Micro Random Forest: A Local, High-Speed Implementation of a Machine-Learning Fault Location Method for Distribution Power Systems
2023 IEEE Power & Energy Society General Meeting (PESGM)(2023)
摘要
This work proposes and tests a Machine-Learning (ML), Traveling Wave-based fault location framework for distribution systems that only requires local voltage data. The feature creation stage is as follows: first, for a desired node, the 3-Phase measurements at a sampling frequency of 1 MHz are summarized into the ground mode using the Karrenbauer Transform. Second, the voltage ground mode is decomposed into 3 frequency bands using the Discrete Wavelet Transform. Third, the Parseval’s Theorem is employed to calculate the signals’ energy, which are later used to train Random Forest (RF) algorithms. The method is designed to run on a commercially-available Digital Signal Processing board. To adhere to the limited memory space and computing specifications of such a device, the processing is done in batches of 128 samples. The size of the ML models is reduced as well, which gives the name of “micro RF” to the method. Even observing these constraints, the micro RF method achieves an accuracy of more than 96% on the IEEE 34 nodes system, with a total execution time of 1.2 milliseconds.
更多查看译文
关键词
Power System Protection, Fault Location, Traveling Wave, Discrete Wavelet Transform, Random Forest
AI 理解论文
溯源树
样例
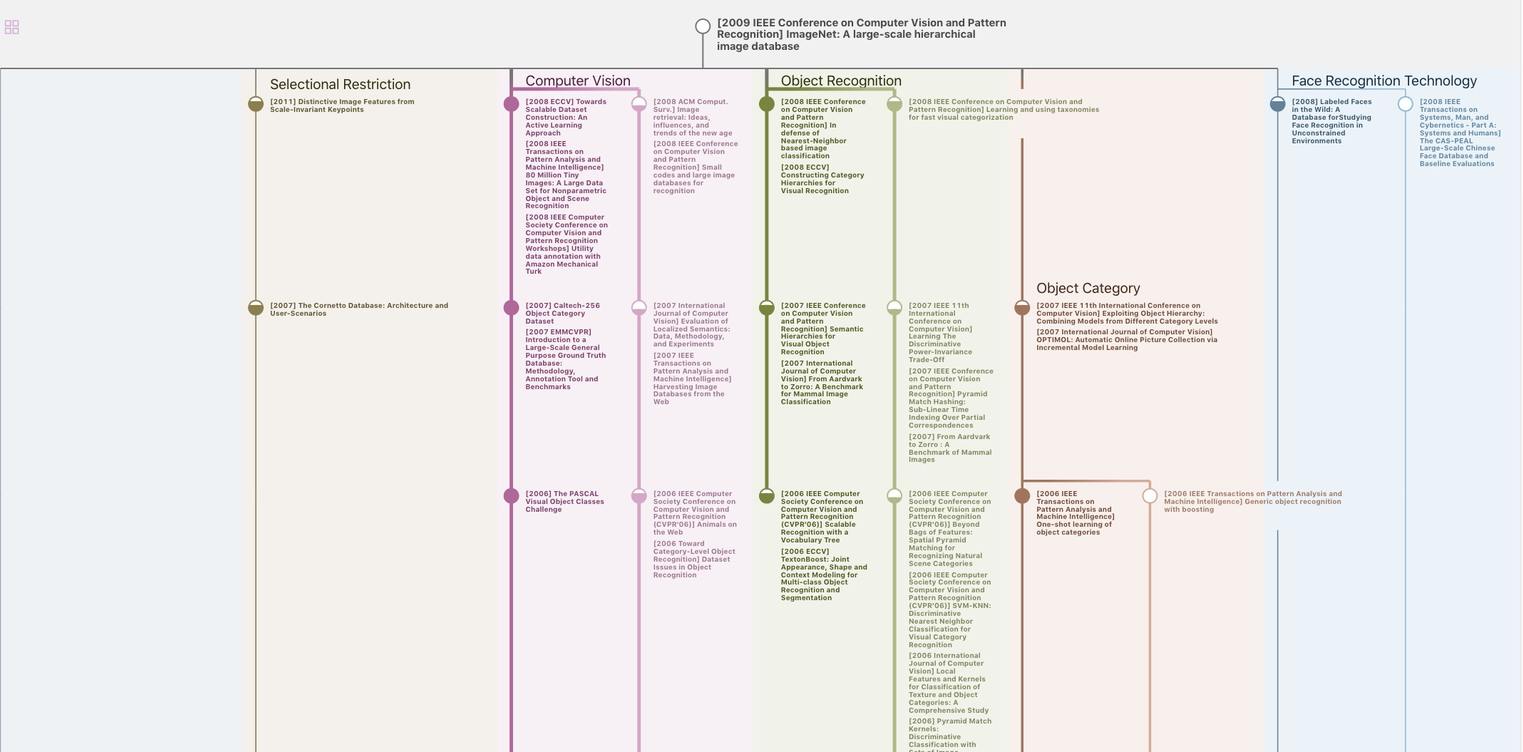
生成溯源树,研究论文发展脉络
Chat Paper
正在生成论文摘要