Time-domain Subtractive Readout Scheme for Scalable Capacitive Analog In-Memory Computing
2023 IEEE 36th International System-on-Chip Conference (SOCC)(2023)
摘要
In-memory computing is a promising architecture to meet the exploding demand for data-intensive workloads, including deep neural networks. In particular, analog in-memory computings (AIMCs) is a promising way to build matrix multiplication accelerators that take full advantage of data parallelism and reusability. However, most AIMCs use voltage readout circuits that have no benefit from CMOS scaling, which is an obstacle to improving computational density. We propose a method that combines capacitive AIMC and readout with near-memory time-subtraction, which is theoretically scalable concerning miniaturization and row/column parallelism and is adjustable with output resolution. We have evaluated the signed multi-bit dot product operation in post-layout simulation using circuits designed with a 180-nm process. Even with × 16 increase in row-parallelism (9 to 144), the time resolution required for readout was successfully reduced to a variation of 0.39%.
更多查看译文
关键词
Analog In-memory Computing,Capacitive In-Memory Computing,Time-domain Computing
AI 理解论文
溯源树
样例
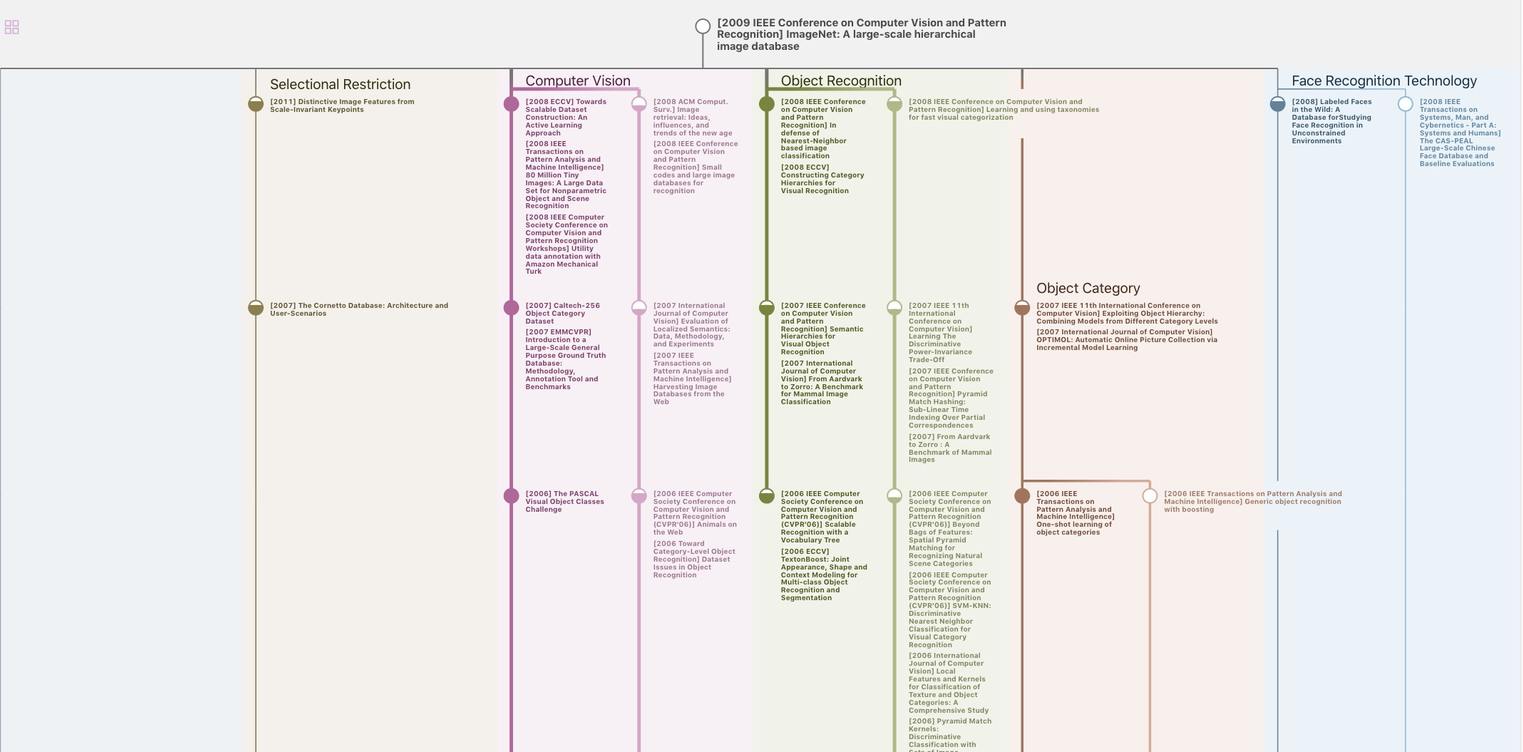
生成溯源树,研究论文发展脉络
Chat Paper
正在生成论文摘要