Machine Learning-Enabled Tactile Sensor Design for Dynamic Touch Decoding.
Advanced science (Weinheim, Baden-Wurttemberg, Germany)(2023)
摘要
Skin-like flexible sensors play vital roles in healthcare and human-machine interactions. However, general goals focus on pursuing intrinsic static and dynamic performance of skin-like sensors themselves accompanied with diverse trial-and-error attempts. Such a forward strategy almost isolates the design of sensors from resulting applications. Here, a machine learning (ML)-guided design of flexible tactile sensor system is reported, enabling a high classification accuracy (≈99.58%) of tactile perception in six dynamic touch modalities. Different from the intuition-driven sensor design, such ML-guided performance optimization is realized by introducing a support vector machine-based ML algorithm along with specific statistical criteria for fabrication parameters selection to excavate features deeply concealed in raw sensing data. This inverse design merges the statistical learning criteria into the design phase of sensing hardware, bridging the gap between the device structures and algorithms. Using the optimized tactile sensor, the high-quality recognizable signals in handwriting applications are obtained. Besides, with the additional data processing, a robot hand assembled with the sensor is able to complete real-time touch-decoding of an 11-digit braille phone number with high accuracy.
更多查看译文
关键词
human–machine interactions,laser‐induced graphene,machine learning,tactile sensor,touch decoding
AI 理解论文
溯源树
样例
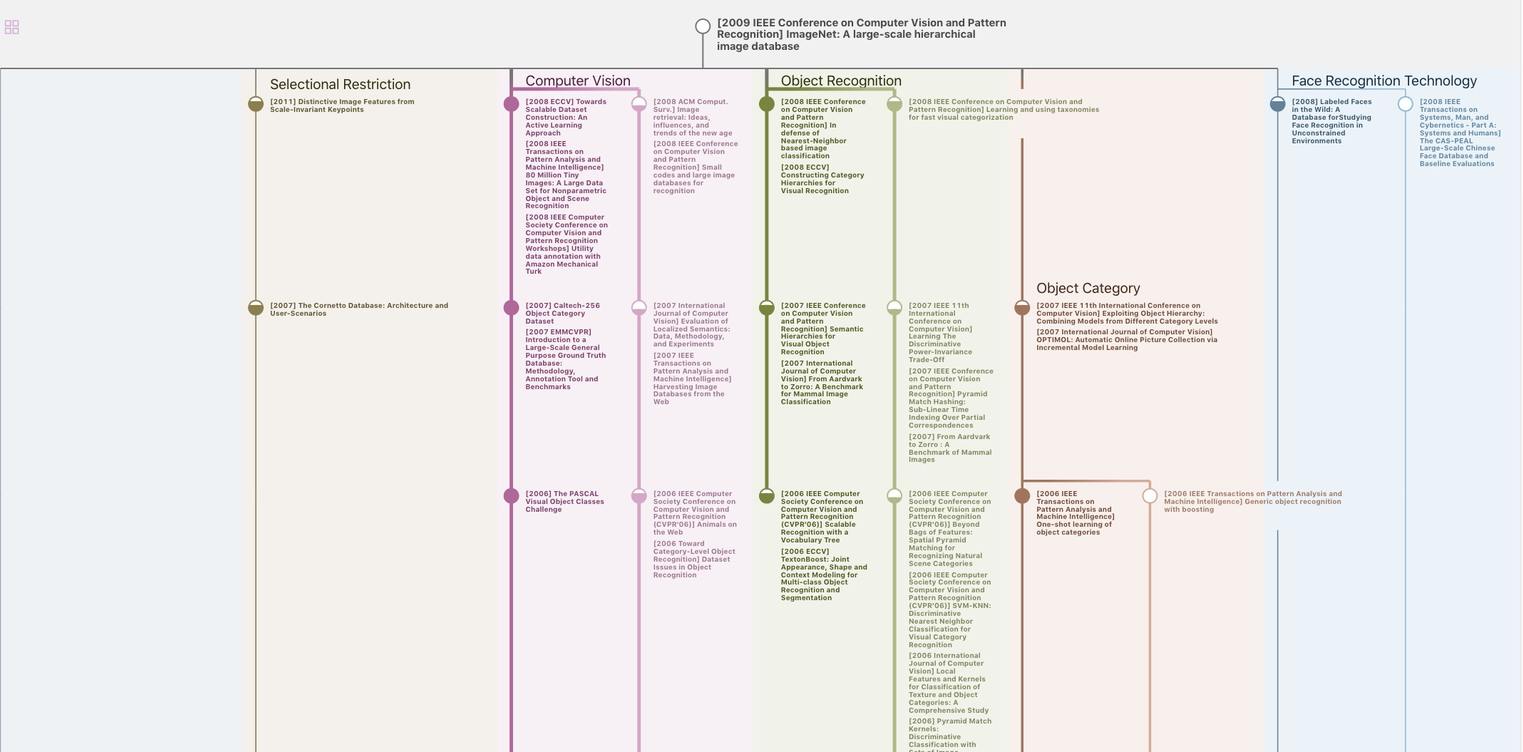
生成溯源树,研究论文发展脉络
Chat Paper
正在生成论文摘要