Arabic Sentiment Analysis with Noisy Deep Explainable Model
PROCEEDINGS OF 2023 7TH INTERNATIONAL CONFERENCE ON NATURAL LANGUAGE PROCESSING AND INFORMATION RETRIEVAL, NLPIR 2023(2023)
摘要
Sentiment Analysis (SA) is an essential task for numerous real-world applications. However, the majority of SA research focuses on high-resource languages such as English and Chinese, while limited-resource languages like Arabic and Bengali receive less attention. Additionally, existing Arabic sentiment analysis methods based on advanced artificial intelligence (AI) approaches tend to operate as black boxes, making it challenging to comprehend the reasoning behind their predictions. This paper proposes an explainable sentiment classification framework for the Arabic language. We introduce a noise layer to different deep learning (DL) models, including BiLSTM and CNN-BiLSTM, to address the issue of overfitting. The proposed framework enables the explanation of specific predictions by training a local surrogate explainable model, shedding light on the reasons behind each sentiment prediction (positive or negative). Experiments were conducted on publicly available benchmark Arabic SA datasets, and the results demonstrated that the inclusion of noise layers in the DL model improves performance for the Arabic language by mitigating overfitting. Our method also outperformed several state-of-the-art approaches. Moreover, the introduction of explainability with the noise layer enhances transparency and accountability, making the model suitable for practical adoption in AI-enabled systems.
更多查看译文
关键词
Arabic Sentiment Analysis,Noise layer,BiLSTM,CNN,Explainable AI (XAI),Interpretability
AI 理解论文
溯源树
样例
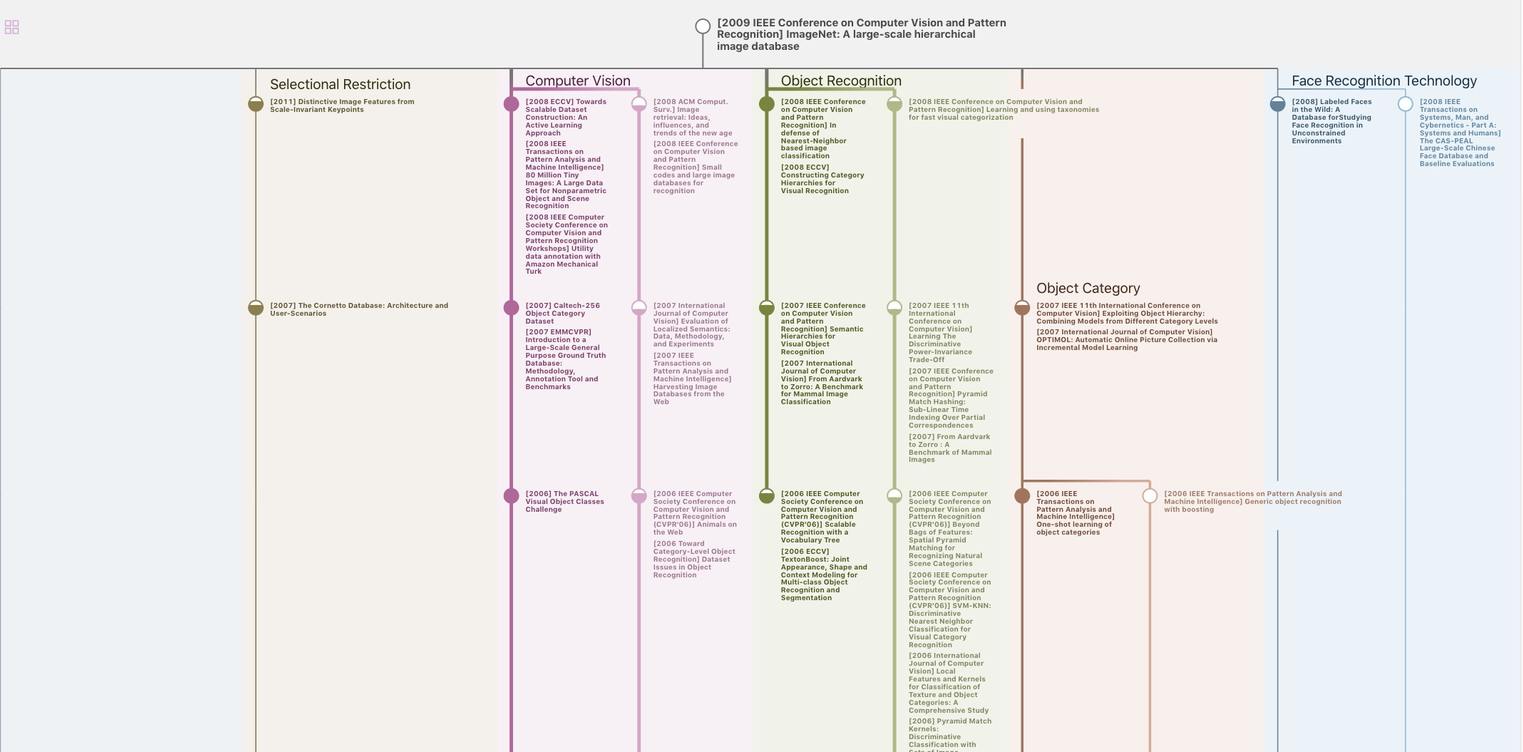
生成溯源树,研究论文发展脉络
Chat Paper
正在生成论文摘要