In-Domain GAN Inversion for Faithful Reconstruction and Editability.
CoRR(2024)
摘要
Generative Adversarial Networks (GANs) have significantly advanced image synthesis through mapping randomly sampled latent codes to high-fidelity synthesized images. However, applying well-trained GANs to real image editing remains challenging. A common solution is to find an approximate latent code that can adequately recover the input image to edit, which is also known as GAN inversion. To invert a GAN model, prior works typically focus on reconstructing the target image at the pixel level, yet few studies are conducted on whether the inverted result can well support manipulation at the semantic level. This work fills in this gap by proposing in-domain GAN inversion, which consists of a domain-guided encoder and a domain-regularized optimizer, to regularize the inverted code in the native latent space of the pre-trained GAN model. In this way, we manage to sufficiently reuse the knowledge learned by GANs for image reconstruction, facilitating a wide range of editing applications without any retraining. We further make comprehensive analyses on the effects of the encoder structure, the starting inversion point, as well as the inversion parameter space, and observe the trade-off between the reconstruction quality and the editing property. Such a trade-off sheds light on how a GAN model represents an image with various semantics encoded in the learned latent distribution. Code, models, and demo are available at the project page https://genforce.github.io/idinvert/.
更多查看译文
关键词
Generative adversarial network,GAN inversion,image editing
AI 理解论文
溯源树
样例
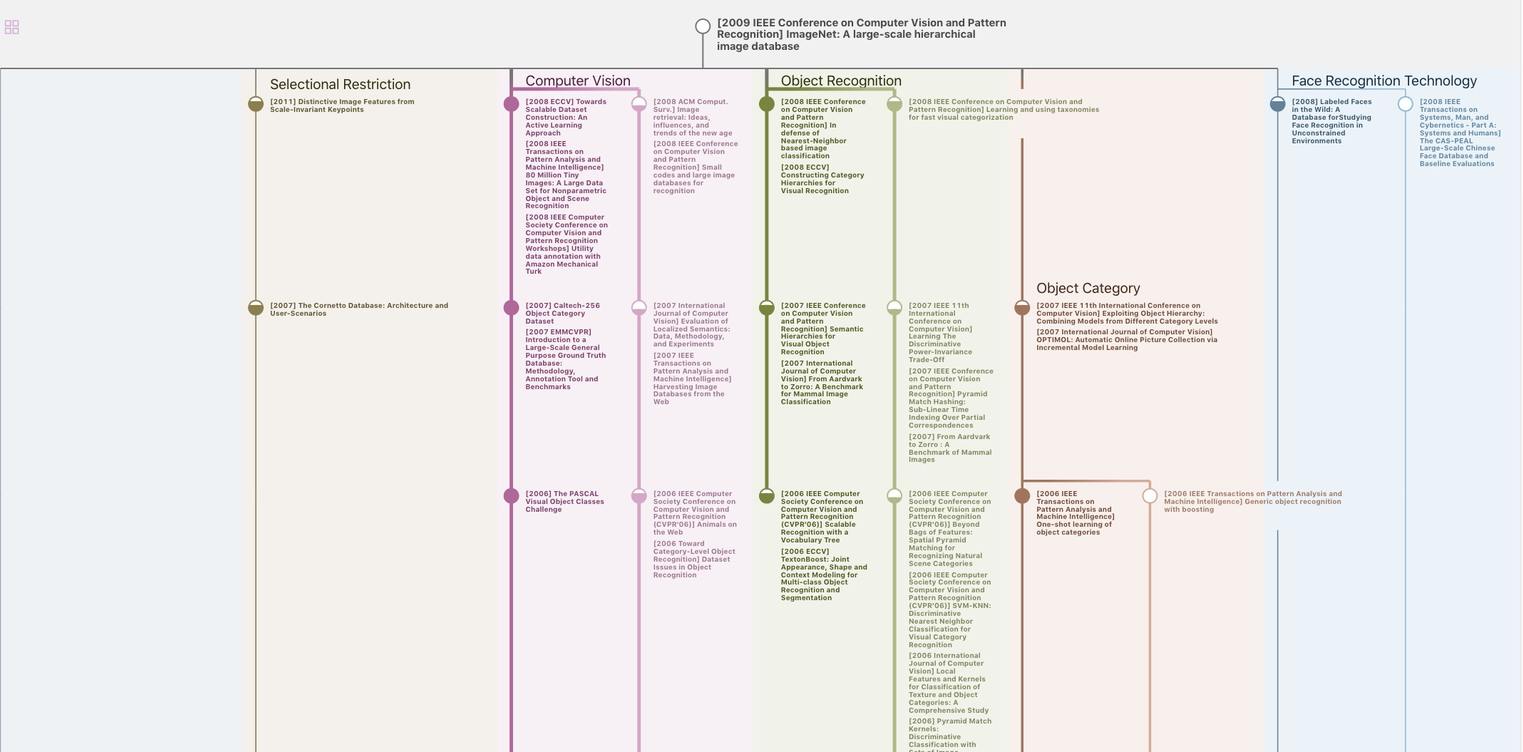
生成溯源树,研究论文发展脉络
Chat Paper
正在生成论文摘要