Realistic fault detection of li-ion battery via dynamical deep learning
Nature Communications(2023)
摘要
Accurate evaluation of Li-ion battery (LiB) safety conditions can reduce unexpected cell failures, facilitate battery deployment, and promote low-carbon economies. Despite the recent progress in artificial intelligence, anomaly detection methods are not customized for or validated in realistic battery settings due to the complex failure mechanisms and the lack of real-world testing frameworks with large-scale datasets. Here, we develop a realistic deep-learning framework for electric vehicle (EV) LiB anomaly detection. It features a dynamical autoencoder tailored for dynamical systems and configured by social and financial factors. We test our detection algorithm on released datasets comprising over 690,000 LiB charging snippets from 347 EVs. Our model overcomes the limitations of state-of-the-art fault detection models, including deep learning ones. Moreover, it reduces the expected direct EV battery fault and inspection costs. Our work highlights the potential of deep learning in improving LiB safety and the significance of social and financial information in designing deep learning models.
更多查看译文
关键词
realistic fault detection,dynamical deep learning,li-ion
AI 理解论文
溯源树
样例
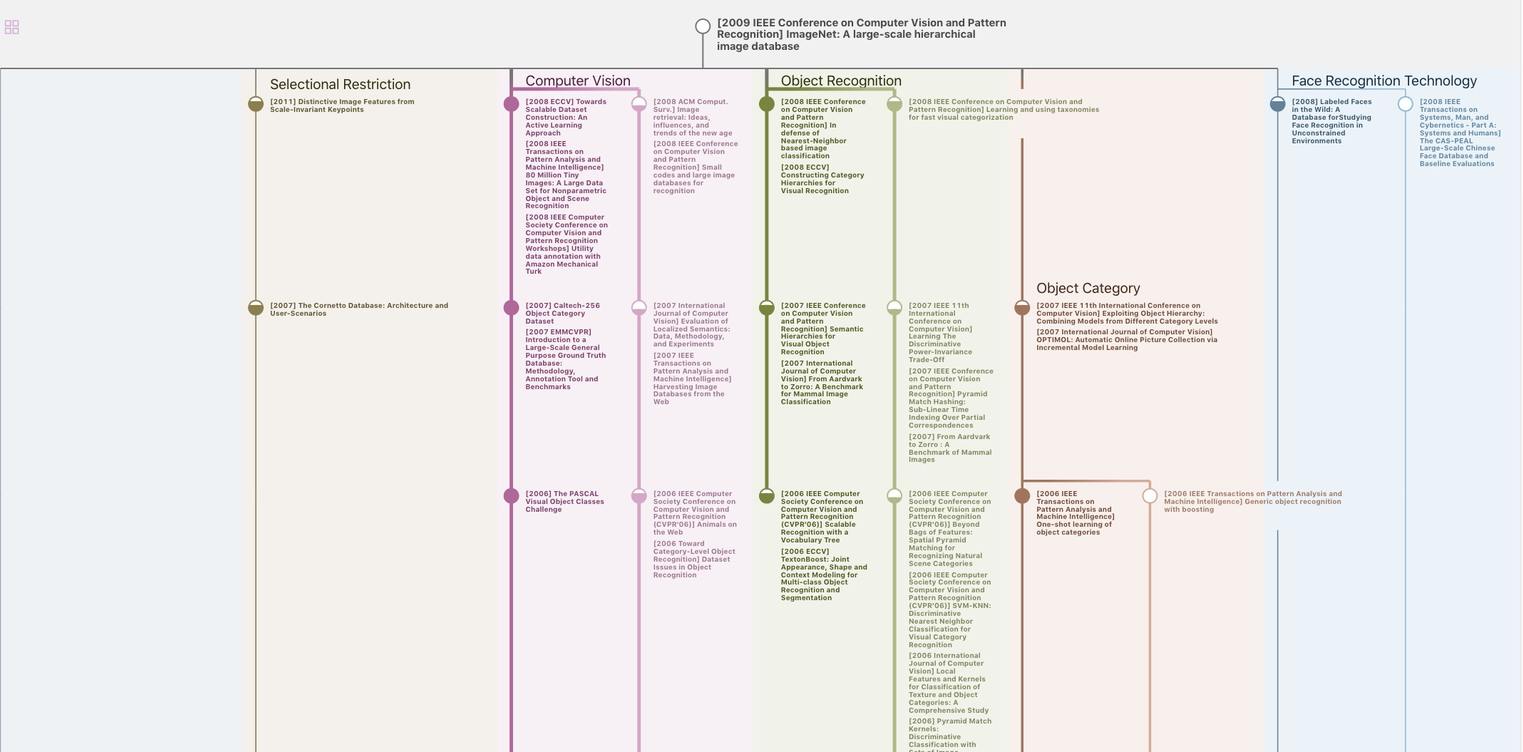
生成溯源树,研究论文发展脉络
Chat Paper
正在生成论文摘要