Connecting Speech Encoder and Large Language Model for ASR
ICASSP 2024 - 2024 IEEE International Conference on Acoustics, Speech and Signal Processing (ICASSP)(2023)
摘要
The impressive capability and versatility of large language models (LLMs) have aroused increasing attention in automatic speech recognition (ASR), with several pioneering studies attempting to build integrated ASR models by connecting a speech encoder with an LLM. This paper presents a comparative study of three commonly used structures as connectors, including fully connected layers, multi-head cross-attention, and Q-Former. Speech encoders from the Whisper model series as well as LLMs from the Vicuna model series with different model sizes were studied. Experiments were performed on the commonly used LibriSpeech, Common Voice, and GigaSpeech datasets, where the LLMs with Q-Formers demonstrated consistent and considerable word error rate (WER) reductions over LLMs with other connector structures. Q-Former-based LLMs can generalise well to out-of-domain datasets, where 12% relative WER reductions over the Whisper baseline ASR model were achieved on the Eval2000 test set without using any in-domain training data from Switchboard. Moreover, a novel segment-level Q-Former is proposed to enable LLMs to recognise speech segments with a duration exceeding the limitation of the encoders, which results in 17% relative WER reductions over other connector structures on 90-second-long speech data.
更多查看译文
关键词
Large language model,automatic speech recognition,Q-Former,long-form speech
AI 理解论文
溯源树
样例
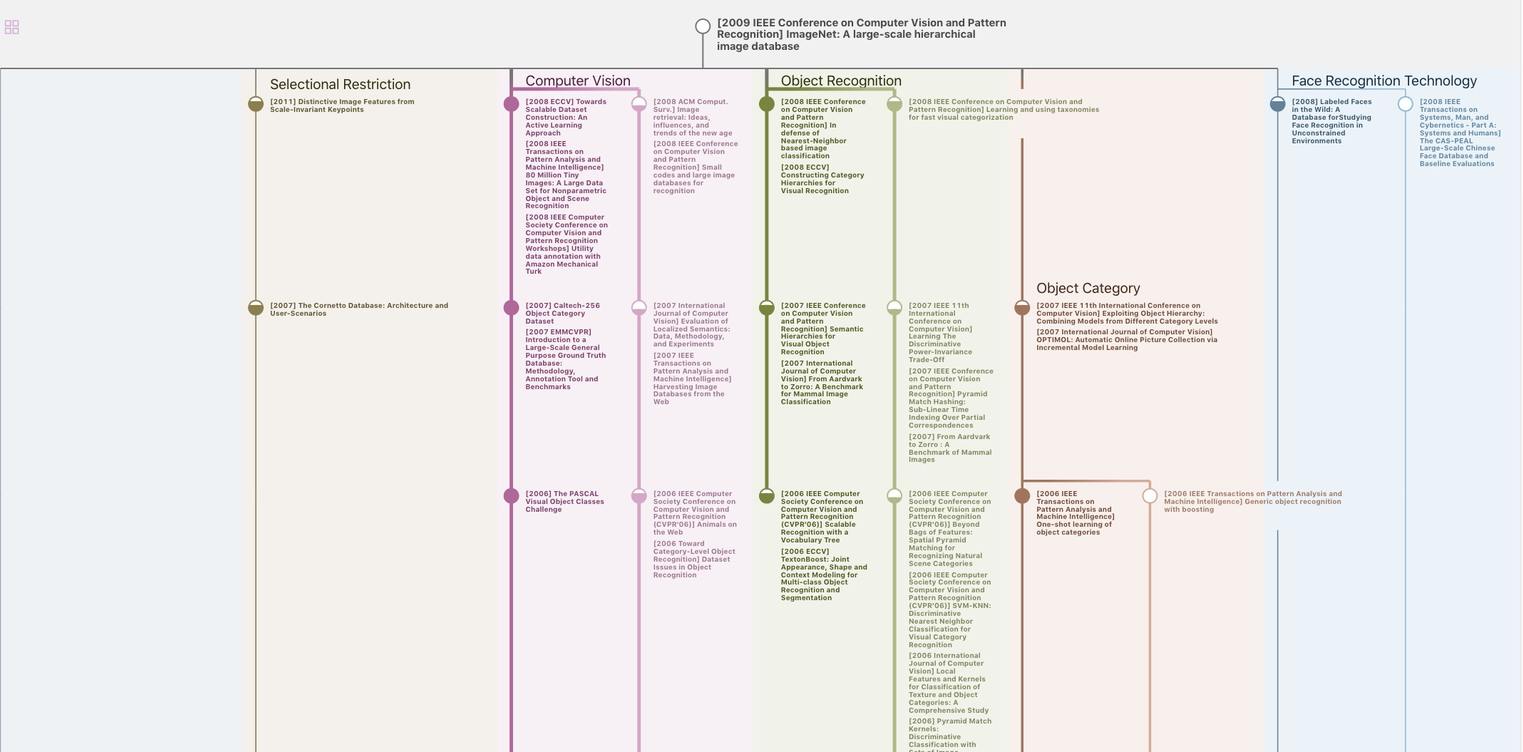
生成溯源树,研究论文发展脉络
Chat Paper
正在生成论文摘要