Solving the Discretization-based Feature Construction Problem using Bi-level Evolutionary Optimization
2023 IEEE Congress on Evolutionary Computation (CEC)(2023)
摘要
Feature construction represents a crucial data preprocessing technique in machine learning applications because it ensures the creation of new informative features from the original ones. This fact leads to the improvement of the classification performance and the reduction of the problem dimensionality. Since many feature construction methods require discrete data, it is important to perform discretization in order to transform the constructed features given in continuous values into their corresponding discrete versions. To deal with this situation, the aim of this paper is to jointly perform feature construction and feature discretization in a synchronous manner in order to benefit from the advantages of each process. Thus, we propose here to model the discretization-based feature construction task as a bi-level optimization problem in which the constructed features are evaluated based on their optimized sequence of cut-points. The resulting algorithm is termed Discretization-Based Feature Construction (Bi-DFC) where the proposed model is solved using an improved version of an existing co-evolutionary algorithm, named I-CEMBA that ensures the variation of concatenation trees. Bi-DFC performs the selection of original attributes at the upper level and ensures the creation and the evaluation of constructed features at the upper level based on their optimal corresponding sequence of cut-points. The obtained experimental results on ten high-dimensional datasets illustrate the ability of Bi-DFC in outperforming relevant state-of-the-art approaches in terms of classification results.
更多查看译文
关键词
Feature construction,data discretization,bi-level optimization,evolutionary algorithms
AI 理解论文
溯源树
样例
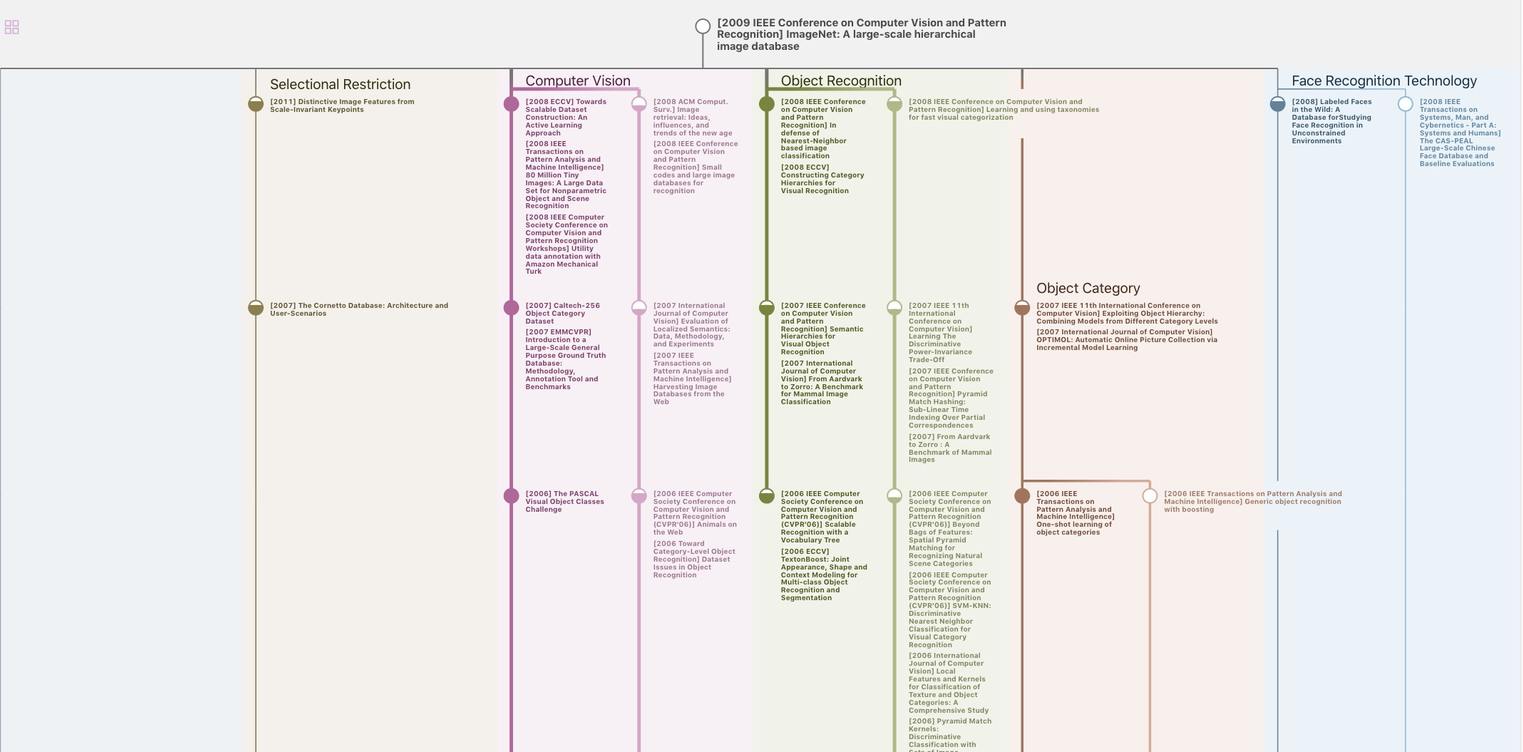
生成溯源树,研究论文发展脉络
Chat Paper
正在生成论文摘要