Toward Unbounded Search Space Exploration by Particle Swarm Optimization in Multi-Modal Optimization Problem
2023 IEEE Congress on Evolutionary Computation (CEC)(2023)
摘要
Since we cannot guarantee that the global optimal solution is included within a “pre-determined” search space in unknown problems such as real world problems, this paper addresses the “unbounded” search space exploration in swarm optimization. For this issue, this paper proposes the following three methods that dynamically extend the search area: Dynamic Search Area Exploration based on Gradient (DSAEG), which preferentially searches the area of a high gradient of solution space, Dynamic Search Area Exploration based on Novelty (DSAE-N), which preferentially searches the area where is far from the current search area, and Dynamic Search Area Exploration based on Gradient and Novelty (DSAE-GN), which integrates DSAE-G with DSAE-N. To investigate the effectiveness of the proposed three methods, this paper evaluates them in the six multi-modal optimization problems by applying them into Niching Migratory Multi-Swarm Optimiser (NMMSO) based on Particle Swarm Optimization (PSO). The intensive comparison of the proposed methods with NMMSO have been revealed the following implications: (1) DSAE-GN quickly finds almost of the global optimal solutions regardless of the shape of the evaluation function while DSAE-G and DSAE-N cannot always find them; and (2) DSAE-GN can find almost of the global optimal solutions even if the search area is not given, while NMMSO cannot find them even if the search area which contains all global optimal solutions is given.
更多查看译文
关键词
unbounded search space,multi-modal optimization,swarm optimization,niching migratory multi-swarm optimiser
AI 理解论文
溯源树
样例
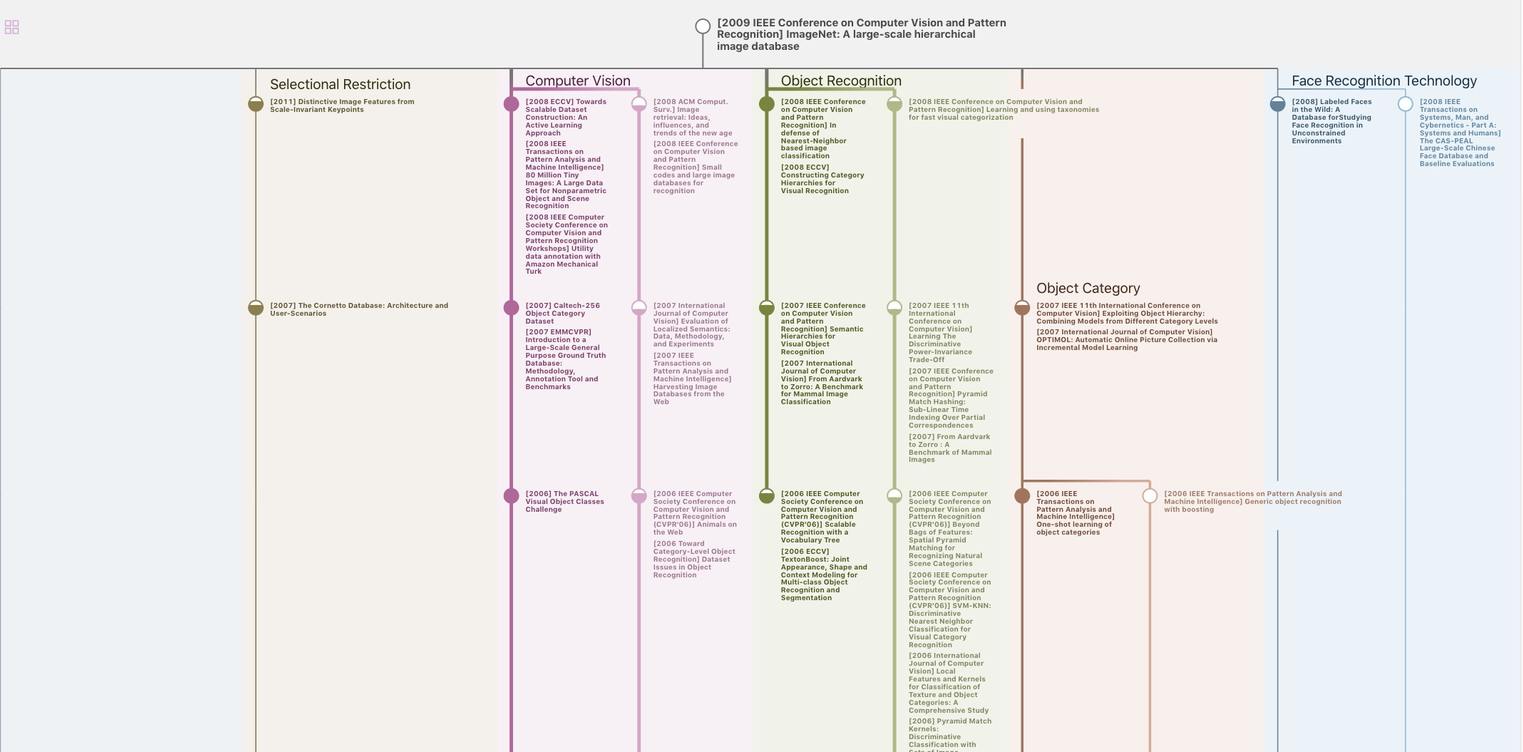
生成溯源树,研究论文发展脉络
Chat Paper
正在生成论文摘要