Confidence Calibration for Systems with Cascaded Predictive Modules
CoRR(2023)
摘要
Existing conformal prediction algorithms estimate prediction intervals at target confidence levels to characterize the performance of a regression model on new test samples. However, considering an autonomous system consisting of multiple modules, prediction intervals constructed for individual modules fall short of accommodating uncertainty propagation over different modules and thus cannot provide reliable predictions on system behavior. We address this limitation and present novel solutions based on conformal prediction to provide prediction intervals calibrated for a predictive system consisting of cascaded modules (e.g., an upstream feature extraction module and a downstream regression module). Our key idea is to leverage module-level validation data to characterize the system-level error distribution without direct access to end-to-end validation data. We provide theoretical justification and empirical experimental results to demonstrate the effectiveness of proposed solutions. In comparison to prediction intervals calibrated for individual modules, our solutions generate improved intervals with more accurate performance guarantees for system predictions, which are demonstrated on both synthetic systems and real-world systems performing overlap prediction for indoor navigation using the Matterport3D dataset.
更多查看译文
关键词
cascaded predictive modules,calibration
AI 理解论文
溯源树
样例
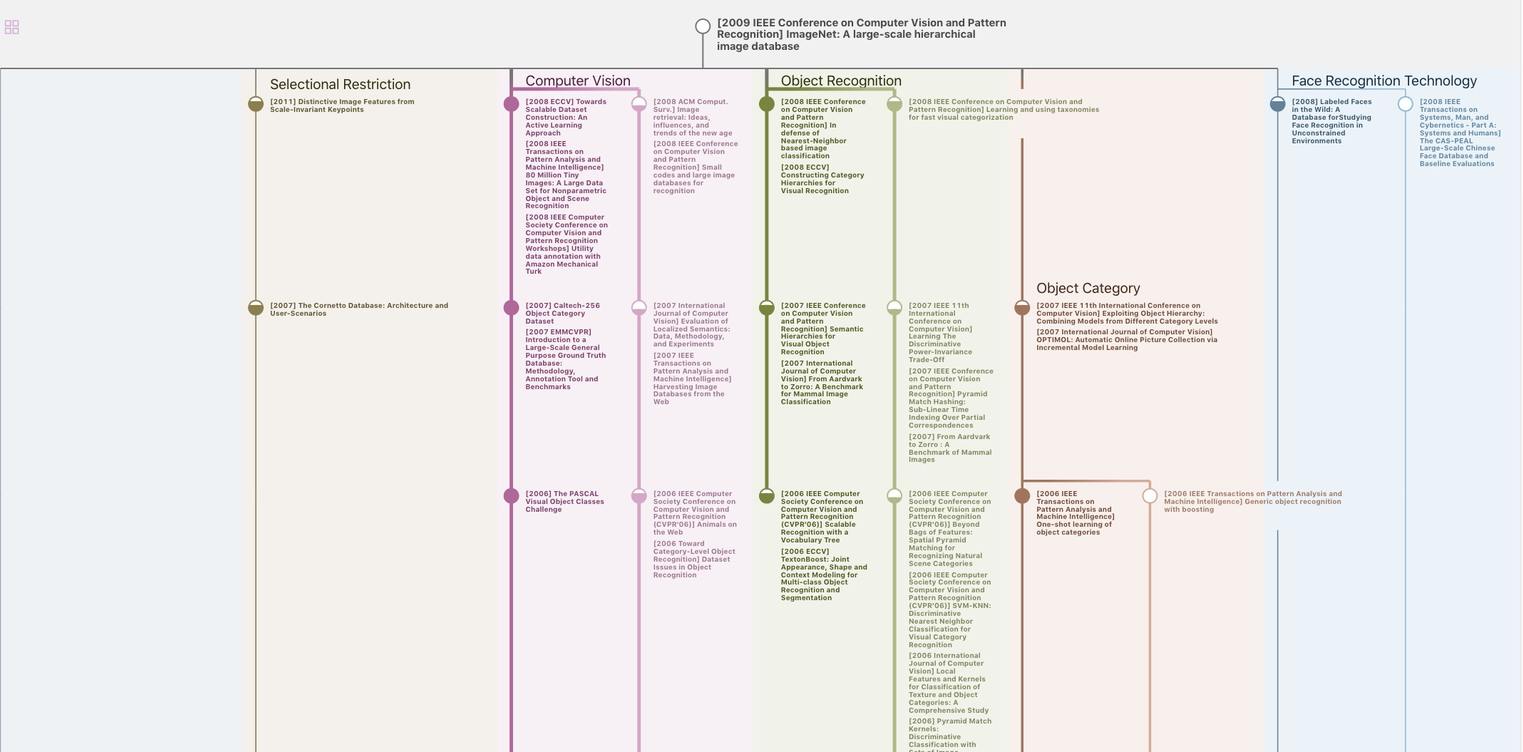
生成溯源树,研究论文发展脉络
Chat Paper
正在生成论文摘要