An Improved Version of MMOEA/DC Based on Alternative Clustering Definitions
2023 IEEE Congress on Evolutionary Computation (CEC)(2023)
摘要
Multimodal multiobjective optimization problems (MMOPs) have recently received considerable attention since they emerge in many real-world applications (e.g., in multiobjective knapsack problems and flow shop scheduling). However, MMOPs constitute a very particular class of problem. Indeed, looking for an adequate Pareto front (PF) representation is insufficient. MMOPs contain multiple subsets within the Pareto optimal Set, each independently mapping to the same Pareto Front. So, traditional multiobjective evolutionary algorithms (MOEAs) are inappropriate for solving MMOPs. This has motivated the design of algorithms which are suitable for addressing MMOPs. This paper proposes modifying MMOEA/DC, which is an algorithm specifically designed for solving MMOPs, that adopts a dual clustering method in both decision and objective space. Particularly, we were interested in using clustering in decision space, which allows classifying solutions into multiple local clusters. Our proposed approach modifies the neighborhood definition in order to provide more robustness to the algorithm as well as to improve the clustering process in decision space and to reduce the influence of the control parameters. The efficiency of the proposed framework is validated by comparing its performance on test instances of two test suites (MMF and MMMPO) with respect to the original MMOEA/DC and other state-of-the-art algorithms designed for solving MMOPs.
更多查看译文
关键词
Multimodal multi-objective optimization,Evolutionary computation,Clustering
AI 理解论文
溯源树
样例
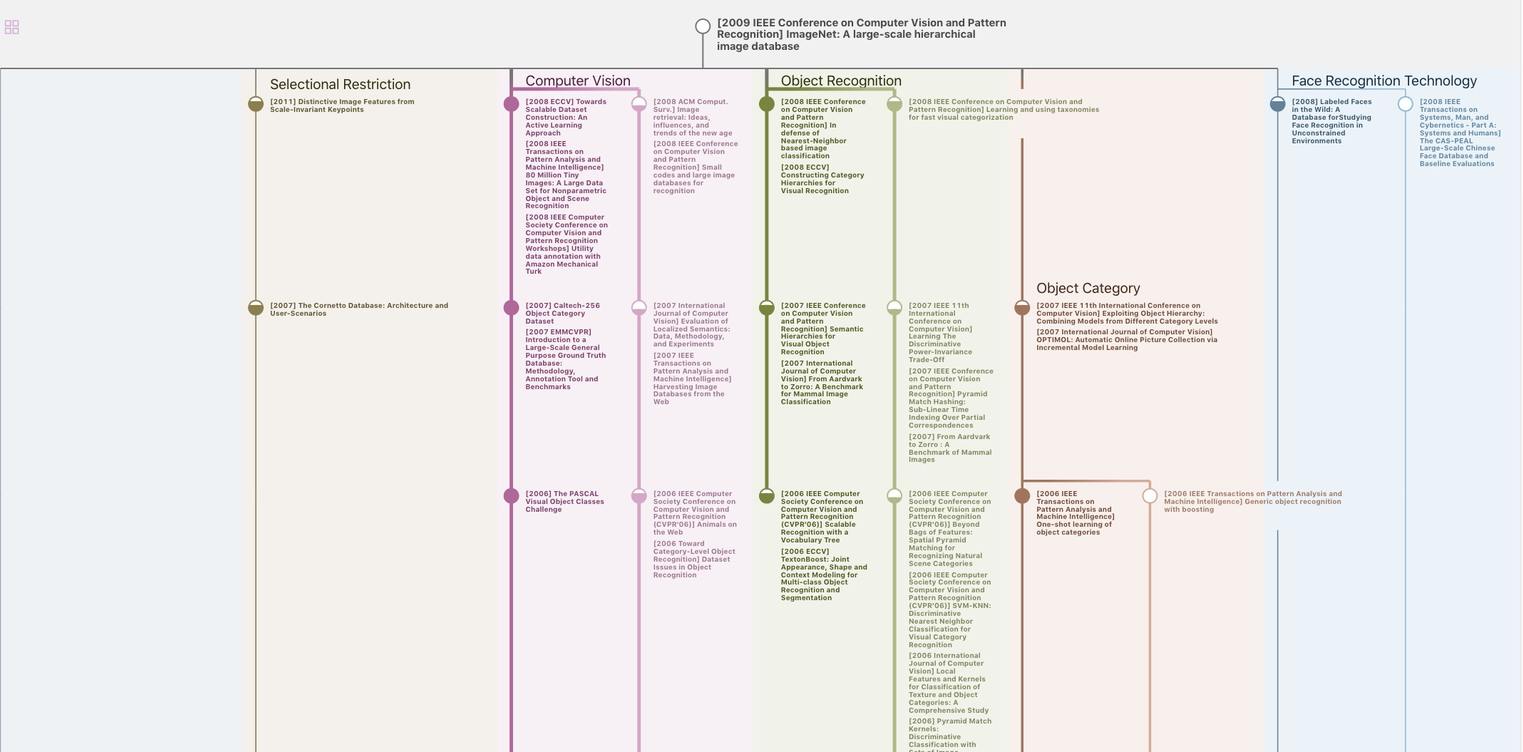
生成溯源树,研究论文发展脉络
Chat Paper
正在生成论文摘要