A New Optimization Idea: Parallel Search-Based Golden Jackal Algorithm
IEEE Access(2023)
摘要
As the variety and scope of optimization problems continue to expand, the applicability of a single algorithm may not be universal. To address this challenge, this paper introduces an enhanced iteration of the Golden Jackal Optimization (GJO) algorithm, termed the Parallel Search-based Golden Jackal Optimization (PGJO) algorithm. This algorithm integrates the concept of parallel search into the initialization, updating, and selection mechanisms. It includes a chaotic mapping preselection during the initialization phase, employs a cloning-like strategy for population updates, and incorporates an advanced simulated annealing approach during the selection phase. Through a comprehensive comparison of PGJO with various intelligent optimization algorithms across diverse benchmark functions and real-world scenarios, we affirm its effectiveness in terms of enhanced convergence accuracy and reduced required iterations.
更多查看译文
关键词
Optimization, Statistics, Sociology, Behavioral sciences, Search problems, Particle swarm optimization, Approximation algorithms, Globalization, Golden jackal optimization, parallel search, global search, initialization mechanism
AI 理解论文
溯源树
样例
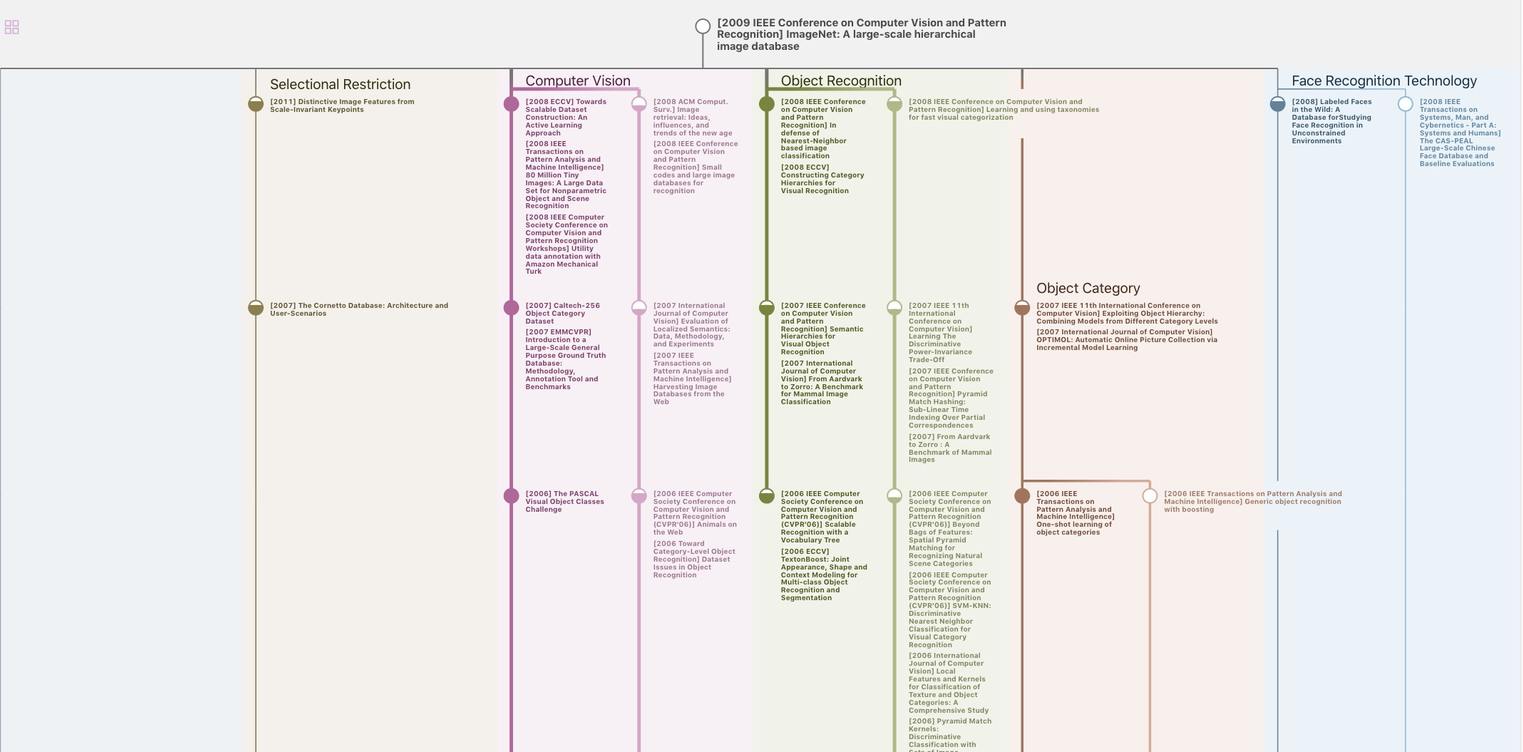
生成溯源树,研究论文发展脉络
Chat Paper
正在生成论文摘要