Fixed confidence community mode estimation
PERFORMANCE EVALUATION(2023)
摘要
Our aim is to estimate the largest community (a.k.a., mode) in a population composed of multiple disjoint communities. This estimation is performed in a fixed confidence setting via sequential sampling of individuals with replacement. We consider two sampling models: (i) an identityless model, wherein only the community of each sampled individual is revealed, and (ii) an identity-based model, wherein the learner is able to discern whether or not each sampled individual has been sampled before, in addition to the community of that individual. The former model corresponds to the classical problem of identifying the mode of a discrete distribution, whereas the latter seeks to capture the utility of identity information in mode estimation. For each of these models, we establish information theoretic lower bounds on the expected number of samples needed to meet the prescribed confidence level, and propose sound algorithms with a sample complexity that is provably asymptotically optimal. Our analysis highlights that identity information can indeed be utilized to improve the efficiency of community mode estimation.
更多查看译文
关键词
Mode estimation,Identity-based sampling,PAC algorithms,Generalized likelihood ratio (GLR) statistics
AI 理解论文
溯源树
样例
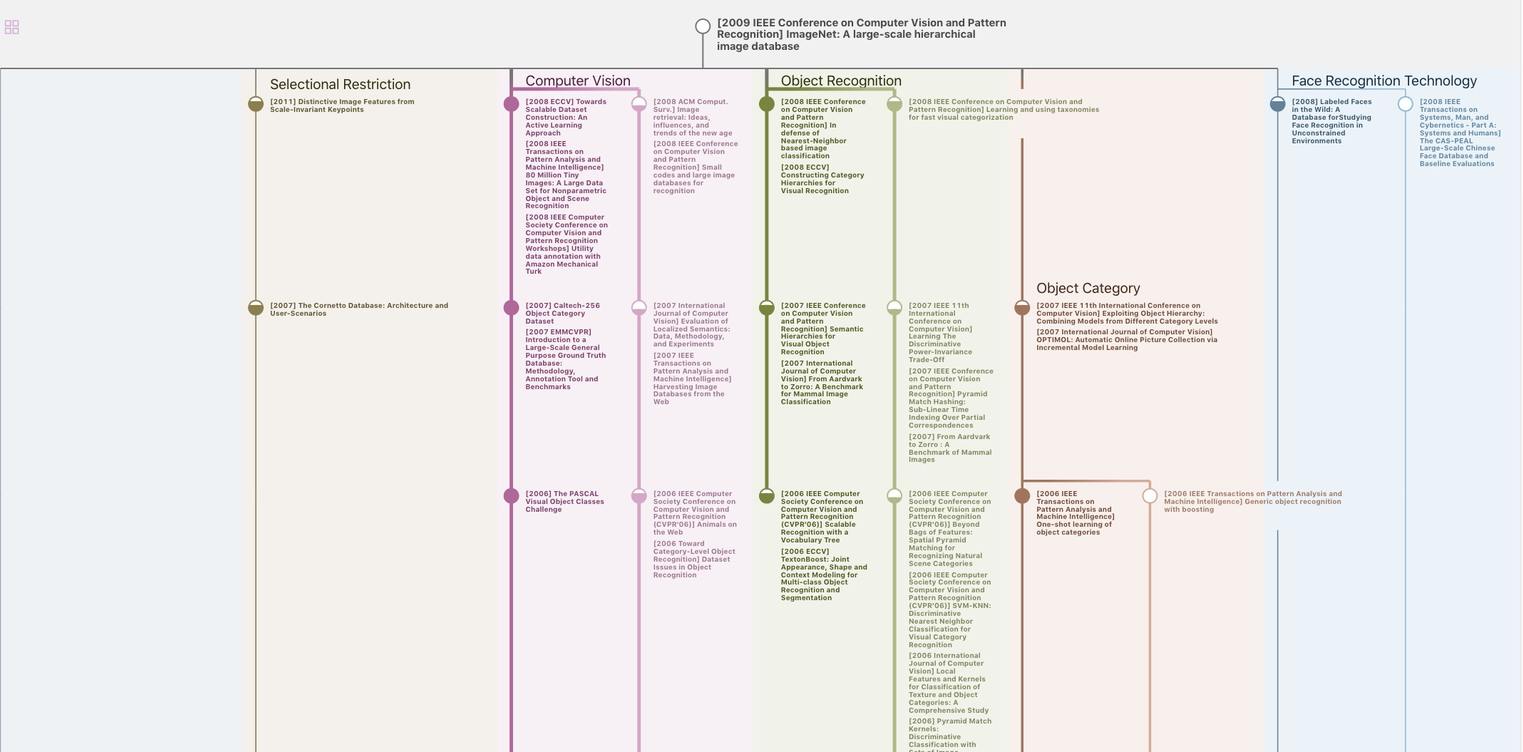
生成溯源树,研究论文发展脉络
Chat Paper
正在生成论文摘要