Combining prompt learning with contextual semantics for inductive relation prediction
EXPERT SYSTEMS WITH APPLICATIONS(2024)
摘要
Inductive relation prediction for knowledge graphs aims to predict missing relations between two new entities. Most previous studies on relation prediction are limited to the transductive setting and could not be applied to it. Recently, some inductive methods have been proposed to handle it by learning the topological semantics. However, they solely rely on structural information, disregarding the role of prior knowledge. In cases of sparse structures, this limitation is magnified, thereby hindering the inductive ability. Prior knowledge can not only filter out invalid topological structures but also complement the topological semantics. To this end, We propose a novel inductive model, PLCS, which incorporates prompt learning with contextual semantics to fully exploit prior knowledge. To filter out irrelevant topological structures, we innovatively employ hard prompts to mine prior knowledge in pre-trained language models (PLMs) as the basis for subgraph extraction. Additionally, we enhance semantic representation by integrating relation text descriptions into relation embeddings during initialization, supplementing topological semantics. The experimental results on four benchmark datasets show the superiority of PLCS over existing state-of-the-art methods.
更多查看译文
关键词
Knowledge graph,Inductive relation prediction,Prompt learning
AI 理解论文
溯源树
样例
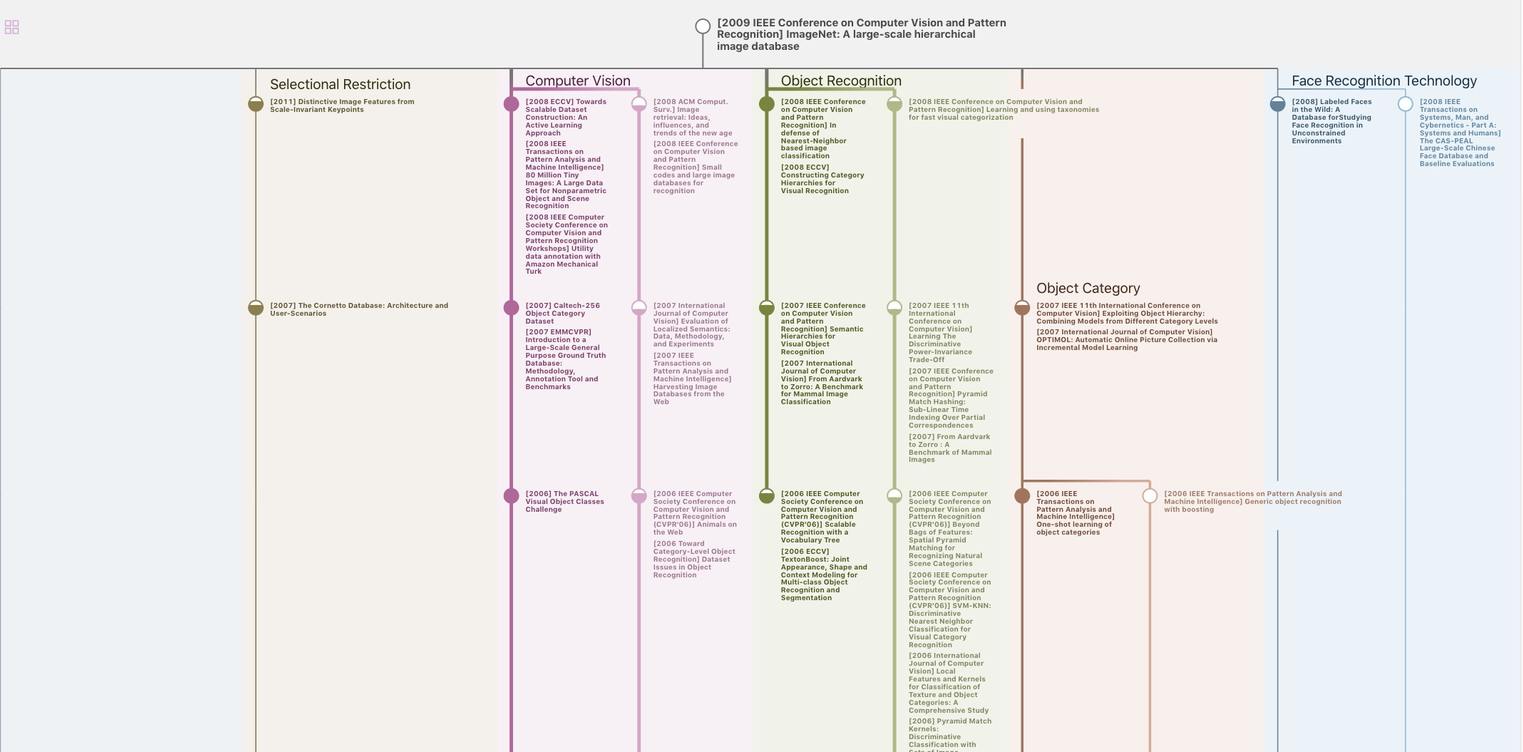
生成溯源树,研究论文发展脉络
Chat Paper
正在生成论文摘要