Magnetic resonance image segmentation of rectal tumors based on improved CycleGAN and U-Net models
Multimedia Tools and Applications(2024)
摘要
Accurate segmentation of rectal tumor lesion regions can provide an essential basis for clinical treatment and prognosis monitoring of tumors. However, there are many problems in the rectal tumor segmentation task at present: the lack of high-quality datasets; the mainstream segmentation network cannot complete the high-precision segmentation task of rectal tumors. In this paper, we investigate image enhancement and segmentation algorithms for convolutional neural networks and construct a rectal tumor MRI dataset by improving the CycleGAN network and loss function to achieve domain migration and reconstruction of rectal tumor CT and MRI images, given the small amount of rectal tumor image data and the existence of different modalities and regimes in CT and MRI. For the rectal tumor segmentation problem, a novel segmentation network DCMSG-UNet was designed based on the U-Net network. this network uses dilated convolution and multi-headed self-attention mechanisms to improve the base feature extraction module of the segmentation network, adds a decoder path, and uses the GAM hybrid attention mechanism to amplify the dimensional interaction features of the additional decoder path. Comparison experiments with six network models, DeepLabV3+, U-Net, UNet++, UNet3+, TransUNet, and Swin-Unet, show that the segmented region obtained from the DCMSG-UNet model proposed in this paper is closer to the real tumor region, with a DICE metric of 0.8416 and a Hausdorff distance of 11.3229, which can effectively segment the tumor. The experimental results show that our proposed method performs significantly better than the above methods, with a DICE metrics improvement of about 6%. To visualize the segmentation results, this paper designed a rectal tumor MRI image segmentation system based on PyQt5 to realize human-computer interaction and assist doctors in clinical diagnosis.
更多查看译文
关键词
Medical image segmentation,Data enhancement,U-Net,Attention mechanism,CycleGAN
AI 理解论文
溯源树
样例
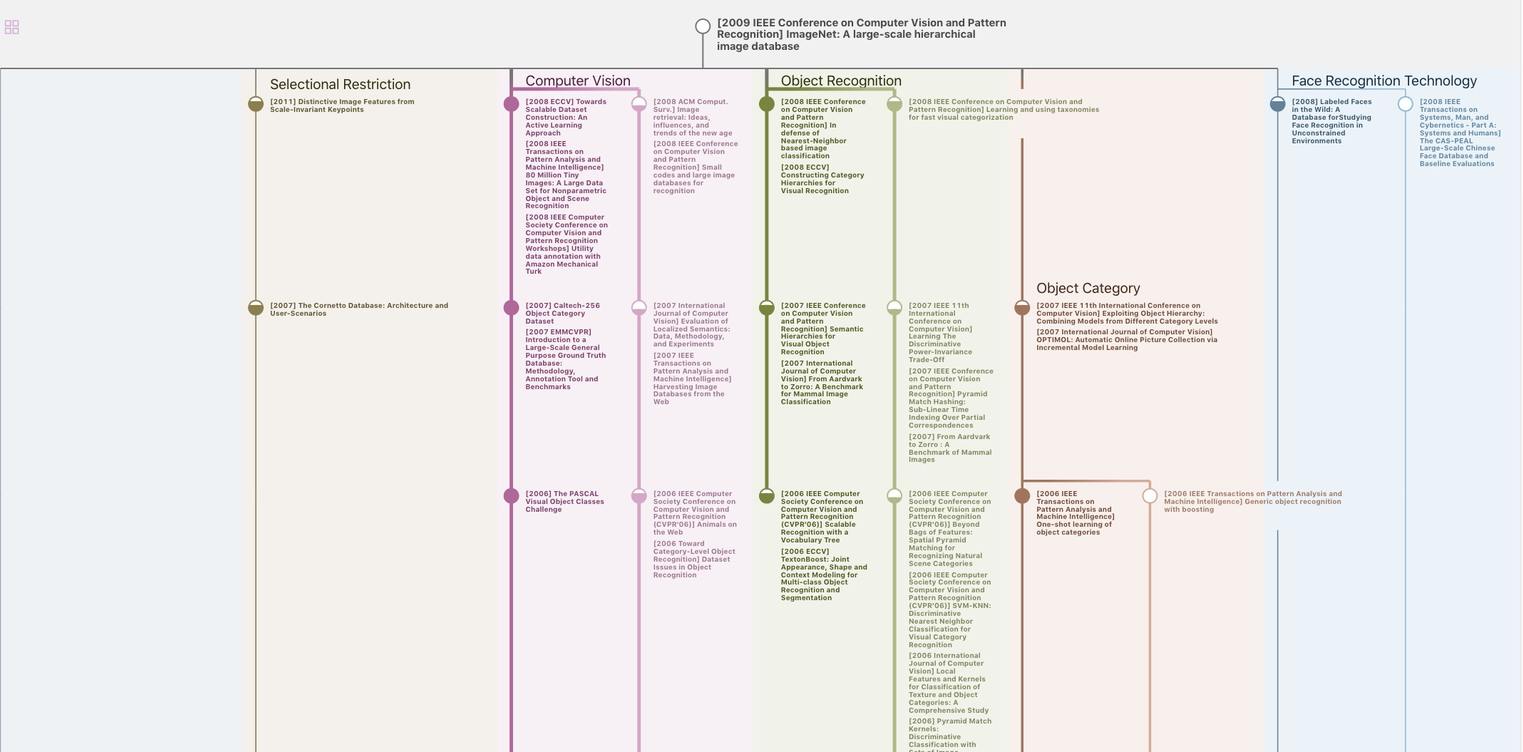
生成溯源树,研究论文发展脉络
Chat Paper
正在生成论文摘要