Deep Learning-Based Index Modulation for Underground Communications
IEEE Open Journal of the Communications Society(2023)
摘要
The world population is rapidly increasing, which in turn increases food needs. On the other hand, food production is the main cause of water withdrawal. Precision agriculture, based on the Internet of Underground Things (IoUT), has recently been proposed to reduce water withdrawals. IoUT comprises sensors and communication devices that are partially or fully submerged beneath the ground surface. However, the current technology used in IoUT is challenged by inefficient spectral and energy efficiency. This paper proposes a deep learning (DL)-based index modulation technique to increase spectral efficiency without raising the system’s bit error rate. The article decreases the peak-to-average power ratio (PAPR) and enhances energy efficiency by employing the X-transform time-domain synchronous index modulation (TDS-IM) orthogonal frequency division multiplexing (OFDM) in underground communication. Unlike the radio frequency channel, the underground channel is environment-dependent. Therefore, in this paper, we propose a DL receiver detector to eliminate such environmental dependency and simplify the system’s complexity. This proposal can establish new identification parameters, achieving accurate estimation of the modulated symbols even in harsh communication channels. The results of the simulation indicate the superior performance of the proposed scheme in terms of both spectral and energy efficiency compared to the benchmarks, as well as its ability to improve the system’s bit error rate.
更多查看译文
关键词
Underground communications,IoUT,17 IM,DL,energy efficiency,spectral efficiency
AI 理解论文
溯源树
样例
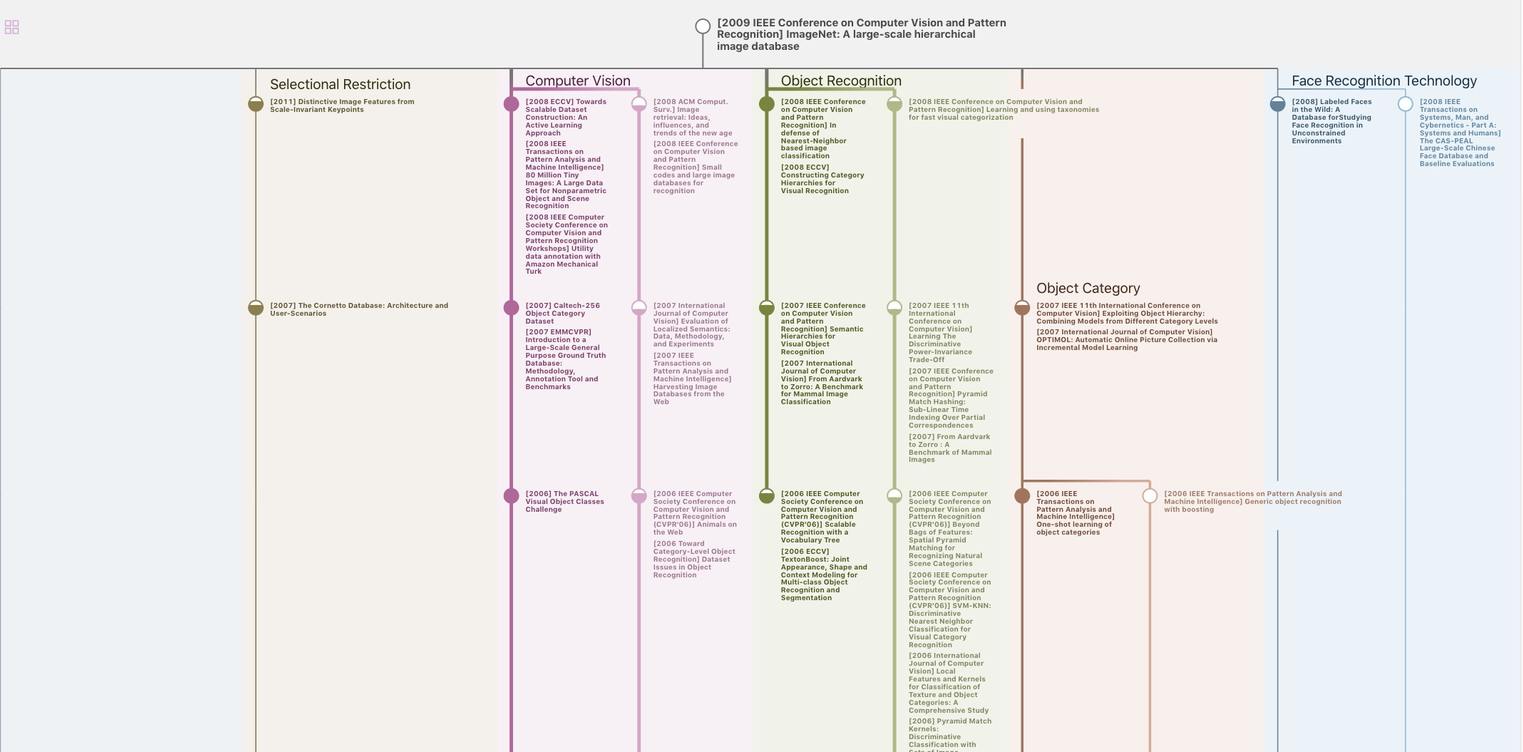
生成溯源树,研究论文发展脉络
Chat Paper
正在生成论文摘要