledge,manipulation
AI 理解论文
溯源树
样例
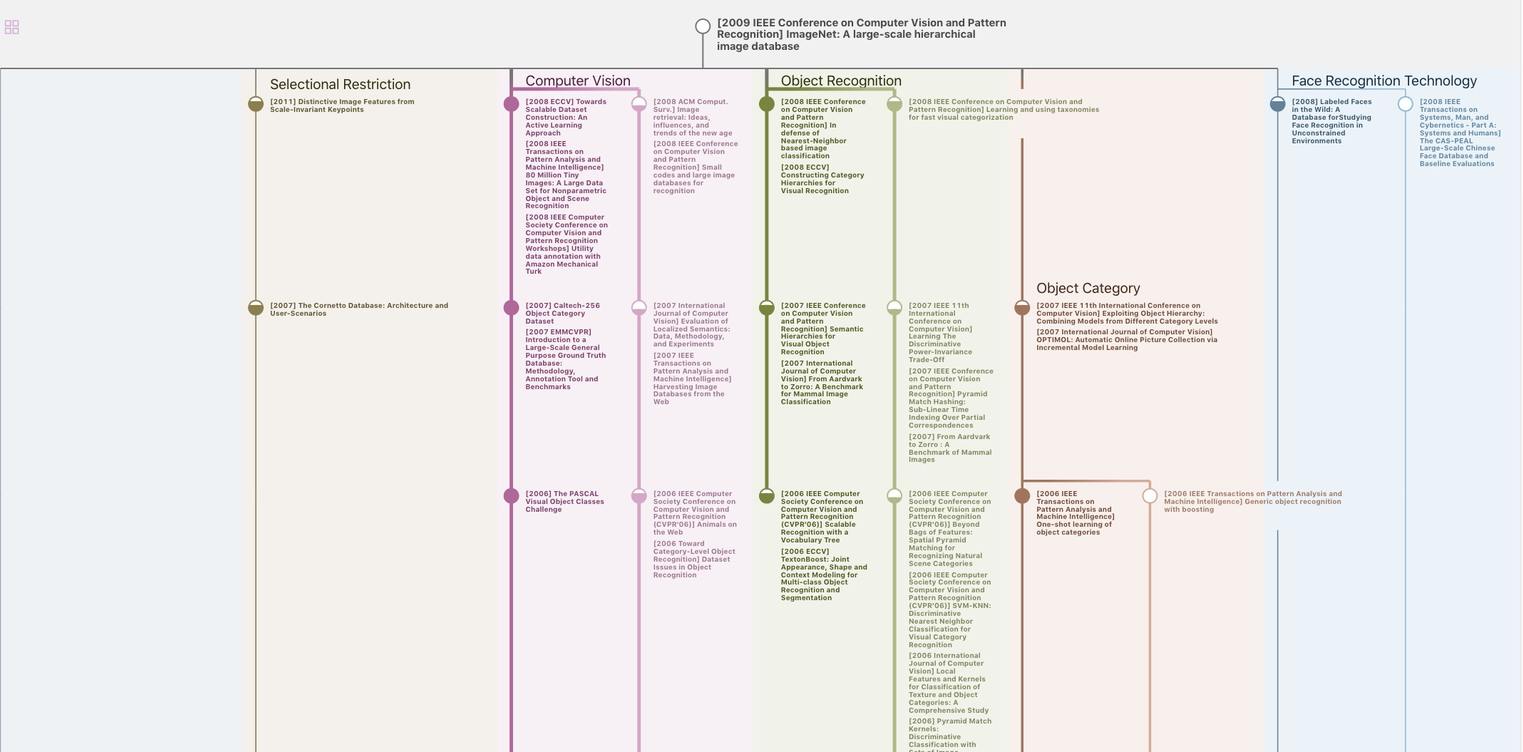
生成溯源树,研究论文发展脉络
Chat Paper
正在生成论文摘要