A Multi-Layer Long Short-Term Memory Neural Network on Battery State-of-Health Estimation with Transfer Learning
2023 IEEE 3rd International Conference on Industrial Electronics for Sustainable Energy Systems (IESES)(2023)
摘要
Due to the important part of batteries in industrial systems, its safety analysis has causes widespread attention from researchers, and its effective maintenance decision-making is needed. Data-driven state-of-health (SOH) estimation can provide useful information by monitoring historical data during the aging process, but it can be failed in the cross-domain scenarios due to the different data distributions. To tackle this issue, we propose a long short-term memory (LSTM) neural network with an additional fully connected dense as a basic predictor, and apply the pretrain and fine-tuning training algorithm to realize the high-performance prediction. By validated in two real-world datasets, we find that blindly expanding the training set may have a negative impact on model accuracy, and the proposed TL-LSTM can achieve a great performance under the cross-domain tasks.
更多查看译文
关键词
SOH Estimation,battery,transfer learning,long short-term memory
AI 理解论文
溯源树
样例
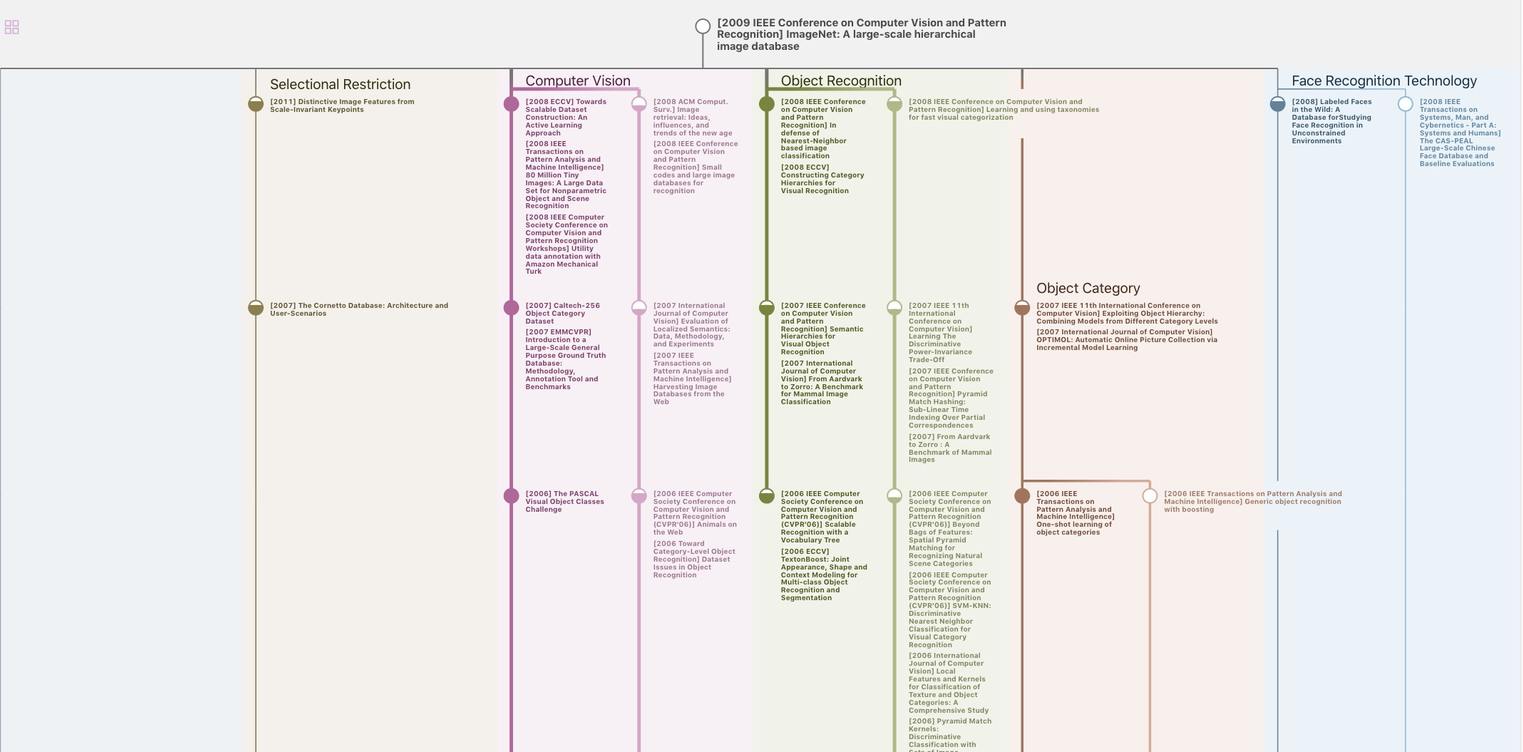
生成溯源树,研究论文发展脉络
Chat Paper
正在生成论文摘要