Attention and Pooling based Sigmoid Colon Segmentation in 3D CT images
CoRR(2023)
摘要
Segmentation of the sigmoid colon is a crucial aspect of treating diverticulitis. It enables accurate identification and localisation of inflammation, which in turn helps healthcare professionals make informed decisions about the most appropriate treatment options. This research presents a novel deep learning architecture for segmenting the sigmoid colon from Computed Tomography (CT) images using a modified 3D U-Net architecture. Several variations of the 3D U-Net model with modified hyper-parameters were examined in this study. Pyramid pooling (PyP) and channel-spatial Squeeze and Excitation (csSE) were also used to improve the model performance. The networks were trained using manually annotated sigmoid colon. A five-fold cross-validation procedure was used on a test dataset to evaluate the network's performance. As indicated by the maximum Dice similarity coefficient (DSC) of 56.92+/-1.42%, the application of PyP and csSE techniques improves segmentation precision. We explored ensemble methods including averaging, weighted averaging, majority voting, and max ensemble. The results show that average and majority voting approaches with a threshold value of 0.5 and consistent weight distribution among the top three models produced comparable and optimal results with DSC of 88.11+/-3.52%. The results indicate that the application of a modified 3D U-Net architecture is effective for segmenting the sigmoid colon in Computed Tomography (CT) images. In addition, the study highlights the potential benefits of integrating ensemble methods to improve segmentation precision.
更多查看译文
关键词
Image segmentation,deep learning,sigmoid colon segmentation,Computed Tomography,diverticulitis
AI 理解论文
溯源树
样例
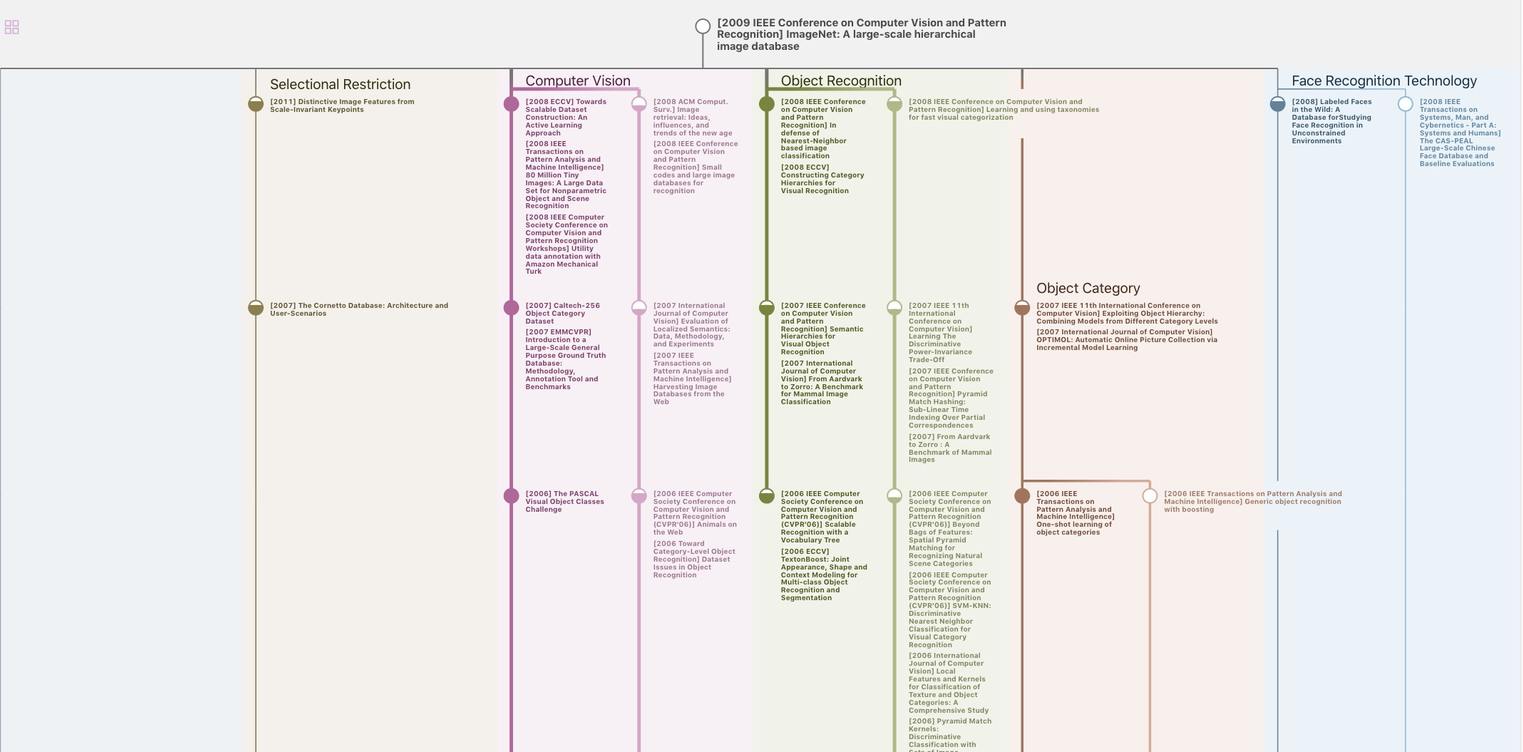
生成溯源树,研究论文发展脉络
Chat Paper
正在生成论文摘要