A comparison of controller architectures and learning mechanisms for arbitrary robot morphologies
CoRR(2023)
摘要
The main question this paper addresses is: What combination of a robot controller and a learning method should be used, if the morphology of the learning robot is not known in advance? Our interest is rooted in the context of morphologically evolving modular robots, but the question is also relevant in general, for system designers interested in widely applicable solutions. We perform an experimental comparison of three controller-and-learner combinations: one approach where controllers are based on modelling animal locomotion (Central Pattern Generators, CPG) and the learner is an evolutionary algorithm, a completely different method using Reinforcement Learning (RL) with a neural network controller architecture, and a combination `in-between' where controllers are neural networks and the learner is an evolutionary algorithm. We apply these three combinations to a test suite of modular robots and compare their efficacy, efficiency, and robustness. Surprisingly, the usual CPG-based and RL-based options are outperformed by the in-between combination that is more robust and efficient than the other two setups.
更多查看译文
关键词
evolutionary robotics,Reinforcement learning,controller,learning algorithm,CPG
AI 理解论文
溯源树
样例
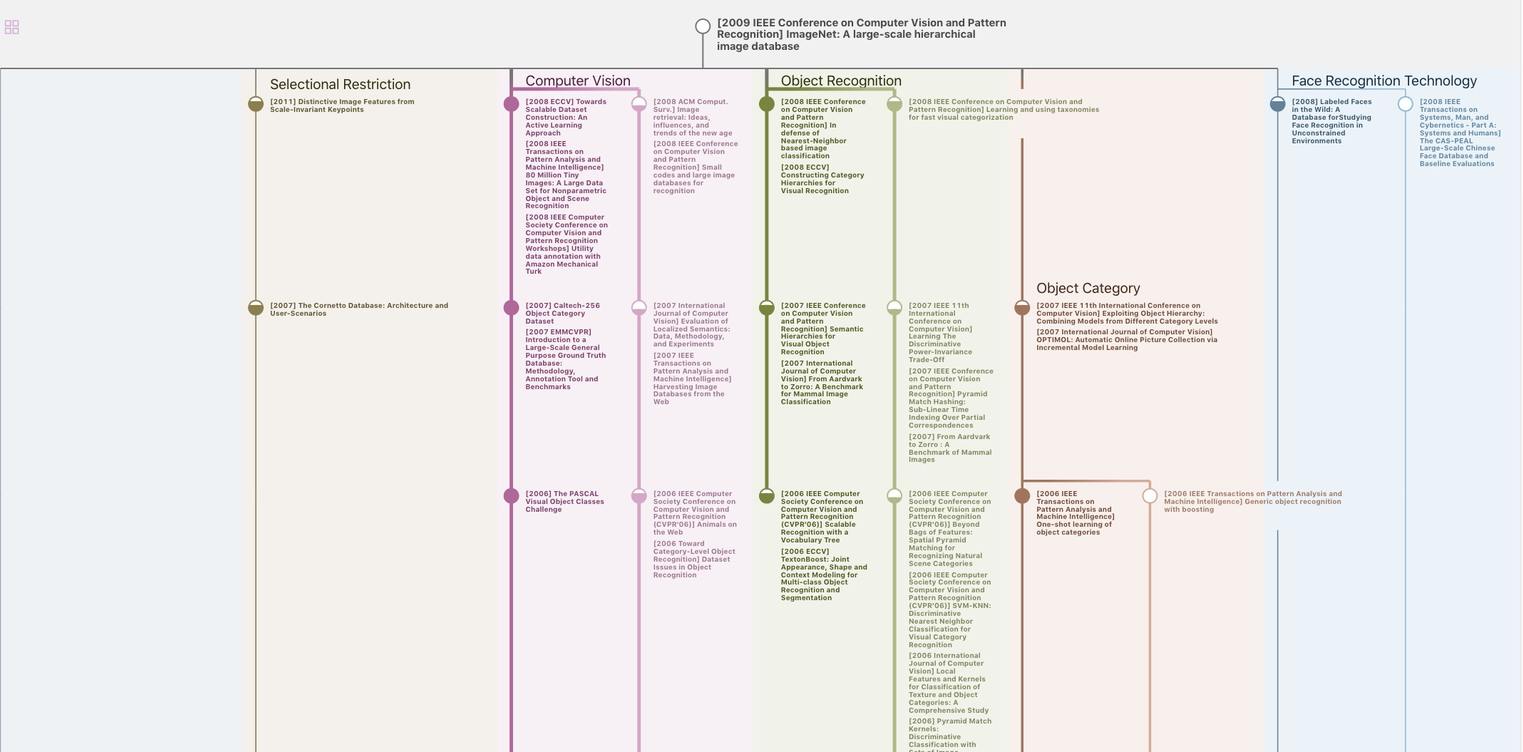
生成溯源树,研究论文发展脉络
Chat Paper
正在生成论文摘要