Detect Everything with Few Examples
arXiv (Cornell University)(2023)
摘要
Few-shot object detection aims at detecting novel categories given a few
example images. Recent methods focus on finetuning strategies, with complicated
procedures that prohibit a wider application. In this paper, we introduce
DE-ViT, a few-shot object detector without the need for finetuning. DE-ViT's
novel architecture is based on a new region-propagation mechanism for
localization. The propagated region masks are transformed into bounding boxes
through a learnable spatial integral layer. Instead of training prototype
classifiers, we propose to use prototypes to project ViT features into a
subspace that is robust to overfitting on base classes. We evaluate DE-ViT on
few-shot, and one-shot object detection benchmarks with Pascal VOC, COCO, and
LVIS. DE-ViT establishes new state-of-the-art results on all benchmarks.
Notably, for COCO, DE-ViT surpasses the few-shot SoTA by 15 mAP on 10-shot and
7.2 mAP on 30-shot and one-shot SoTA by 2.8 AP50. For LVIS, DE-ViT outperforms
few-shot SoTA by 20 box APr.
更多查看译文
关键词
few examples
AI 理解论文
溯源树
样例
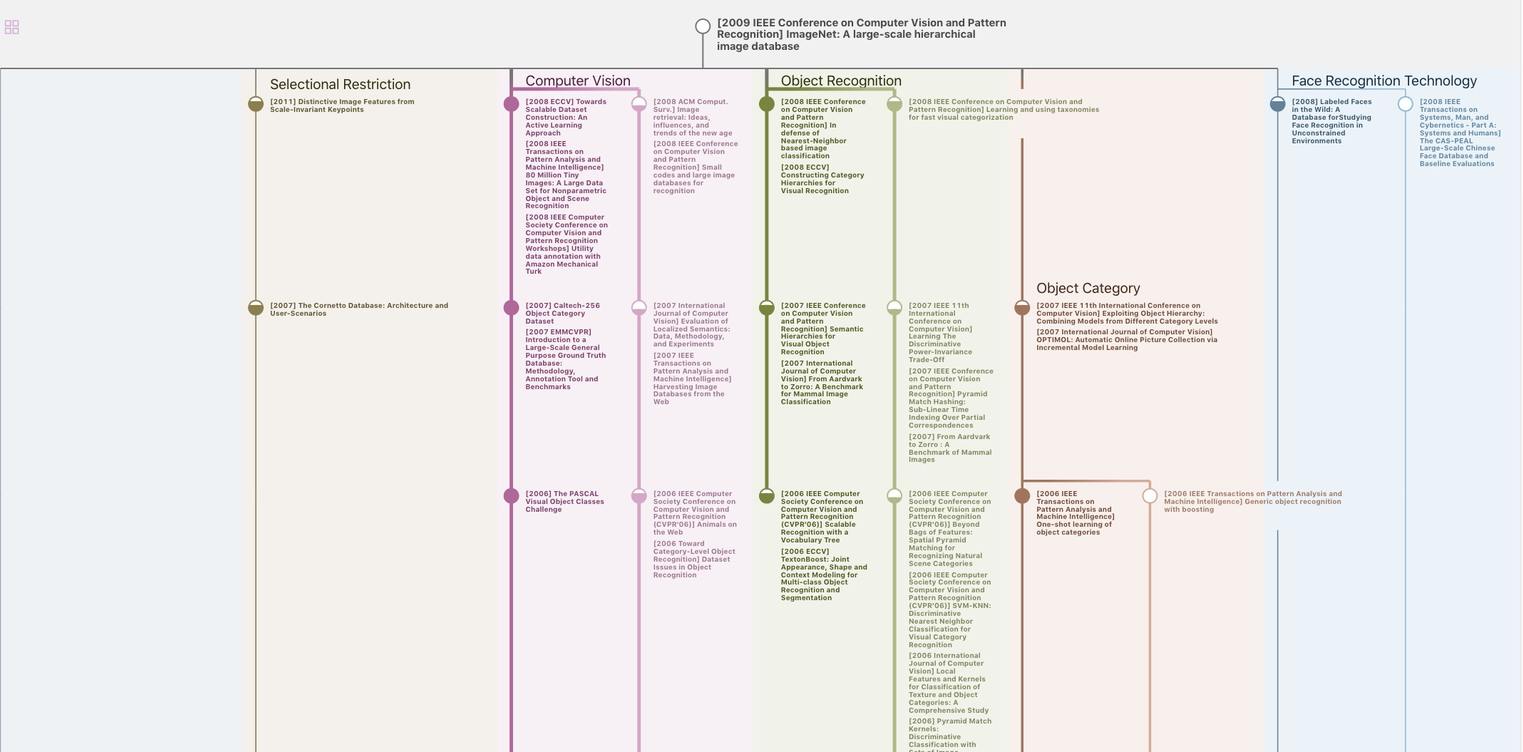
生成溯源树,研究论文发展脉络
Chat Paper
正在生成论文摘要