A radiomics-based logistic regression model for the assessment of emphysema severity
Tuberkuloz ve toraks(2023)
摘要
A radiomics-based logistic regression model for the assessment of emphysema severityIntroduction: The aim of this study is to develop a model that differentiates between the radiological patterns of severe and mild emphysema using radiomics parameters, as well as to examine the parameters included in the model.Materials and Methods: Over the last 12 months, a total of 354 patients were screened based on the presence of terms such as "Fleischner", "CLE", and "centriacinar" in their thoracic CT reports, culminating in a study population of 82 patients. The study population was divided into Group 1 (Fleischner mild and moderate; n= 45) and Group 2 (Fleischner confluent and advanced destructive; n= 37). Volumetric segmentation was performed, focusing on the upper lobe segments of both lungs. From these segmented volumes, radiomics parameters including shape, size, first-order, and second-order features were calculated. The best model parameters were selected based on the Bayesian Information Criterion and further optimized through grid search. The final model was tested using 1000 iterations of bootstrap resampling.Results: In the training set, performance metrics were calculated with a sensitivity of 0.862, specificity of 0.870, accuracy of 0.863, and AUC of 0.910. Correspondingly, in the test set, these values were sensitivity= 0.848; specificity= 0.865; accuracy= 0.857; and AUC= 0.907.Conclusion: The logistic regression model, composed of radiomics parameters and trained on a limited number of cases, effectively differentiated between mild and severe radiological patterns of emphysema using computed tomography images.
更多查看译文
关键词
Emphysema, machine learning, logistic regression
AI 理解论文
溯源树
样例
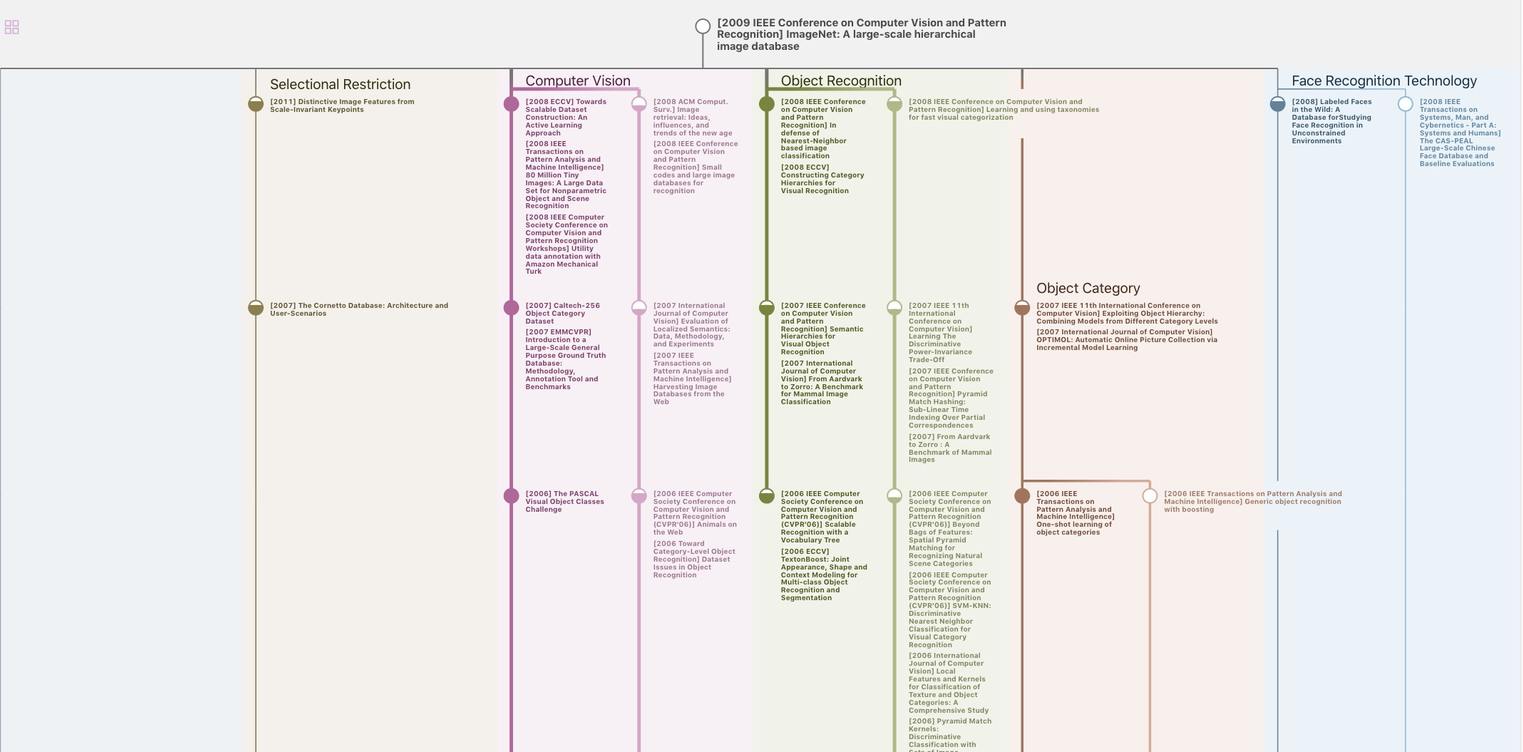
生成溯源树,研究论文发展脉络
Chat Paper
正在生成论文摘要