Diffusion Augmentation for Sequential Recommendation
PROCEEDINGS OF THE 32ND ACM INTERNATIONAL CONFERENCE ON INFORMATION AND KNOWLEDGE MANAGEMENT, CIKM 2023(2023)
摘要
Sequential recommendation (SRS) has become the technical foundation in many applications recently, which aims to recommend the next item based on the user's historical interactions. However, sequential recommendation often faces the problem of data sparsity, which widely exists in recommender systems. Besides, most users only interact with a few items, but existing SRS models often underperform these users. Such a problem, named the long-tail user problem, is still to be resolved. Data augmentation is a distinct way to alleviate these two problems, but they often need fabricated training strategies or are hindered by poor-quality generated interactions. To address these problems, we propose a Diffusion Augmentation for Sequential Recommendation (DiffuASR) for a higher quality generation. The augmented dataset by DiffuASR can be used to train the sequential recommendation models directly, free from complex training procedures. To make the best of the generation ability of the diffusion model, we first propose a diffusion-based pseudo sequence generation framework to fill the gap between image and sequence generation. Then, a sequential U-Net is designed to adapt the diffusion noise prediction model U-Net to the discrete sequence generation task. At last, we develop two guide strategies to assimilate the preference between generated and origin sequences. To validate the proposed DiffuASR, we conduct extensive experiments on three real-world datasets with three sequential recommendation models. The experimental results illustrate the effectiveness of DiffuASR. As far as we know, DiffuASR is one pioneer that introduce the diffusion model to the recommendation. The implementation code is available online (12).
更多查看译文
关键词
Sequential Recommendation,Data Augmentation,Diffusion Model
AI 理解论文
溯源树
样例
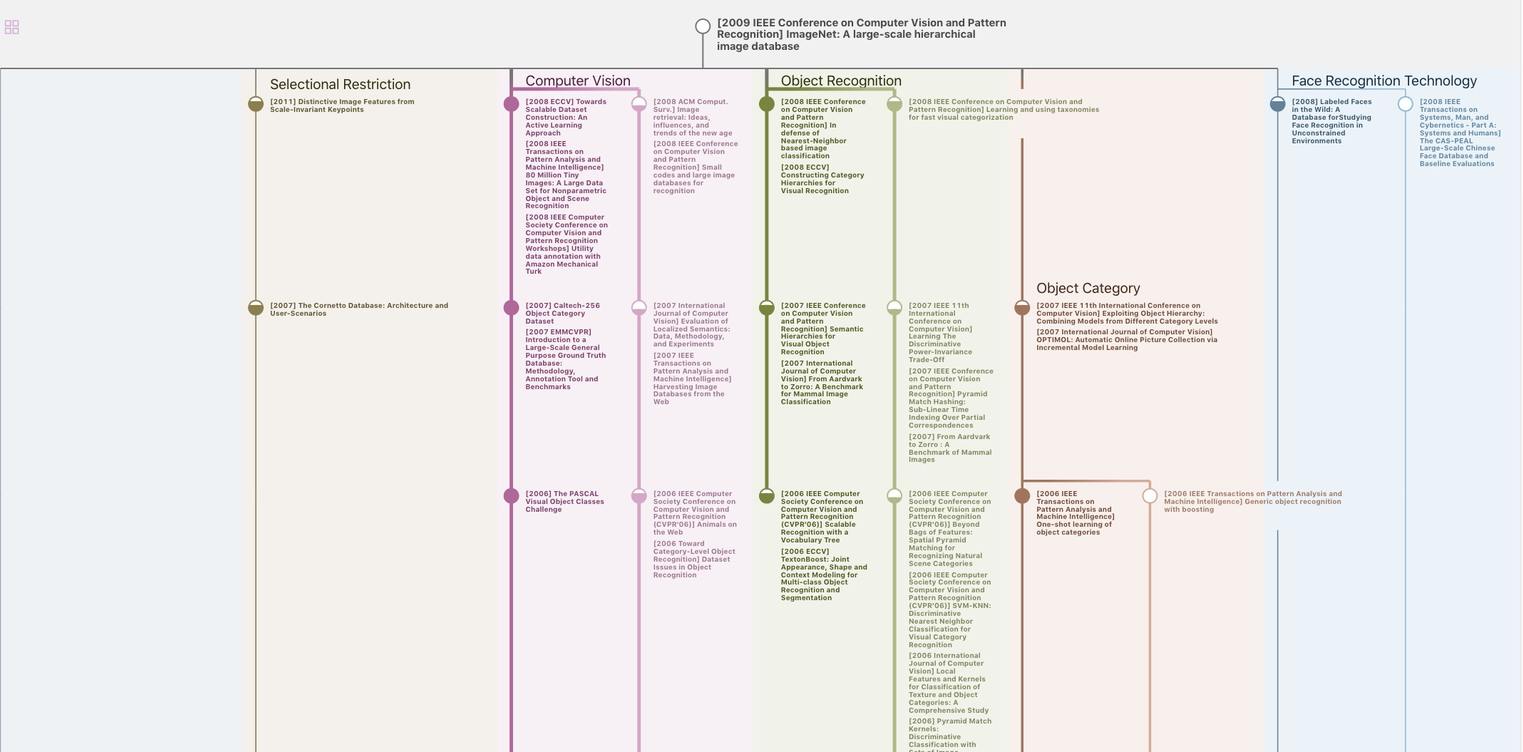
生成溯源树,研究论文发展脉络
Chat Paper
正在生成论文摘要