Synthetic Boost: Leveraging Synthetic Data for Enhanced Vision-Language Segmentation in Echocardiography
SIMPLIFYING MEDICAL ULTRASOUND, ASMUS 2023(2023)
摘要
Accurate segmentation is essential for echocardiography-based assessment of cardiovascular diseases (CVDs). However, the variability among sonographers and the inherent challenges of ultrasound images hinder precise segmentation. By leveraging the joint representation of image and text modalities, Vision-Language Segmentation Models (VLSMs) can incorporate rich contextual information, potentially aiding in accurate and explainable segmentation. However, the lack of readily available data in echocardiography hampers the training of VLSMs. In this study, we explore using synthetic datasets from Semantic Diffusion Models (SDMs) to enhance VLSMs for echocardiography segmentation. We evaluate results for two popular VLSMs (CLIPSeg and CRIS) using seven different kinds of language prompts derived from several attributes, automatically extracted from echocardiography images, segmentation masks, and their metadata. Our results show improved metrics and faster convergence when pretraining VLSMs on SDM-generated synthetic images before finetuning on real images. The code, configs, and prompts are available at https://github.com/naamiinepal/synthetic-boost.
更多查看译文
关键词
Vision-Language Models,Vision-Language Segmentation Models,Echocardiography,Synthetic Data
AI 理解论文
溯源树
样例
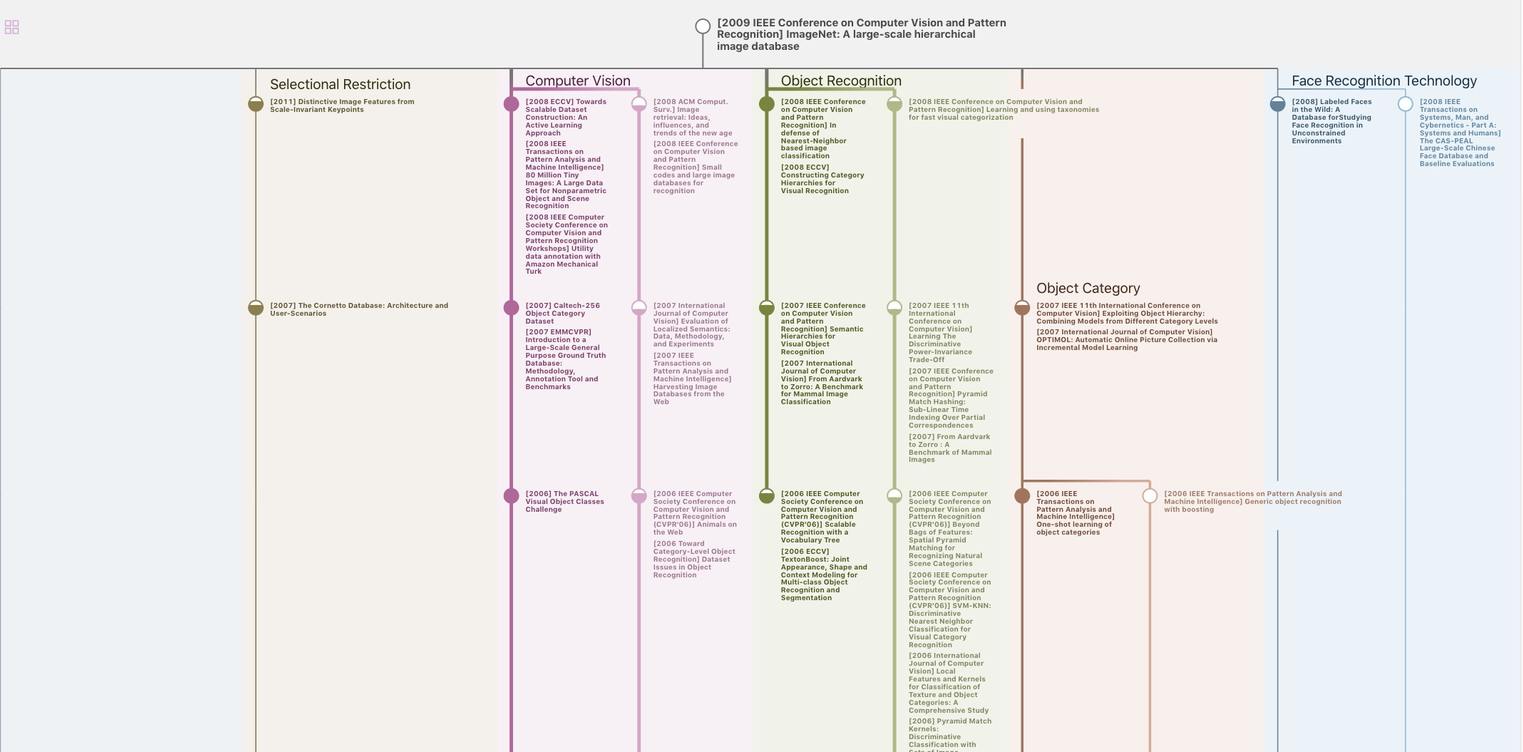
生成溯源树,研究论文发展脉络
Chat Paper
正在生成论文摘要