Generalized network-based dimensionality analysis
EXPERT SYSTEMS WITH APPLICATIONS(2024)
摘要
Network analysis opens new horizons for data analysis methods, as the results of ever-developing network science can be integrated into classical data analysis techniques. This paper presents the generalized version of network-based dimensionality reduction and analysis (NDA). The main contributions of this paper are as follows: (1) The proposed generalized dimensionality reduction and analysis (GNDA) method already handles low-dimensional high-sample-size (LDHSS) and high-dimensional and low-sample-size (HDLSS) at the same time. In addition, compared with existing methods, we show that only the proposed GNDA method adequately estimates the number of latent variables (LVs). (2) The proposed GNDA already considers any symmetric and nonsymmetric similarity functions between indicators (i.e., variables or observations) to specify LVs. (3) The proposed prefiltering and resolution parameters provide the hierarchical version of GNDA to check the robustness of LVs. The proposed GNDA method is compared with traditional dimensionality reduction methods on various simulated and real-world datasets.
更多查看译文
关键词
Dimensionality reduction,Nonparametric,Network science,Modularity,Similarity graphs
AI 理解论文
溯源树
样例
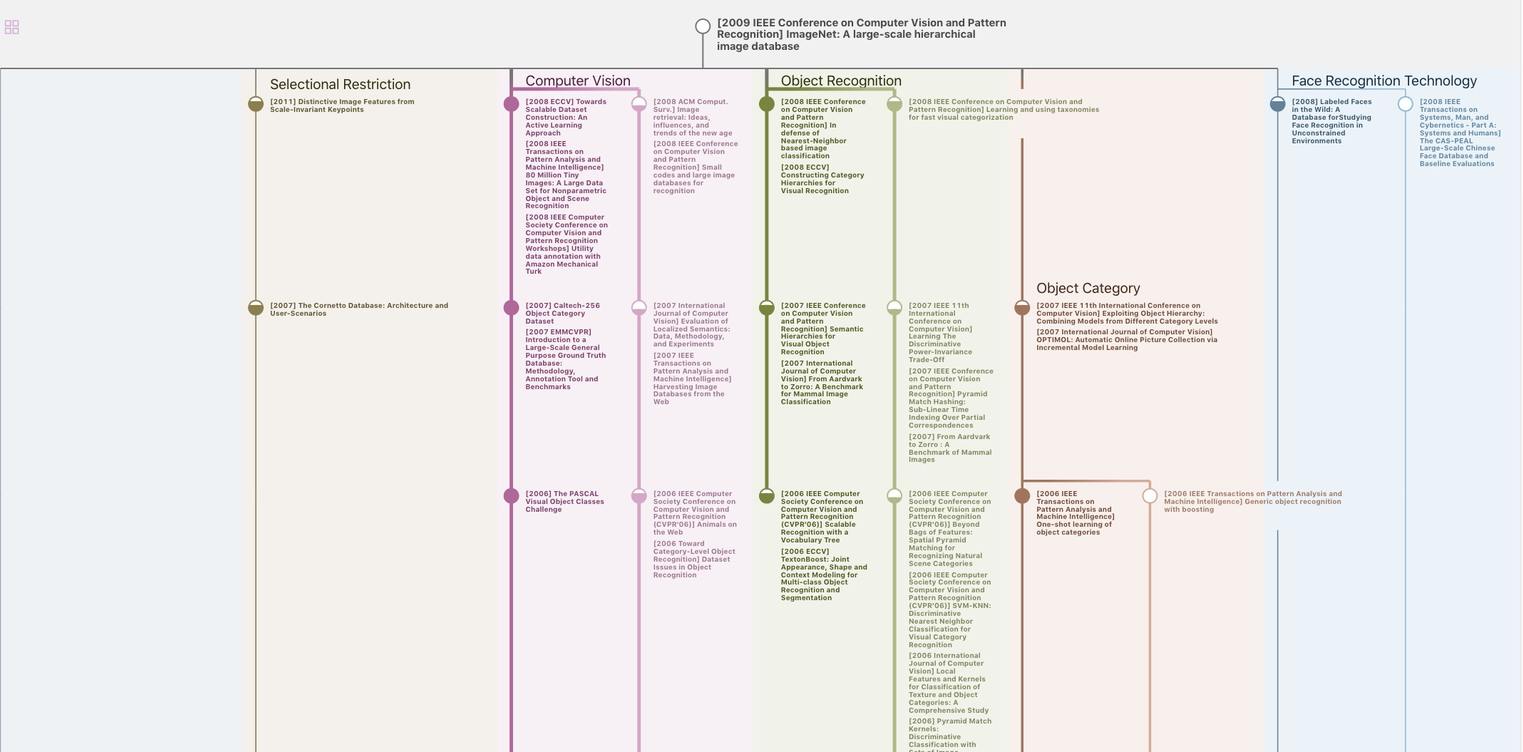
生成溯源树,研究论文发展脉络
Chat Paper
正在生成论文摘要