Causal Reasoning: Charting a Revolutionary Course for Next-Generation AI-Native Wireless Networks
IEEE VEHICULAR TECHNOLOGY MAGAZINE(2024)
摘要
Despite the basic premise that next-generation wireless networks (e.g., 6G) will be artificial intelligence (AI)-native, to date, most existing efforts remain either qualitative or incremental extensions to existing "AI for wireless" paradigms. Indeed, creating AI-native wireless networks faces significant technical challenges due to the limitations of data-driven, training-intensive AI. These limitations include the black-box nature of the AI models; their curve-fitting nature, which can limit their ability to reason and adapt; their reliance on large amounts of training data; and the energy inefficiency of large neural networks (NNs). In response to these limitations, this article presents a comprehensive, forward-looking vision that addresses these shortcomings by introducing a novel framework for building AI-native wireless networks, grounded in the emerging field of causal reasoning. Causal reasoning, founded on causal discovery, causal representation learning (CRL), and causal inference, can help build explainable, reasoning-aware, and sustainable wireless networks. Embracing such a human-like AI foundation can revolutionize the design of AI-native wireless networks, laying the foundations for creating self-sustaining networks that ensure uninterrupted connectivity. Toward fulfilling this vision, we first highlight several wireless networking challenges that can be addressed by causal discovery and representation, including ultrareliable beamforming for terahertz (THz) systems, near-accurate physical twin modeling for digital twins (DTs), training data augmentation, and semantic communication (SC). We showcase how incorporating causal discovery can assist in achieving dynamic adaptability, resilience, and cognition in addressing these challenges. Furthermore, we outline potential frameworks that leverage causal inference to achieve the overarching objectives of future-generation networks, including intent management, dynamic adaptability, human-level cognition, reasoning, and the critical element of time sensitivity. We conclude by offering recommendations shaping the roadmap toward causality-driven AI-native next-generation wireless networking.
更多查看译文
关键词
Cognition,Artificial intelligence,Wireless networks,Wireless sensor networks,Adaptation models,Data models,Vehicle dynamics
AI 理解论文
溯源树
样例
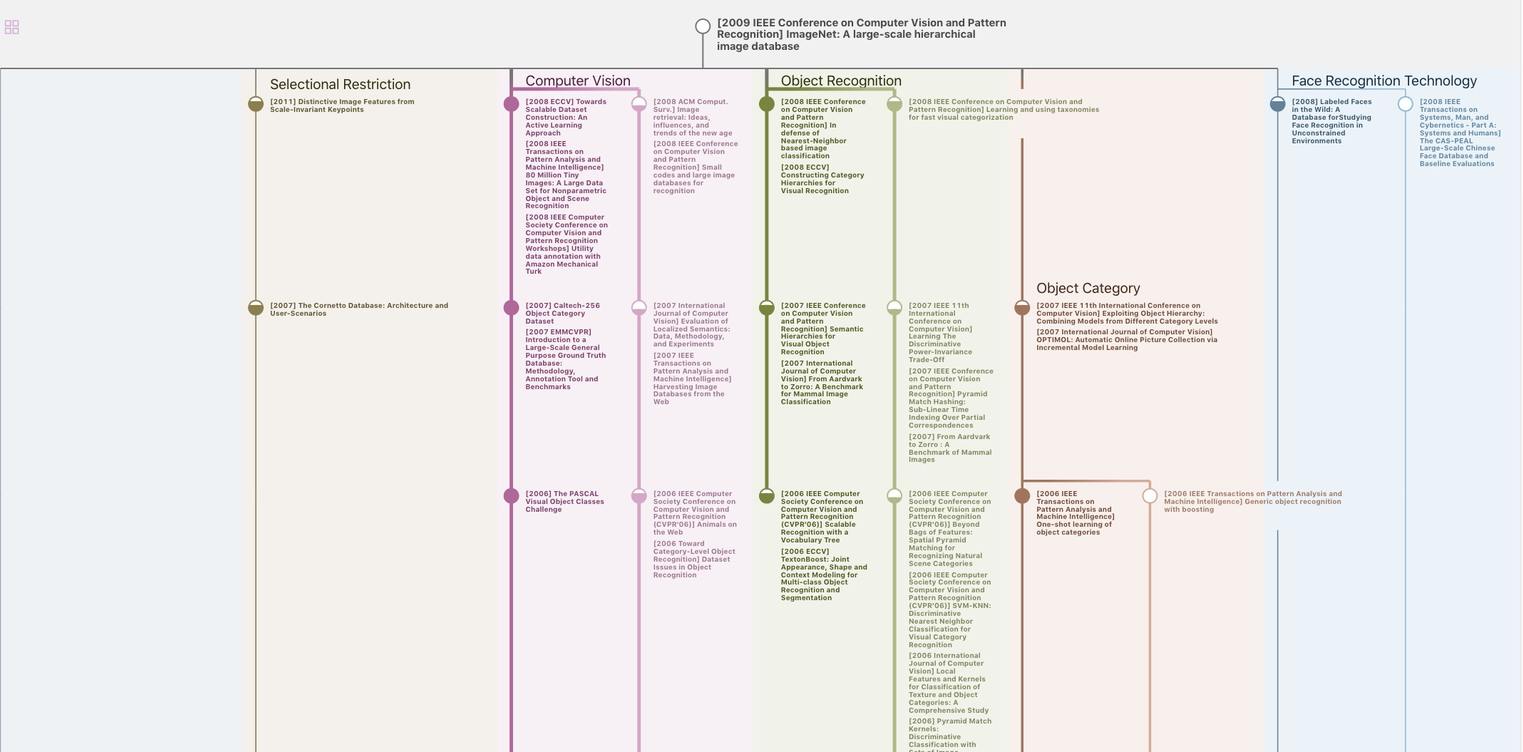
生成溯源树,研究论文发展脉络
Chat Paper
正在生成论文摘要