Ensemble of deep reinforcement learning with optimization model for DDoS attack detection and classification in cloud based software defined networks
MULTIMEDIA TOOLS AND APPLICATIONS(2023)
摘要
Distributed Denial of Service (DDoS) attacks pose a challenging threat to the availability and performance of cloud-based Software-Defined Networks (SDNs). Detection and classification of DDoS attacks in such dynamic and highly virtualized environments is critical to maintaining network stability and ensuring uninterrupted services. Therefore, this study develops a metaheuristic with Multi-Layer Ensemble Deep Reinforcement Learning for DDoS Attack Detection and Mitigation (MMEDRL-ADM) technique in the Cloud SDN Environment. The presented MMEDRL-ADM technique leverages metaheuristics with deep learning model for the recognition of DDoS attacks in the SDN data plane. To accomplish this, the presented MMEDRL-ADM technique initially preprocesses the network data. Next, the MMEDRL-ADM technique designs African buffalo optimization algorithm-based feature selection (ABO-FS) to reduce the computation complexity and increase the detection rate. For DDoS attack detection, the multilayer ensemble deep reinforcement learning (MEDRL) technique is used. To adjust the hyperparameter values of the MEDRL technique, an improved grasshopper optimization algorithm (IGOA) is exploited. The design of MEDRL approach with IGOA based hyperparameter tuning demonstrates the novelty of the work. The experimental validation of the MMEDRL-ADM system is tested under a benchmark dataset. The comparison study highlighted the improved performance of the MMEDRL-ADM technique over other models.
更多查看译文
关键词
ddos attack detection,deep reinforcement learning,cloud
AI 理解论文
溯源树
样例
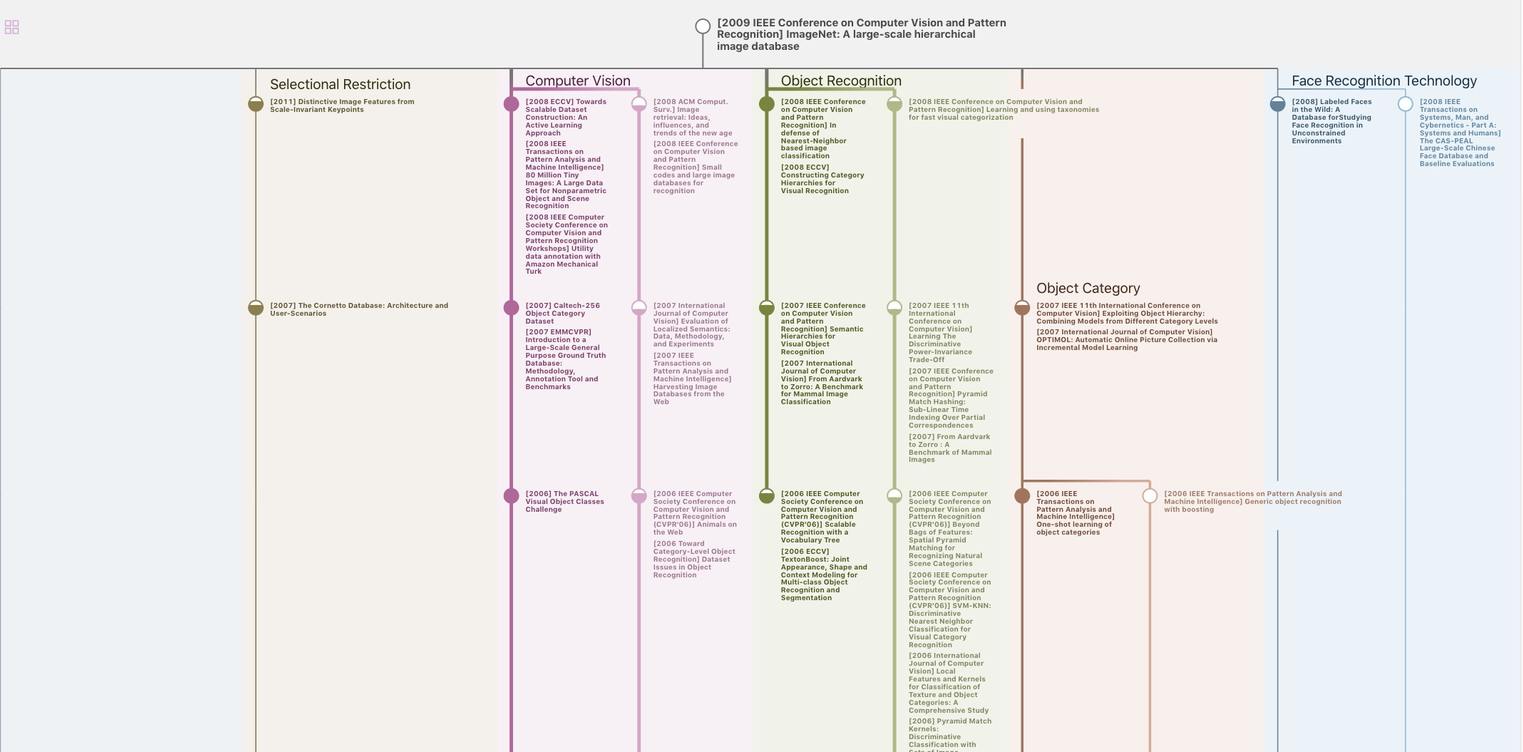
生成溯源树,研究论文发展脉络
Chat Paper
正在生成论文摘要