Knowledge Graph Embedding: An Overview
APSIPA TRANSACTIONS ON SIGNAL AND INFORMATION PROCESSING(2024)
摘要
Many mathematical models have been leveraged to design embeddings for representing Knowledge Graph (KG) entities and relations for link prediction and many downstream tasks. These mathematically-inspired models are not only highly scalable for inference in large KGs, but also have many explainable advantages in modeling different relation patterns that can be validated through both formal proofs and empirical results. In this paper, we make a comprehensive overview of the current state of research in KG completion. In particular, we focus on two main branches of KG embedding (KGE) design: 1) distance-based methods and 2) semantic matching-based methods. We discover the connections between recently proposed models and present an underlying trend that might help researchers invent novel and more effective models. Next, we delve into CompoundE and CompoundE3D, which draw inspiration from 2D and 3D affine operations, respectively. They encompass a broad spectrum of distance-based embedding techniques. We will also discuss an emerging approach for KG completion which leverages pre-trained language models (PLMs) and textual descriptions of entities and relations and offer insights into the integration of KGE embedding methods with PLMs for KG completion.
更多查看译文
关键词
Knowledge Graph Embedding,Knowledge Graph Completion,Link Prediction
AI 理解论文
溯源树
样例
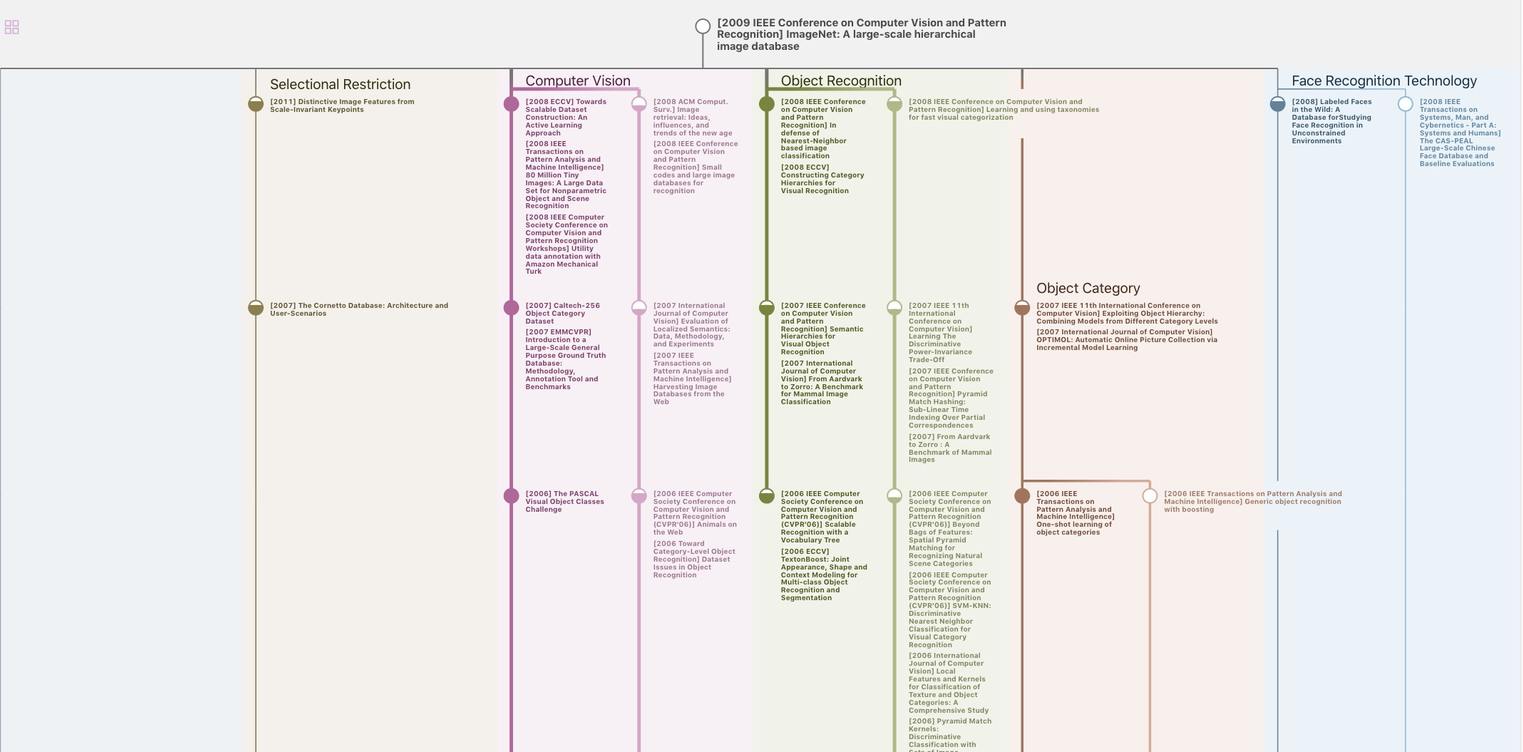
生成溯源树,研究论文发展脉络
Chat Paper
正在生成论文摘要