Tight bounds on Pauli channel learning without entanglement
Physical Review Letters(2023)
摘要
Entanglement is a useful resource for learning, but a precise characterization of its advantage can be challenging. In this work, we consider learning algorithms without entanglement to be those that only utilize separable states, measurements, and operations between the main system of interest and an ancillary system. These algorithms are equivalent to those that apply quantum circuits on the main system interleaved with mid-circuit measurements and classical feedforward. We prove a tight lower bound for learning Pauli channels without entanglement that closes a cubic gap between the best-known upper and lower bound. In particular, we show that $\Theta(2^n\varepsilon^{-2})$ rounds of measurements are required to estimate each eigenvalue of an $n$-qubit Pauli channel to $\varepsilon$ error with high probability when learning without entanglement. In contrast, a learning algorithm with entanglement only needs $\Theta(\varepsilon^{-2})$ rounds of measurements. The tight lower bound strengthens the foundation for an experimental demonstration of entanglement-enhanced advantages for characterizing Pauli noise.
更多查看译文
AI 理解论文
溯源树
样例
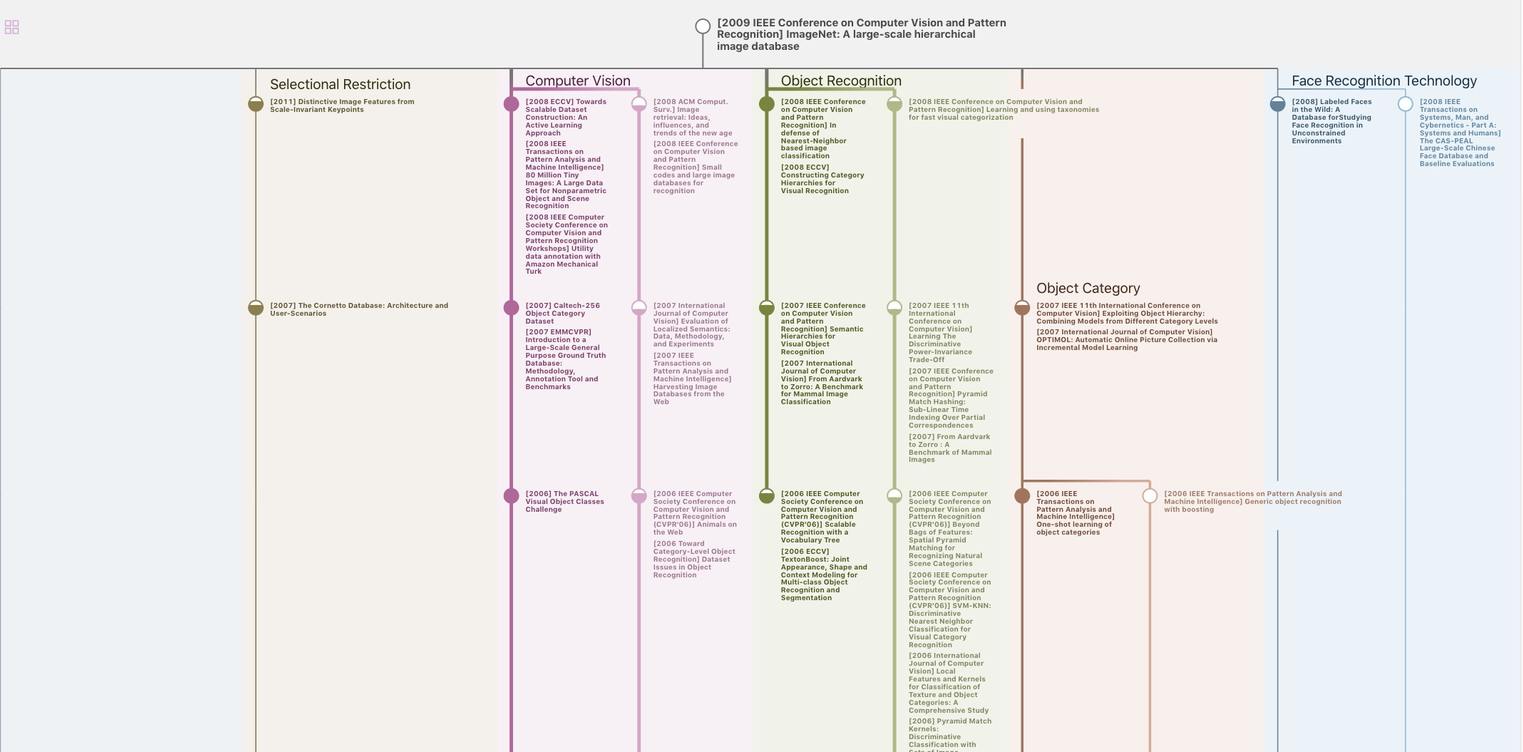
生成溯源树,研究论文发展脉络
Chat Paper
正在生成论文摘要