FlyPaw: Optimized Route Planning for Scientific UAVMissions
2023 IEEE 19th International Conference on e-Science (e-Science)(2023)
摘要
Many Internet of Things (IoT) applications require compute resources that cannot be provided by the devices themselves. On the other hand, processing of the data generated by IoT devices and sensors often has to be performed in real- or near real-time, i.e., with stringent latency requirements in constrained environments (e.g., intermittent network connectivity and limited power envelopes). Examples of such scenarios are autonomous vehicles in the form of cars and drones where the processing and analysis of observational data (e.g., video feeds) need to be performed expeditiously to allow for safe operation of the vehicles and to deliver the results in a timely fashion to the stakeholders of the mission. To support the compute and timeliness requirements of such applications, it is essential to include suitable edge resources to process these workflows, and to develop an end-to-end system that can route the vehicles dynamically and process and deliver mission-critical data and analyzed results. In this paper, we develop and evaluate a dynamic scheduling approach that considers complex tradeoffs between real-time constraints, network availability, and latency sensitivity of the mission. We devise an optimized route planning and data transmission schedule for drone flights. The scheduling algorithm is encapsulated in a novel end-to-end architecture (FlyPaw) and an associated adaptive drone mission control system, which enables deployment and management of an integrated cyberphysical system (CPS) – from real drone testbed to base stations to edge-to-cloud resources. The planning algorithm takes into account measured network communication characteristics, estimated uncertainties of future data link connectivity, and data timeliness requirements of the mission to prioritize candidate decision tree solutions based on a risk metric derived from Sharpe's ratio. Our results show that for given task sets, Net Time to Retrieve, our metric describing the time required to perform end-to-end collection and downstream processing of data, can be significantly reduced compared to other naive approaches. The theoretical improvement provided by our algorithm over other naive approaches is dependent on several factors — task locations, network connectivity, processing times and available resources, and is bounded by the duration of the drone flight.
更多查看译文
关键词
edge computing,network-centric platform,cloud and edge resource provisioning,network management,workflow automation,Unpiloted Aerial Vehicle (UAV) systems,drone video analytics
AI 理解论文
溯源树
样例
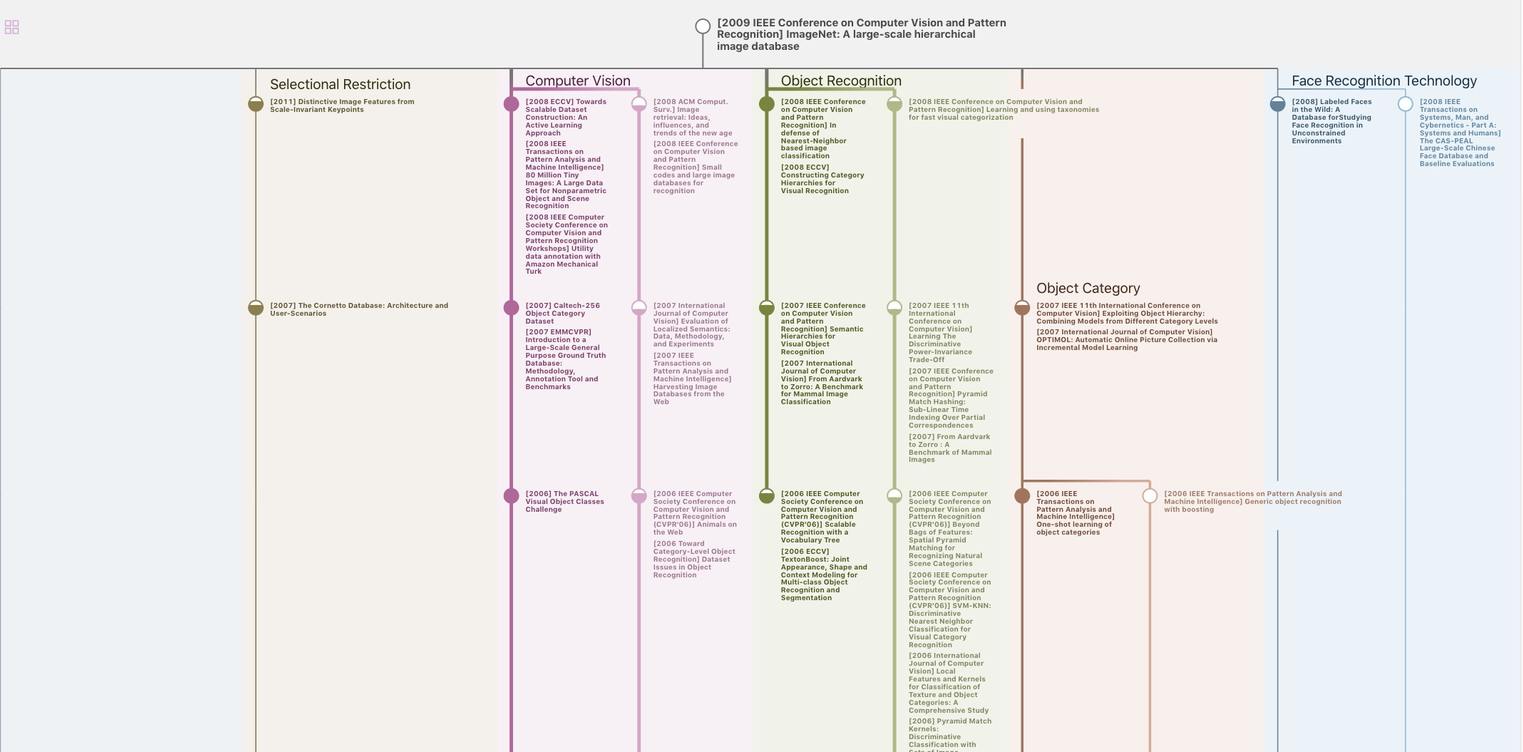
生成溯源树,研究论文发展脉络
Chat Paper
正在生成论文摘要